A Comparative Study on Deep Feature Extraction Approaches for Visual Tracking of Industrial Robots
2022 IEEE Industrial Electronics and Applications Conference (IEACon)(2022)
摘要
Owing to several breakthroughs in deep-learning research, visual object trackers based on deep learning algorithms have been the de facto method in the tracking community. However, the performance of this category of trackers is largely influenced by the richness and quality of the target feature representation. Thus, to extract features with high discriminative power, special attention must be paid to the feature extraction component of the tracker. Despite the significance of feature extraction paradigm in deep learning-based trackers, limited studies have been dedicated to comparatively examining the feature learning and representation capabilities of various existing deep feature extraction approaches in visual tracking. In this paper, we present a comparative study on two different deep feature extraction methods in visual tracking of industrial robots in video. This work examines and visualizes the feature representation capability of two commonly employed feature extraction methods in deep learning-based tracking; namely, Convolutional Neural Network (CNN)-based and Transformer-based feature extraction methods. To demonstrate the effectiveness of the deep learning-based tracking paradigm, this study adopts a Siamese-based tracker that is trained end-to-end offline with ILSVRC15 datasets as well as a Transformer-based tracking architecture that is also pre-trained on the ImageNet dataset. This study also contributes a dataset consisting of images of industrial robots, which was employed for evaluating the two adopted trackers. From this study, we could conclude that the feature learning and representation of the transformer-based feature extraction pipeline largely improved the overall tracking performance of the tracker.
更多查看译文
关键词
visual object tracking,feature extraction,Industrial robots,deep learning,Transformer network,CNN
AI 理解论文
溯源树
样例
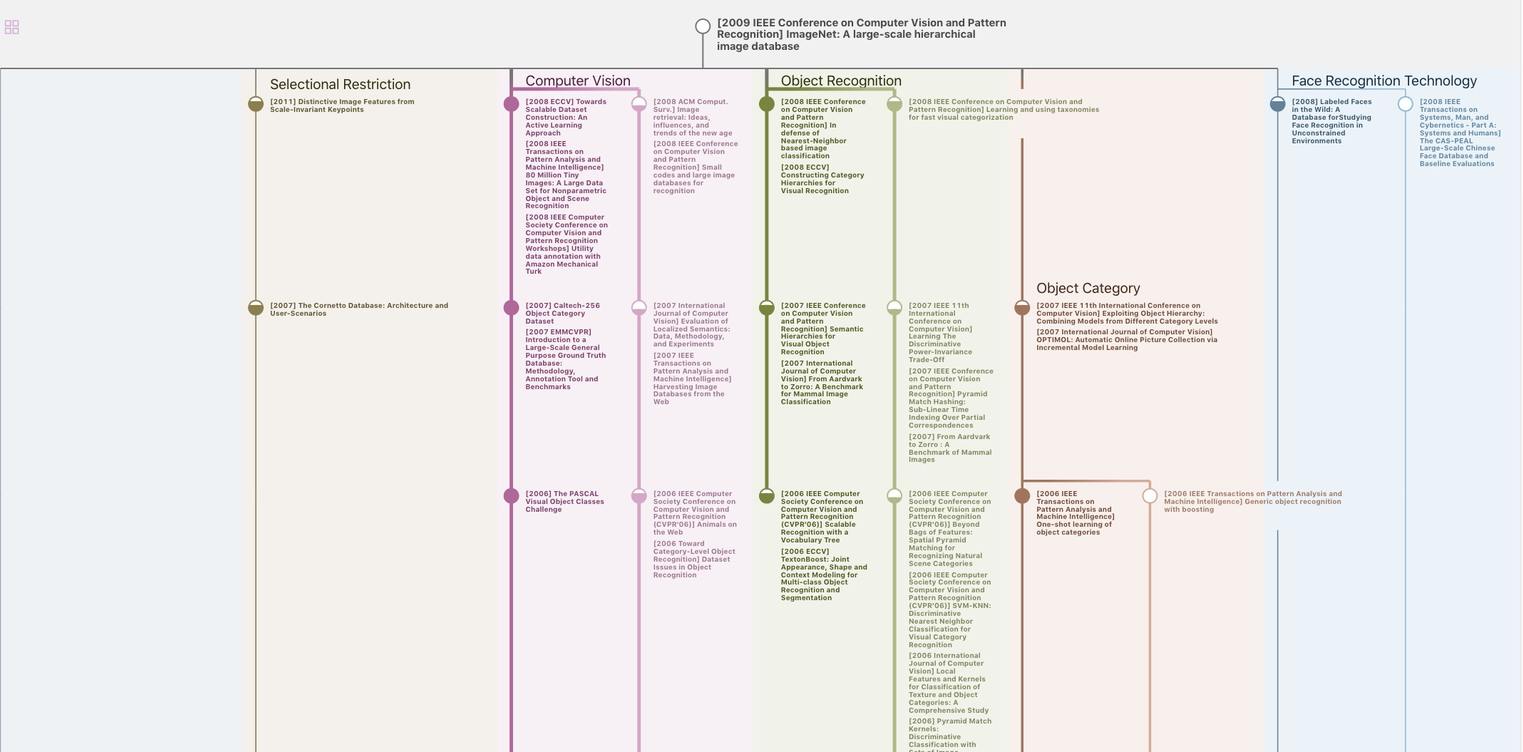
生成溯源树,研究论文发展脉络
Chat Paper
正在生成论文摘要