A Tensor Non-Convex Low Rank and Sparse Constrained Band Selection Scheme for Clustering of Hyperspectral Paper Data.
2022 12TH WORKSHOP ON HYPERSPECTRAL IMAGING AND SIGNAL PROCESSING EVOLUTION IN REMOTE SENSING (WHISPERS)(2022)
摘要
Conventional band selection methods, which vectorize the spectral bands of Hyperspectral Imaging (HSI) data, may fail to consider its intrinsic two dimensional structure. Hence, an effective tensor based band selection scheme, using submodule clustering is proposed, where this framework is capable of preserving the spatial structure of individual spectral bands. In the proposed optimization problem, a representation tensor is derived from the self-expressive representation of spectral bands in the HSI paper data. A similarity matrix is constructed from the representation tensor and then, appropriate number of non-redundant bands are determined by Silhouette clustering evaluation criterion. To capture better low rankness and self-expressiveness property, $l_{\frac{1}{2}}$ -induced Tensor Nuclear Norm (TNN) and $l_{\frac{1}{2}}$ norm regularization are incorporated in the formulated optimization model. Further, the reduced band subset is used in the clustering of hyperspectral paper data. Experimental results prove the efficacy of newly formed band subset on the clustering performance.
更多查看译文
关键词
Band selection,hyperspectral imaging,submodule clustering,sparsity,low rankness
AI 理解论文
溯源树
样例
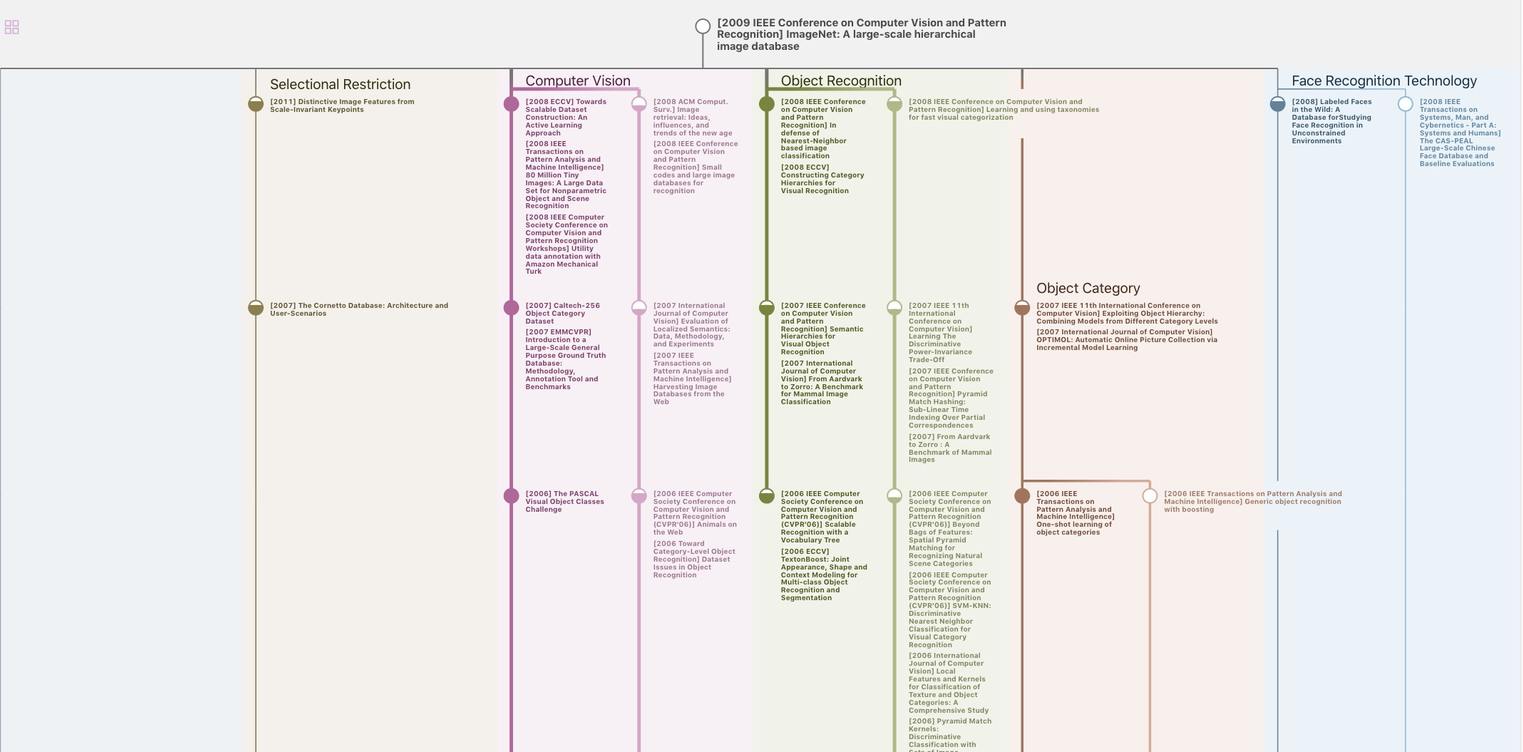
生成溯源树,研究论文发展脉络
Chat Paper
正在生成论文摘要