Machine Learning Based System for Sleep Apnea Classification using EEG Signal
2022 Second International Conference on Computer Science, Engineering and Applications (ICCSEA)(2022)
摘要
Sleep Apnea is a condition in which people and animals cease breathing for a short period of time while sleeping. EEG signals are important in detecting sleep apnea because they detect and record brain activity. This study proposes a method in which the filtered EEG signal is moved for sub-band decomposition after pre-processing and disbanded into five frequency bands: Sigma, Alpha, Beta, Delta, and Theta. The features determined for each frequency band acquired from the filtered EEG signals are energy, kurtosis, MAD, and skewness, which are then imported for the classification process using Decision Tree and Random Forest ML classifiers. Physionet’s publicly available dataset is used to assess each classifier’s accuracy, sensitivity, precision, and specificity. Using the bagging technique, Random Forest method achieves the highest classification accuracy of 99.68 percent, sensitivity of 100 percent, and specificity of 99.37 percent.
更多查看译文
关键词
Decision Tree,Random Forest,Sleep Apnea,EEG,Sub-band filtering,IIR Butterworth bandpass filter.
AI 理解论文
溯源树
样例
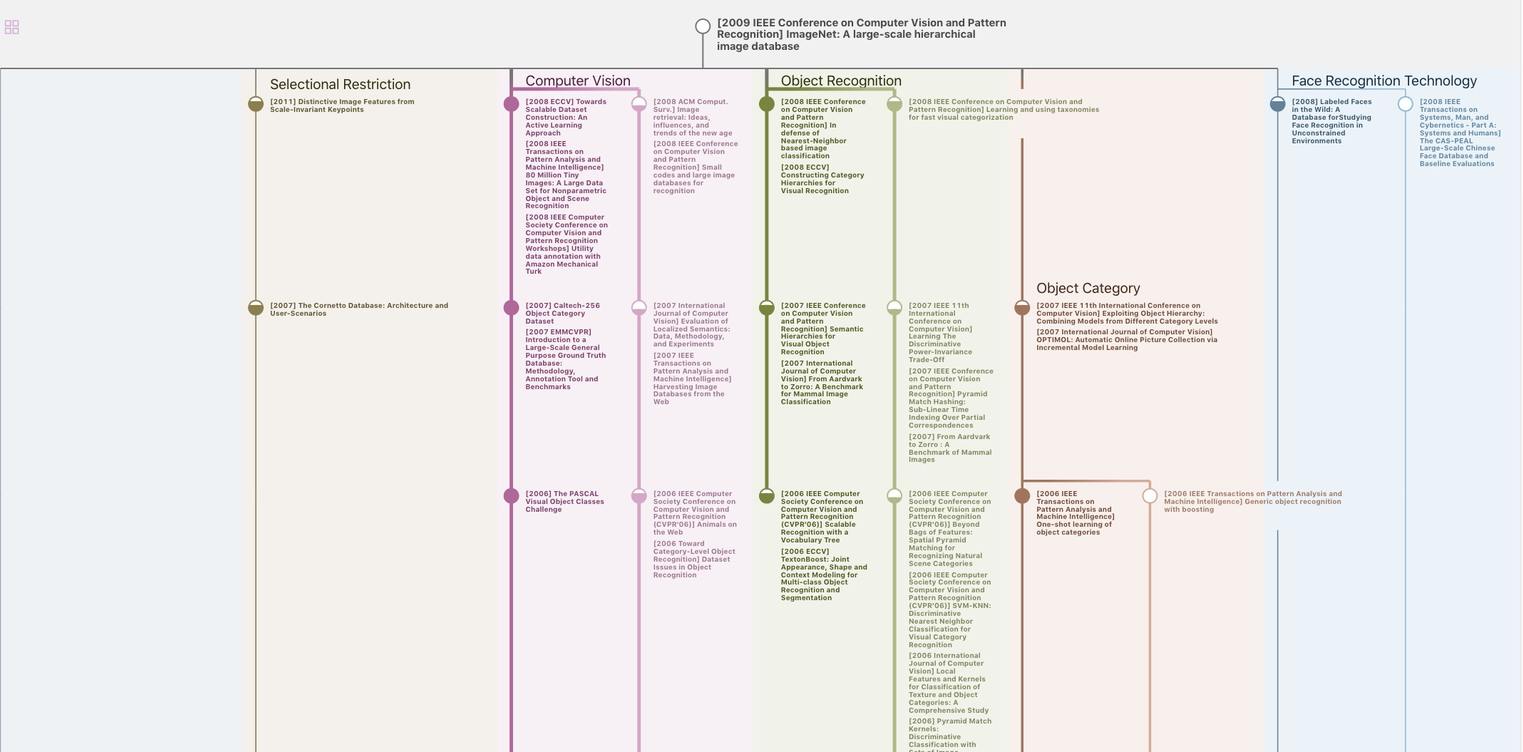
生成溯源树,研究论文发展脉络
Chat Paper
正在生成论文摘要