Vision Transformers Show Improved Robustness in High-Content Image Analysis
2022 9th Swiss Conference on Data Science (SDS)(2022)
摘要
In drug development, image-based bioassays are commonplace, typically run in high throughput on automated microscopes. The resulting cell imaging data comes from multiple instruments and has been acquired at different time points, leading to technical and biological variation in the data, potentially hampering the quantitative analysis across an assay campaign. In this work, we analyze the robustness of a novel concept called Vision Transformers with respect to technical and biological variations. We compare their performance to recent analysis concepts by benchmarking the Cells Out of Sample dataset (COOS) from a high-content imaging screen. The experiments suggest that Vision Transformers are capable of learning more robust representations, thereby even outperforming specially designed deep learning architectures by a large margin.
更多查看译文
关键词
Deep learning,Image classification,Vision Transformer,High Content Image Analysis,Robustness
AI 理解论文
溯源树
样例
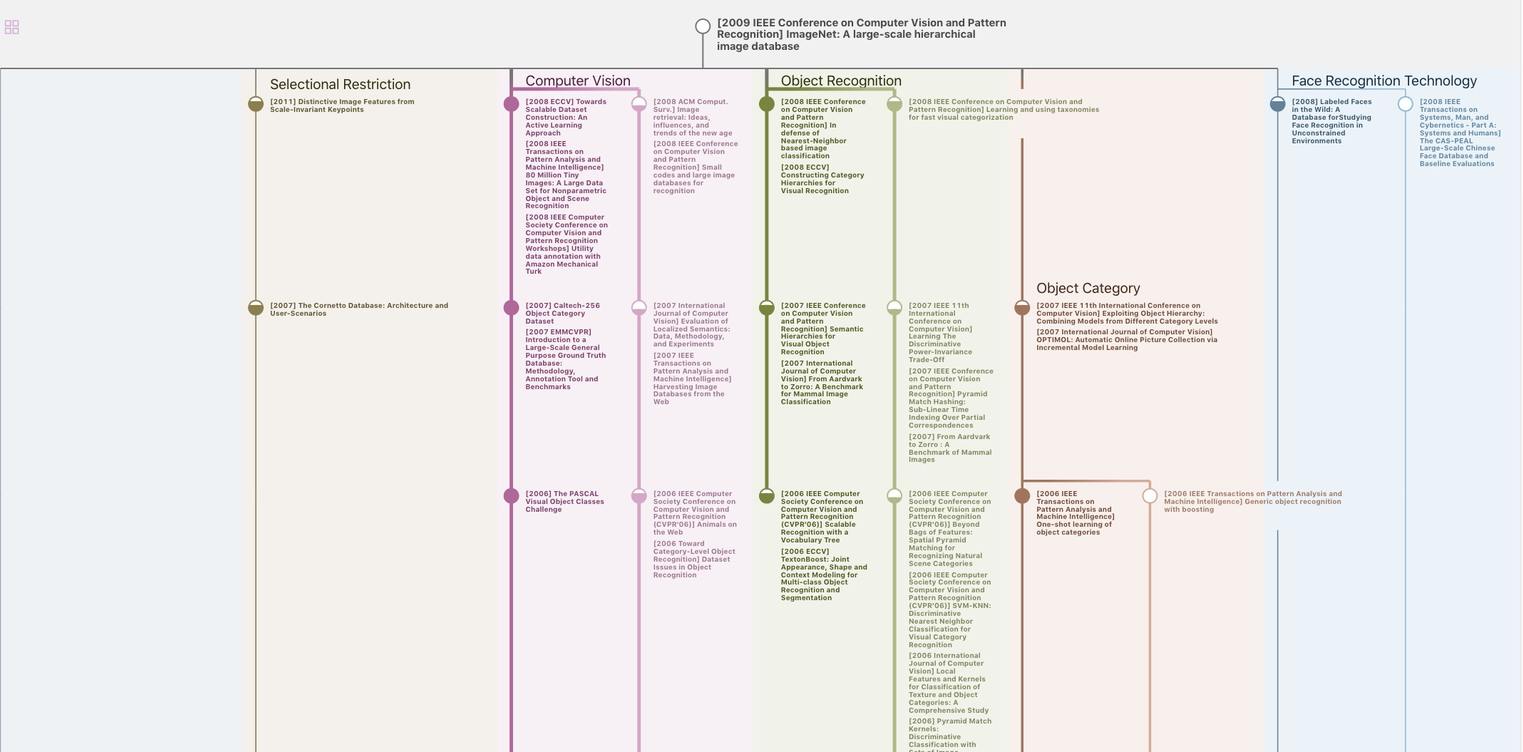
生成溯源树,研究论文发展脉络
Chat Paper
正在生成论文摘要