AutoML and Neural Architecture Search for Gaze Estimation
2022 IEEE 16th International Symposium on Applied Computational Intelligence and Informatics (SACI)(2022)
摘要
This paper focuses on employing the AutoKeras neural architecture search tool and Automatic Machine Learning (AutoML) methods to find an optimal gaze estimation system. The Network Architecture Search (NAS) means automatically tuning already existing deep neural network configurations using a dataset of interest. The algorithm will search in the architectural space for a better neural model along with its optimized parameters. In the paper context, an AutoML solution will perform similarly, but using just ‘pure’ ML models. Considering “Appearance-based Gaze Estimation in the Wild” (MPIIGaze) and “Columbia Gaze Data Set” (CAVE) datasets, the experiments showed results comparable to those of with manually designed models.
更多查看译文
关键词
eye-gaze tracking,gaze estimation,neural architecture search,AutoKeras,AutoML
AI 理解论文
溯源树
样例
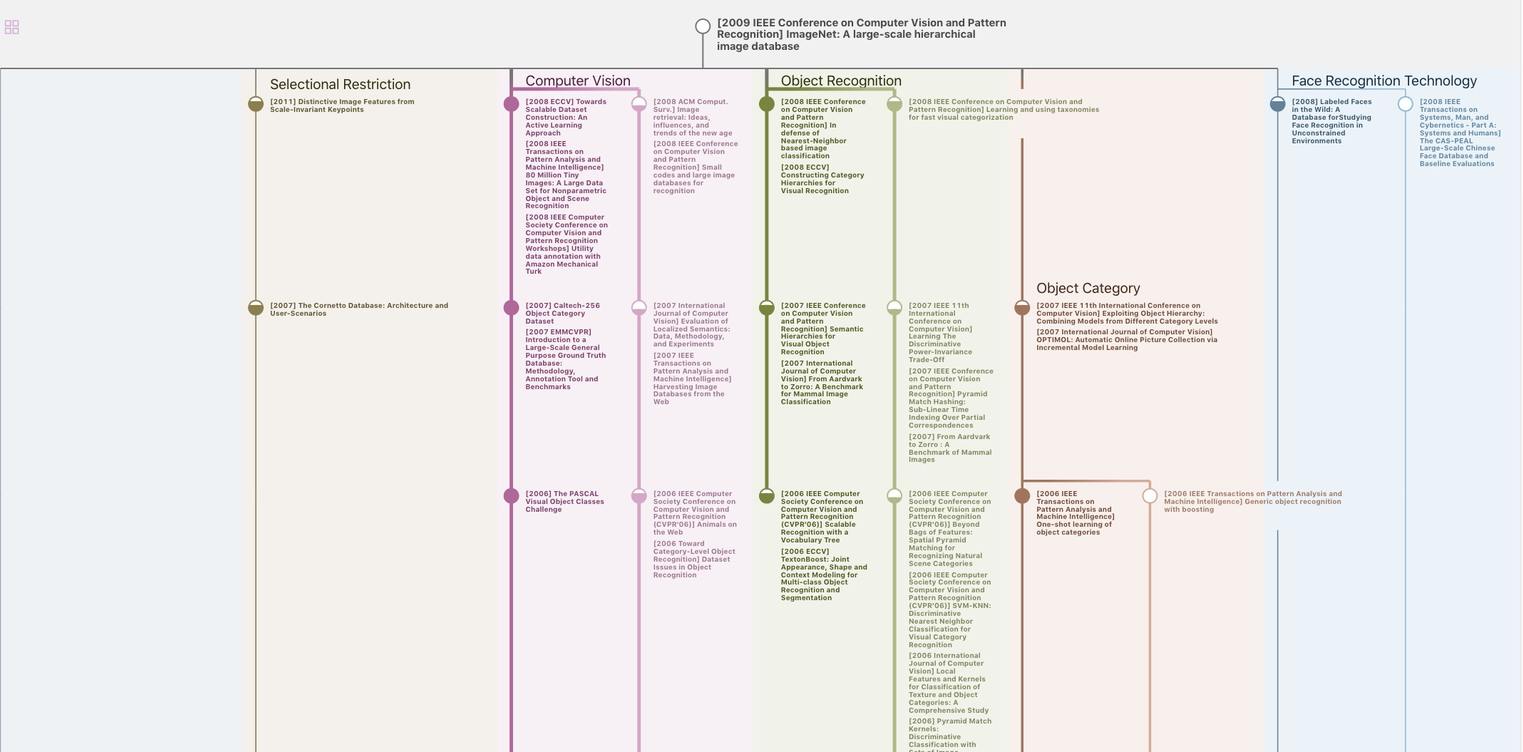
生成溯源树,研究论文发展脉络
Chat Paper
正在生成论文摘要