Data augmentation in semi-supervised adversarial domain adaptation for EEG-based sleep staging
2022 IEEE-EMBS International Conference on Biomedical and Health Informatics (BHI)(2022)
摘要
The upcoming era of wearable health monitoring devices has created a need for automated signal processing algorithms that can be trained with a minimal amount of labeled data. In our previous work, we showed that transfer learning techniques like semi-supervised adversarial domain adaptation can help to achieve this. We applied our method to remote sleep monitoring, by performing sleep staging on single-channel wearable EEG signals. In this work, we propose data augmentation to help in tackling this challenge. By using an artificially increased amount of labeled data, our semi-supervised adversarial domain adaptation method improves its performance on the wearable EEG data. The accuracy is increased consistently by 0.6% to 1.4% relative to the results without augmentation. As both adversarial domain adaptation and data augmentation are strategies to deal with the scarceness of data, we conclude that these methods are can effectively be combined to surpass their individual performance.
更多查看译文
关键词
domain adaptation,transfer learning,electroen-cephalography,deep learning,sleep staging,data augmentation
AI 理解论文
溯源树
样例
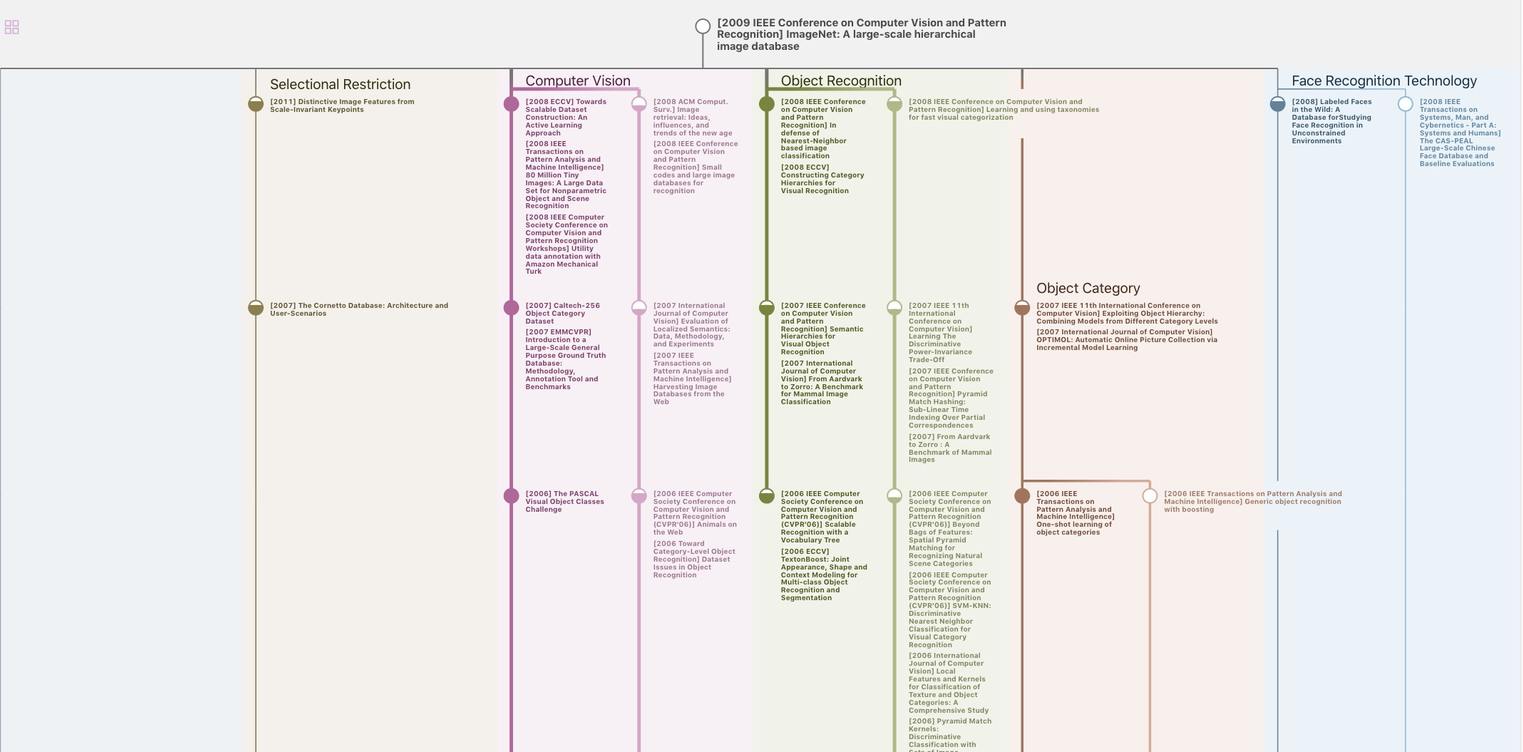
生成溯源树,研究论文发展脉络
Chat Paper
正在生成论文摘要