Augmented Regularity for Efficient Video Anomaly Detection: An edge AI application
2022 IEEE 42nd International Conference on Distributed Computing Systems Workshops (ICDCSW)(2022)
摘要
Video anomaly detection, as a critical edge AI application, may dramatically minimize transmission burden by transmitting just anomalous data. Traditionally, dense consecutive frames with high resolution are utilized as the input to assure that video anomaly detection performed well. Dense input with high resolution, on the other hand, will result in high computation. To address the demand of high performance with little computation on edge devices, we propose an efficient video anomaly detection based on augmented regularity with mutual learning. Sparse frames collected from every two frames with a low-resolution of 160 × 160 are used as the input to decrease processing. Generally, performance will be hampered as a result of the low-quality inputs. To improve performance, an auxiliary network is created that uses dense inputs to mine plentiful patterns from successive frames and promotes the proposed network throughout the training phase via mutual learning. Additionally, we design augmented regularity to increase scene generalization when edge devices are grouped in distributed applications with various scenes. During the training phase, the augmented regularity, which is irrelevant to the input video, is concatenated in the input as a hidden label message. The label message infers that the inputs are normal. In the inference phase, the abnormal information can be detected from the hidden label message through generated errors. Experimental results on benchmark datasets demonstrate that the proposed method can achieve the state-of-the-art at a super-real-time speed of 80fps.
更多查看译文
关键词
anomaly detection,augmented regularity,mutual learning,edge application
AI 理解论文
溯源树
样例
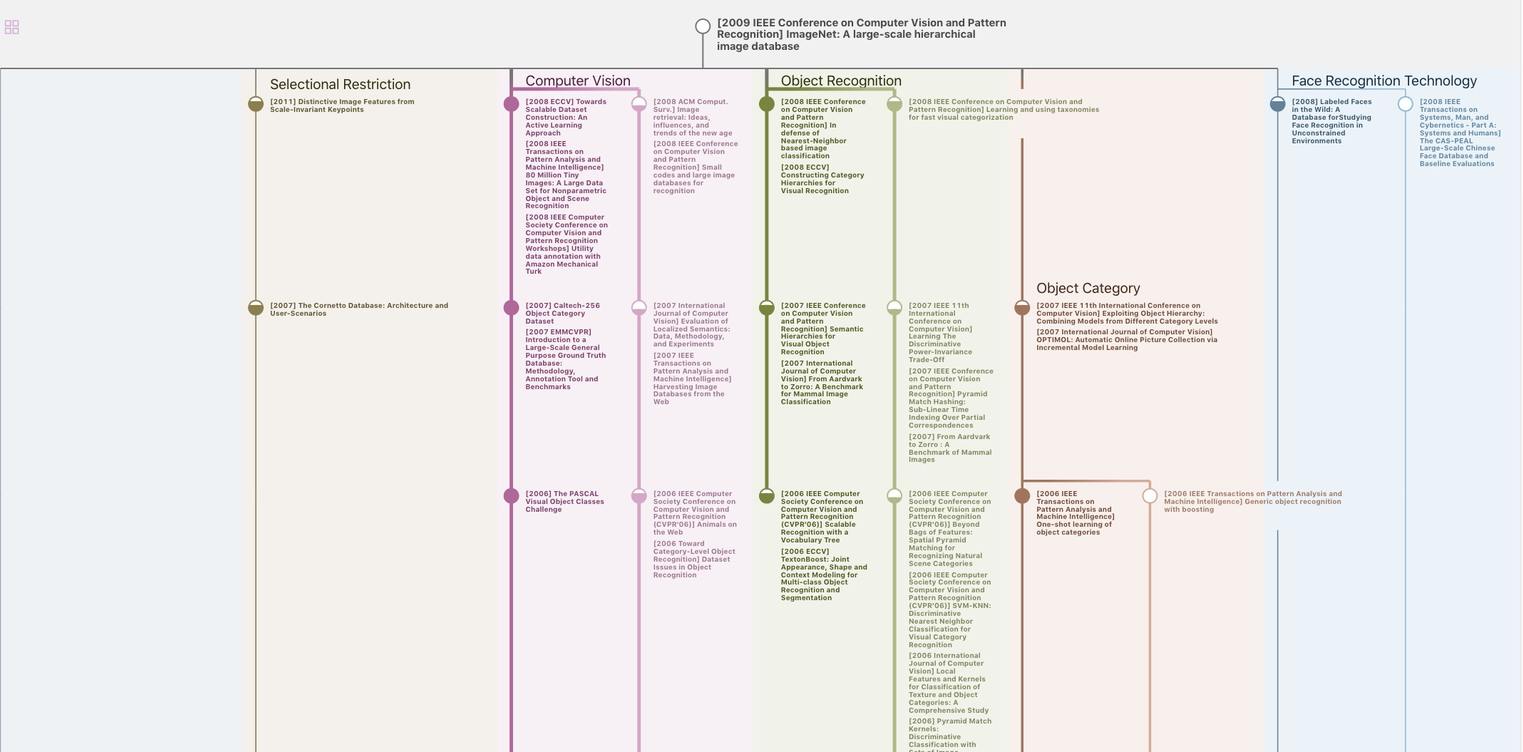
生成溯源树,研究论文发展脉络
Chat Paper
正在生成论文摘要