Multiplanar Instance Segmentation Method for the Detection of Pulmonary Embolism
2022 XVLIII Latin American Computer Conference (CLEI)(2022)
摘要
Pulmonary embolism (PE) is a clinical condition with high mortality and morbidity rates without prompt detection. Computer Aided Detection (CAD) systems support the radiologist in detecting and diagnosing PE using Computed Tomography Pulmonary Angiography (CTPA) images. During the last few years, there have been several contributions to this field by applying Deep Learning models, methods, and algorithms. Still, some challenges need to be addressed to obtain better results. This work is based on the Probability-based Mask R-CNN (P-Mask RCNN) model as our starting point due to the promising results when adapting the Mask R-CNN instance segmentation algorithm for PE detection. With the P-Mask R-CNN model as the backbone, we proposed and implemented a methodology that considers the axial slices of a CTPA image and the sagittal and coronal slices by providing additional information to the whole process. As a result of this exercise, we developed a multiplanar instance segmentation method merging the outputs of three segmentation models trained for each of the three CTPA image planes. The results of feeding a CTPA image into each model were combined into a single volume using three different operations: union, intersection, and majority. An additional contribution surpassing the detection of each model was perceived when compared with the radiologists’ annotations. Testing with the CTPA images of three patients and merging with the union operation resulted in a mean positive percentage detection of 48%, compared to 43% of the axial model, 6.3% of the sagittal model, and 4.8% of the coronal model. Intersection and majority operations also managed to obtain contributions from the three models, which could allow the radiologists to focus on specific parts of the volume where there are the most coincidences. The result of this approach is promising and can help achieve a better performance in detecting PE by focusing on improving specific steps of the methodology in future works.
更多查看译文
关键词
Pulmonary Embolism,Computer Aided Detection,Computed Tomography Pulmonary Angiography,Deep Learning,Instance Segmentation,Mask R-CNN
AI 理解论文
溯源树
样例
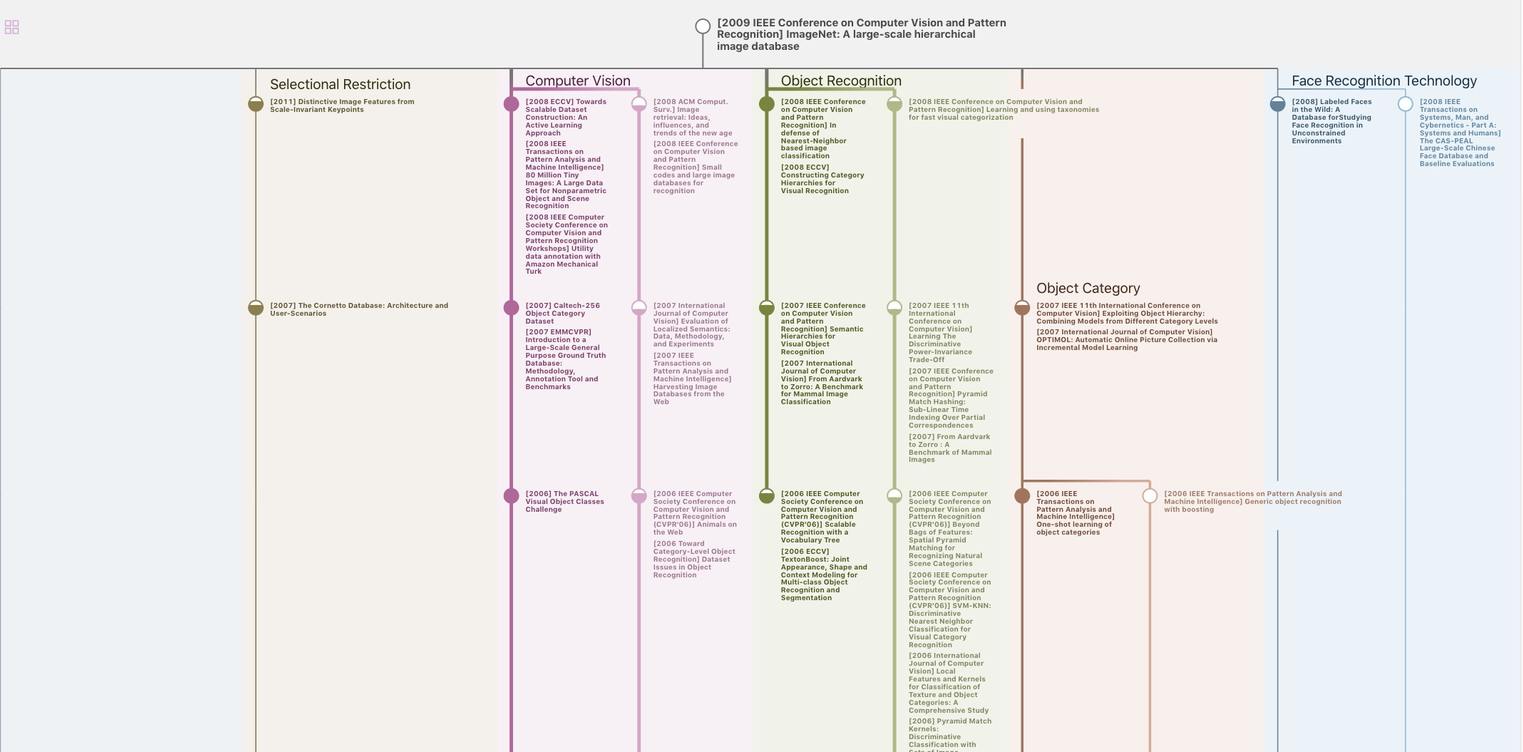
生成溯源树,研究论文发展脉络
Chat Paper
正在生成论文摘要