Temperature Annealing Knowledge Distillation from Averaged Teacher
2022 IEEE 42nd International Conference on Distributed Computing Systems Workshops (ICDCSW)(2022)
摘要
Despite the success of deep neural networks (DNNs) in almost every field, their deployment on edge devices has been restricted due to the significant memory and computational resource requirements. Among various model compression techniques for DNNs, Knowledge Distillation (KD) is a simple but effective one, which transfers the knowledge of a large teacher model to a smaller student model. However, as pointed out in the literature, the student is unable to mimic the teacher perfectly even when it has sufficient capacity. As a result, the student may not be able to retain the teacher's accuracy. What's worse, the student performance may be impaired by the wrong knowledge and potential over- regularization effect of the teacher. In this paper, we propose a novel method TAKDAT which is short for Temperature Annealing Knowledge Distillation from A veraged Teacher. Specifically, TAKDAT comprises of two con-tributions: 1) we propose to use an averaged teacher, which is an equally weighted average of model checkpoints traversed by SGD, in the distillation. Compared to a normal teacher, an averaged teacher provides richer similarity information and has less wrong knowledge; 2) we propose a temperature annealing scheme to gradually reduce the regularization effect of the teacher. Finally, extensive experiments verify the effectiveness of TAKDAT, e.g., it achieves a test accuracy of 74.31 % on CIFARI00 for ResNet32.
更多查看译文
AI 理解论文
溯源树
样例
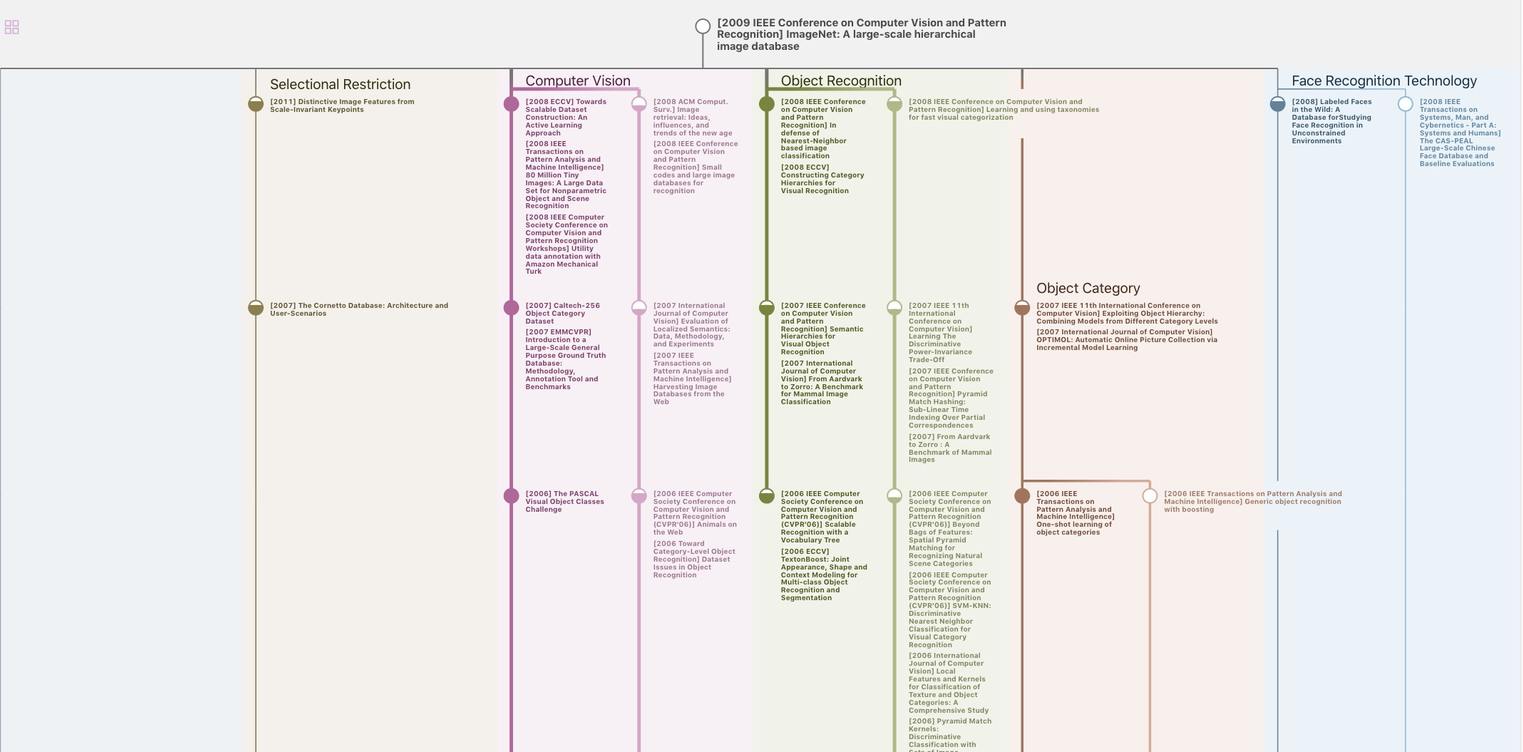
生成溯源树,研究论文发展脉络
Chat Paper
正在生成论文摘要