RIPPLE: Concept-Based Interpretation for Raw Time Series Models in Education
arxiv(2023)
摘要
Time series is the most prevalent form of input data for educational prediction tasks. The vast majority of research using time series data focuses on hand-crafted features, designed by experts for predictive performance and interpretability. However, extracting these features is labor-intensive for humans and computers. In this paper, we propose an approach that utilizes irregular multivariate time series modeling with graph neural networks to achieve comparable or better accuracy with raw time series clickstreams in comparison to hand-crafted features. Furthermore, we extend concept activation vectors for interpretability in raw time series models. We analyze these advances in the education domain, addressing the task of early student performance prediction for downstream targeted interventions and instructional support. Our experimental analysis on 23 MOOCs with millions of combined interactions over six behavioral dimensions show that models designed with our approach can (i) beat state-of-the-art educational time series baselines with no feature extraction and (ii) provide interpretable insights for personalized interventions. Source code: https://github.com/epfl-ml4ed/ripple/.
更多查看译文
关键词
raw time series models,time series,education,concept-based
AI 理解论文
溯源树
样例
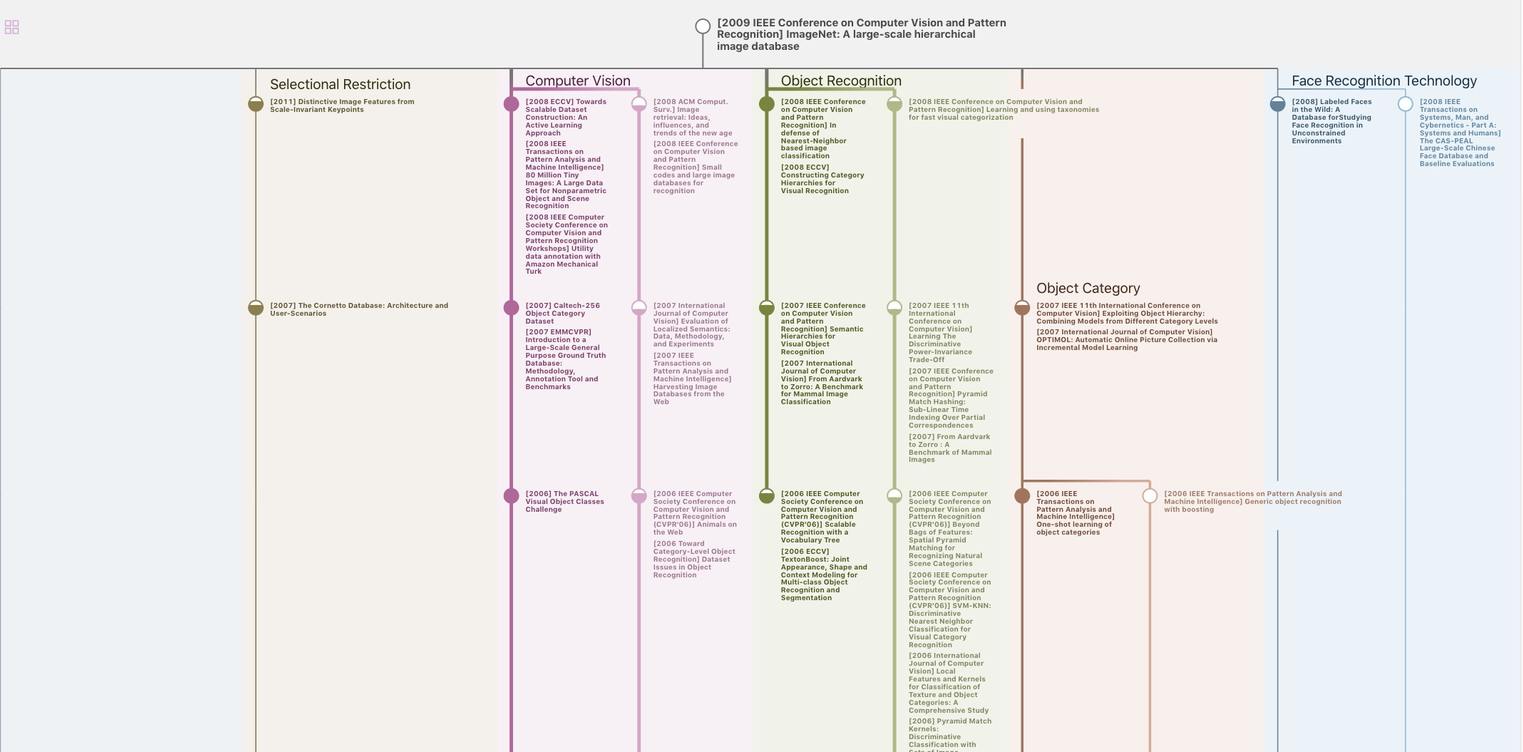
生成溯源树,研究论文发展脉络
Chat Paper
正在生成论文摘要