Automated lesion detection of breast cancer in [F-18] FDG PET/CT using a novel AI-Based workflow
Frontiers in oncology(2022)
摘要
Applications based on artificial intelligence (AI) and deep learning (DL) are rapidly being developed to assist in the detection and characterization of lesions on medical images. In this study, we developed and examined an image-processing workflow that incorporates both traditional image processing with AI technology and utilizes a standards-based approach for disease identification and quantitation to segment and classify tissue within a whole-body [F-18]FDG PET/CT study. MethodsOne hundred thirty baseline PET/CT studies from two multi-institutional preoperative clinical trials in early-stage breast cancer were semi-automatically segmented using techniques based on PERCIST v1.0 thresholds and the individual segmentations classified as to tissue type by an experienced nuclear medicine physician. These classifications were then used to train a convolutional neural network (CNN) to automatically accomplish the same tasks. ResultsOur CNN-based workflow demonstrated Sensitivity at detecting disease (either primary lesion or lymphadenopathy) of 0.96 (95% CI [0.9, 1.0], 99% CI [0.87,1.00]), Specificity of 1.00 (95% CI [1.0,1.0], 99% CI [1.0,1.0]), DICE score of 0.94 (95% CI [0.89, 0.99], 99% CI [0.86, 1.00]), and Jaccard score of 0.89 (95% CI [0.80, 0.98], 99% CI [0.74, 1.00]). ConclusionThis pilot work has demonstrated the ability of AI-based workflow using DL-CNNs to specifically identify breast cancer tissue as determined by [F-18]FDG avidity in a PET/CT study. The high sensitivity and specificity of the network supports the idea that AI can be trained to recognize specific tissue signatures, both normal and disease, in molecular imaging studies using radiopharmaceuticals. Future work will explore the applicability of these techniques to other disease types and alternative radiotracers, as well as explore the accuracy of fully automated and quantitative detection and response assessment.
更多查看译文
关键词
artificial intelligence, machine learning, deep learning, PERCIST v1, 0, breast cancer
AI 理解论文
溯源树
样例
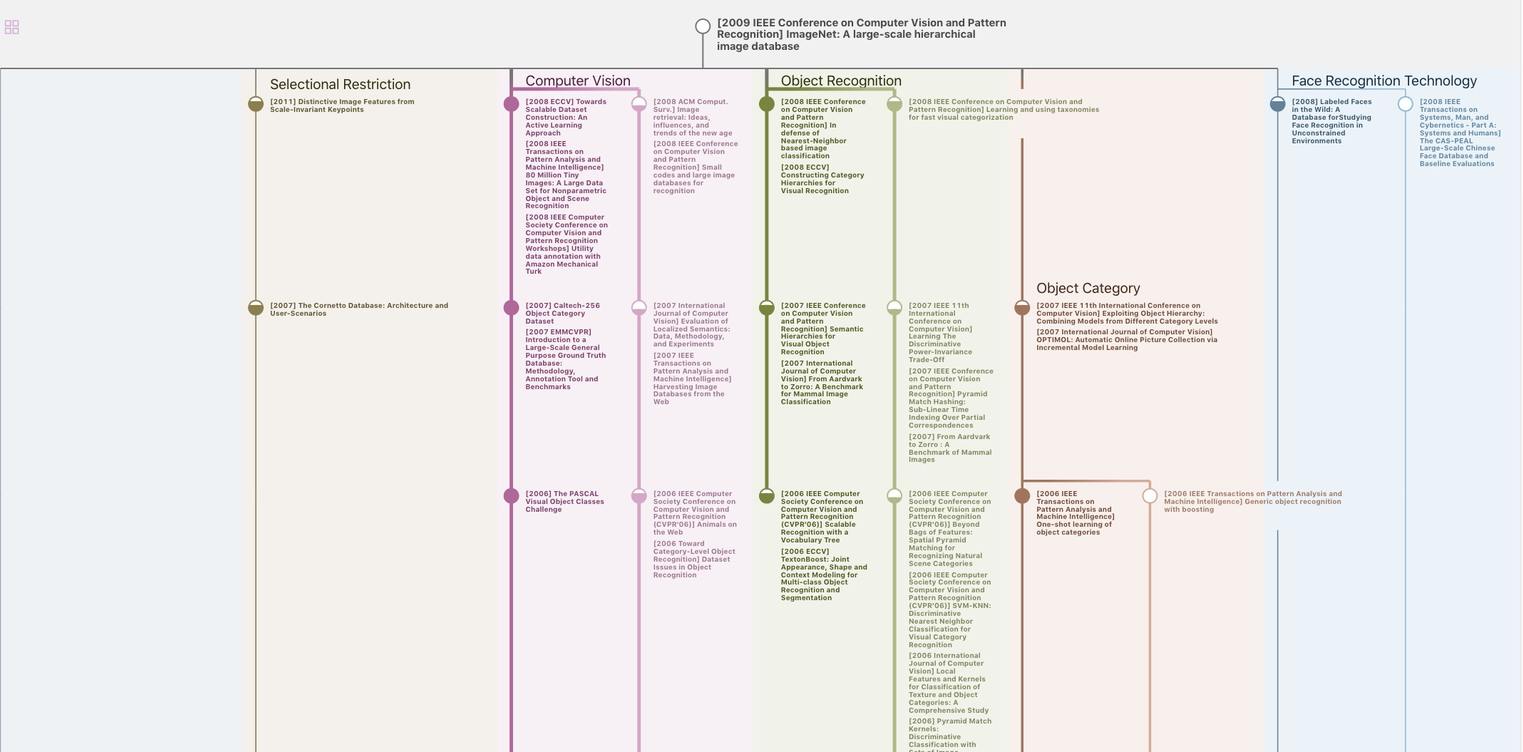
生成溯源树,研究论文发展脉络
Chat Paper
正在生成论文摘要