I Have Seen that Before: Memory Augmented Neural Network for Learning Affordances by Analogy
2022 IEEE International Conference on Development and Learning (ICDL)(2022)
摘要
Humans show a remarkable ability to quickly adapt to new situations without forgetting past ones. Existing learning methods still face problems with catastrophic forgetting when learning about new situations and contexts while taking many iterations to adjust to new input and output pairs. In this work, we propose the application of a Memory augmented network to the problem of learning tool affordances. We consider a network that explicitly indexes an episodic memory of past experiences and retrieves samples of past experience to reason about new situations by analogy, in an approach we call affordances by analogy. The work takes advantage of a tool-object interaction dataset to learn affordances. Our experiments show the model outperforms the baselines in the low sample regime and retains information better when re-trained on a different data distribution. Hinting at a promising direction, this work could enable learning algorithms to retain information better.
更多查看译文
AI 理解论文
溯源树
样例
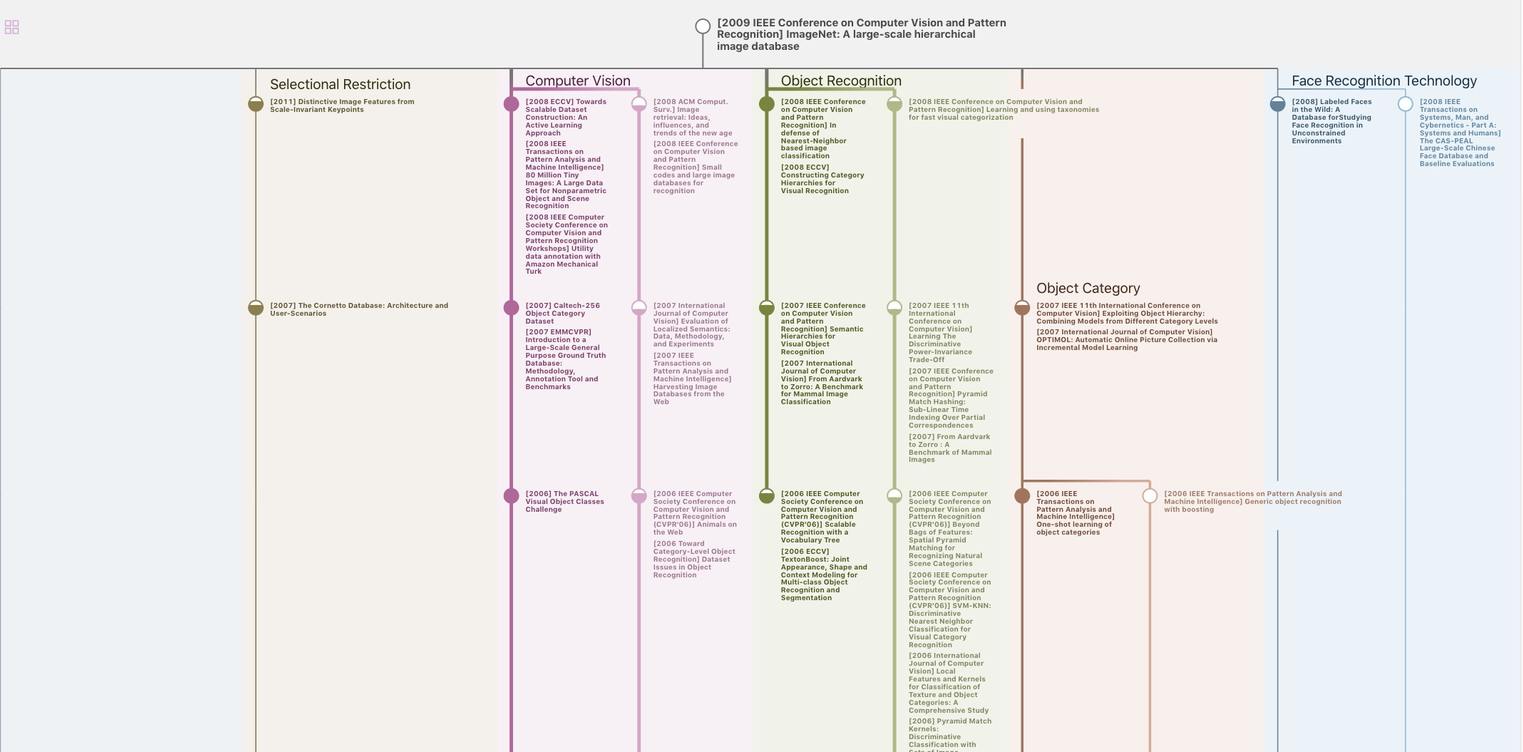
生成溯源树,研究论文发展脉络
Chat Paper
正在生成论文摘要