Variational inference accelerates accurate DNA mixture deconvolution
FORENSIC SCIENCE INTERNATIONAL-GENETICS(2023)
摘要
We investigate a class of DNA mixture deconvolution algorithms based on variational inference, and we show that this can significantly reduce computational runtimes with little or no effect on the accuracy and precision of the result. In particular, we consider Stein Variational Gradient Descent (SVGD) and Variational Inference (VI) with an evidence lower-bound objective. Both provide alternatives to the commonly used Markov-Chain Monte-Carlo methods for estimating the model posterior in Bayesian probabilistic genotyping. We demonstrate that both SVGD and VI significantly reduce computational costs over the current state of the art. Importantly, VI does so without sacrificing precision or accuracy, presenting an overall improvement over previously published methods.
更多查看译文
关键词
Probabilistic genotyping,Stein variational gradient descent,Variational inference,Bayesian inference,Runtime,Precision
AI 理解论文
溯源树
样例
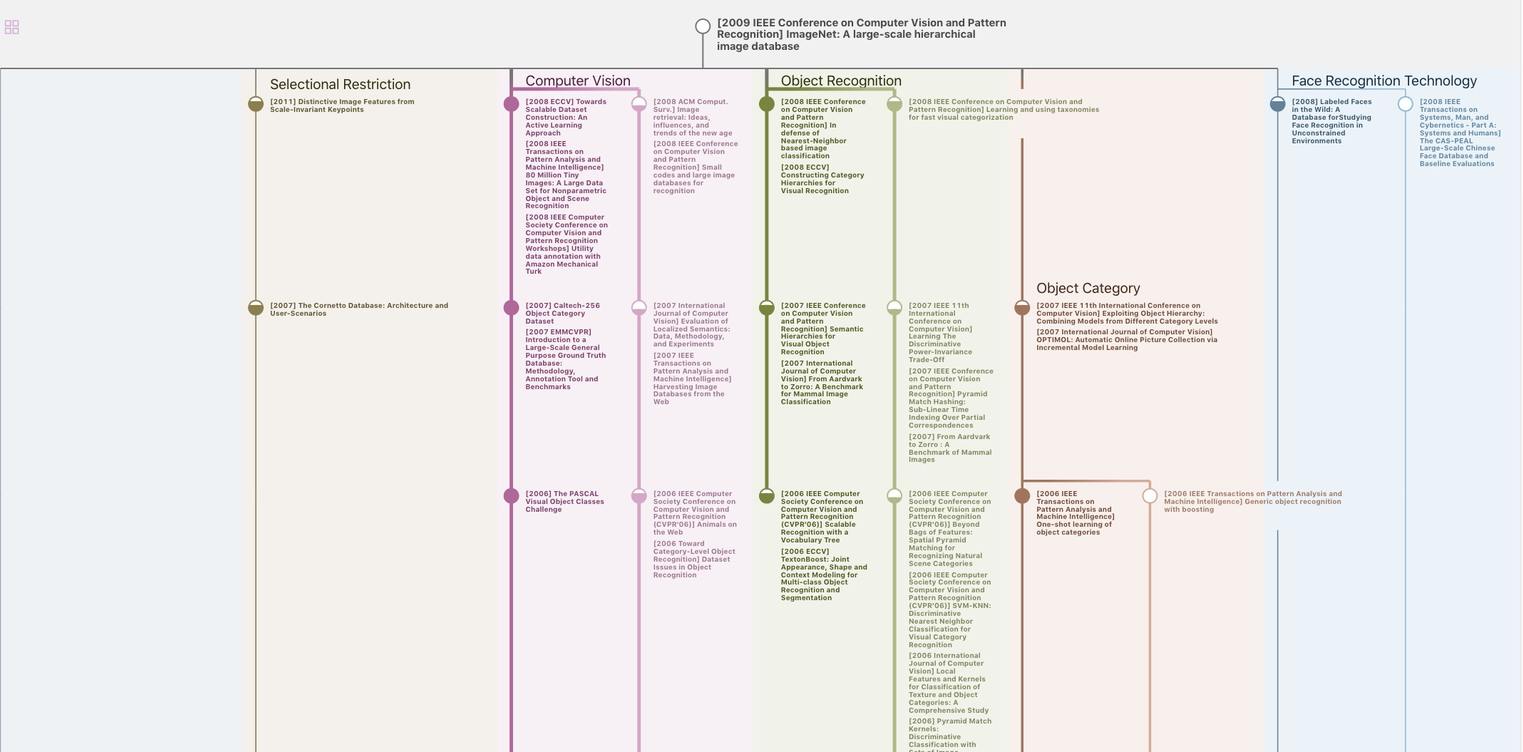
生成溯源树,研究论文发展脉络
Chat Paper
正在生成论文摘要