Truncated representation graph with adaptive weighted and manifold regularization for agglomerative clustering
2022 5th International Conference on Data Science and Information Technology (DSIT)(2022)
摘要
Agglomerative clustering has emerged as a promising technique with its ability to generate the informative cluster hierarchies. The graph learning of data is very common in many agglomerative clustering algorithms. In this problem, the construction of the affinity graph with high quality is a vital element to improve the performance of clustering. To this end, we propose a new method named truncated representation graph with adaptive weighted and manifold regularization. On the one hand, an adaptive weighting matrix is embedded to the reconstruction error term to reinforce the effect of important features during graph learning. It encourages our method to obtain a robust representation graph of the data. On the other hand, the manifold regularization is introduced to capture the geometric structure of the data so that the representation coefficients of data are smooth with respect to the intrinsic data manifold. Moreover, we truncate the trivial representation coefficients to achieve a block-diagonality and more effective graph for agglomerative clustering. The experimental results on various benchmark datasets verify the superiority of our proposed method with respect to the state-of-the-art approaches.
更多查看译文
关键词
Clustering,agglomerative clustering,affinity graph,manifold learning
AI 理解论文
溯源树
样例
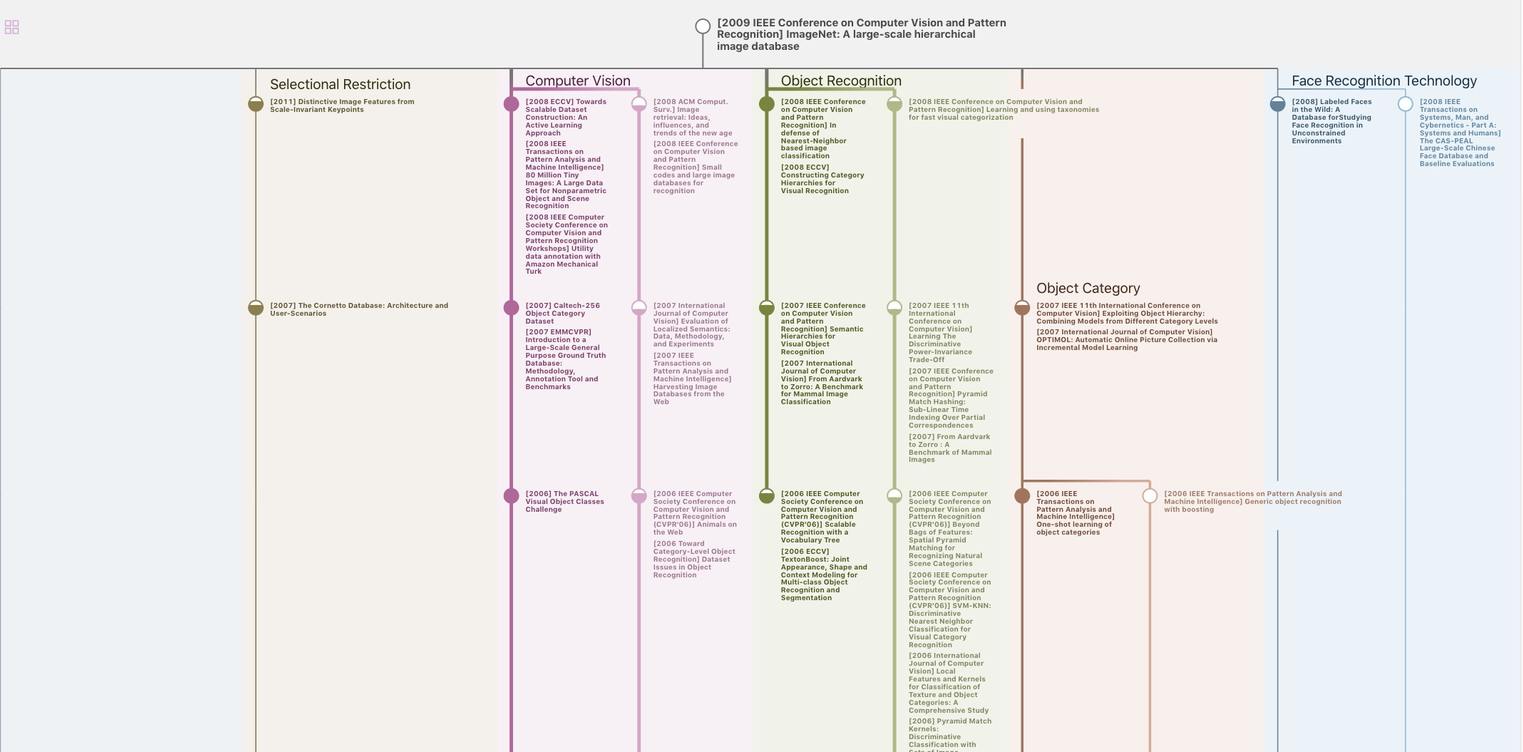
生成溯源树,研究论文发展脉络
Chat Paper
正在生成论文摘要