Comparative Analysis of Different Machine Learning Methods for Hate Speech Recognition in Twitter Text Data
2022 Third International Conference on Intelligent Computing Instrumentation and Control Technologies (ICICICT)(2022)
摘要
The purpose of this work is to solve the challenges faced by us in the field of hate speech recognition on various social media platforms, that is to get a better machine learning model that can detect hate speech with greater accuracy. As the reach of the internet and mobile phones has extended abruptly in the past few years, everyone has the power to share their opinions, but some use it as an opportunity to spread hate among one another. In this paper, we used the Davidson [10] dataset which is the most popular Twitter dataset for hate speech detection, and further we implemented various machine learning-based algorithms and compared them on the basis of various parameters such as accuracy, precision score, recall and F1 scores. After the study, we found out that XGBoost when used with TF-IDF transformer embedding gave us an accuracy of 94.43%, which is the maximum among these three models for the given benchmark dataset.
更多查看译文
关键词
hate speech recognition,offensive Tweets,social media,twitter dataset,LSTM,XGBoost,multinomial Naïve Bayes
AI 理解论文
溯源树
样例
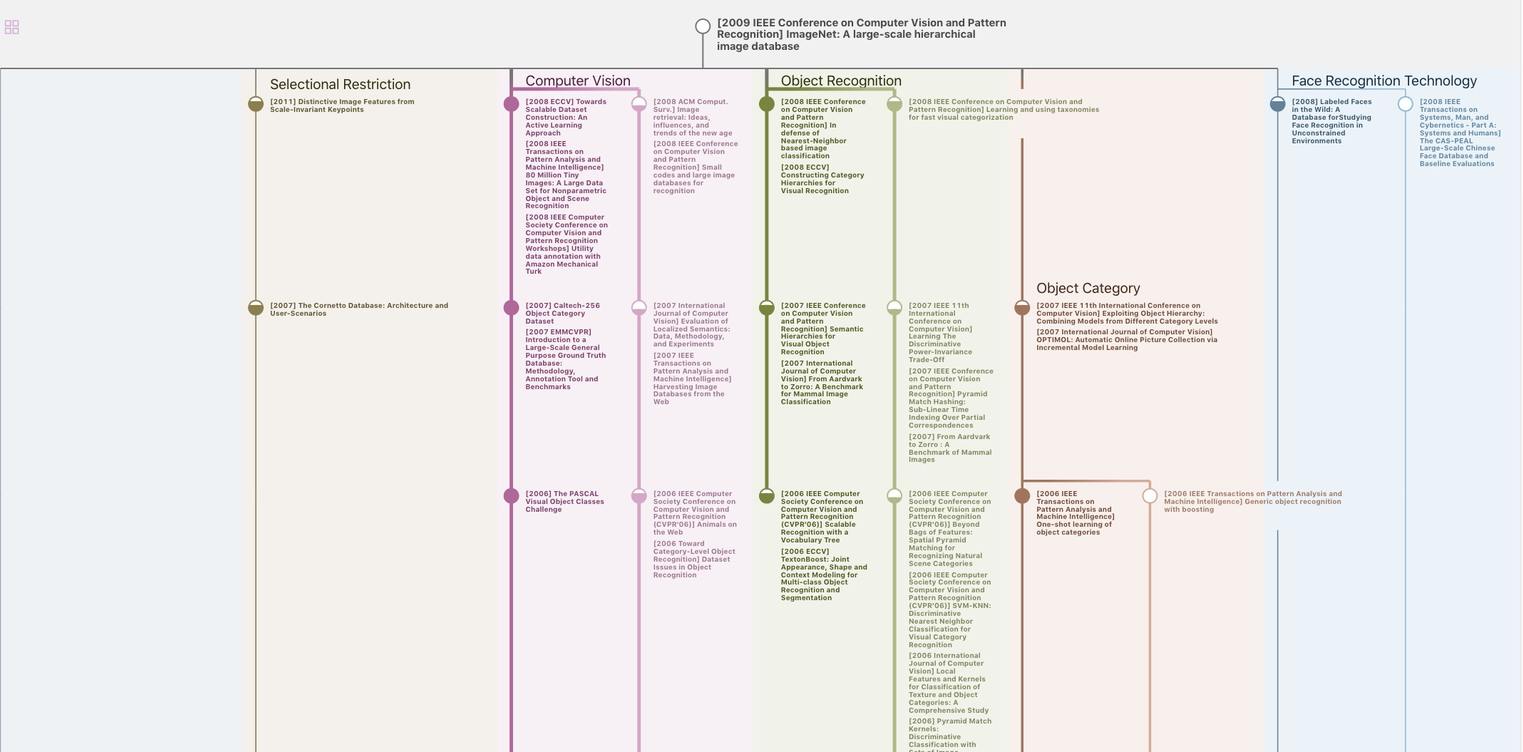
生成溯源树,研究论文发展脉络
Chat Paper
正在生成论文摘要