Lagrange Coded Federated Learning (L-CoFL) Model for Internet of Vehicles
2022 IEEE 42nd International Conference on Distributed Computing Systems (ICDCS)(2022)
摘要
In Internet-of-Vehicles (IoV), smart vehicles can efficiently process various sensing data through federated learning (FL) - a privacy-preserving distributed machine learning (ML) approach that allows collaborative development of the shared ML model without any data exchange. However, traditional FL approaches suffer from poor security against the system noise, e.g., due to low-quality trained data, wireless channel errors, and malicious vehicles generating erroneous results, which affects the accuracy of the developed ML model. To address this problem, we propose a novel FL model based on the concept of Lagrange coded computing (LCC) - a coded distributed computing (CDC) scheme that enables enhancing the system security. In particular, we design the first L-CoFL (Lagrange coded FL) model to improve the accuracy of FL computations in the presence of lowquality trained data and wireless channel errors, and guarantee the system security against malicious vehicles. We apply the proposed L-CoFL model to predict the traffic slowness in IoV and verify the superior performance of our model through extensive simulations.
更多查看译文
关键词
Coded distributed computing (CDC),data privacy,federated learning,Internet of Vehicles (IoV),machine learning,security
AI 理解论文
溯源树
样例
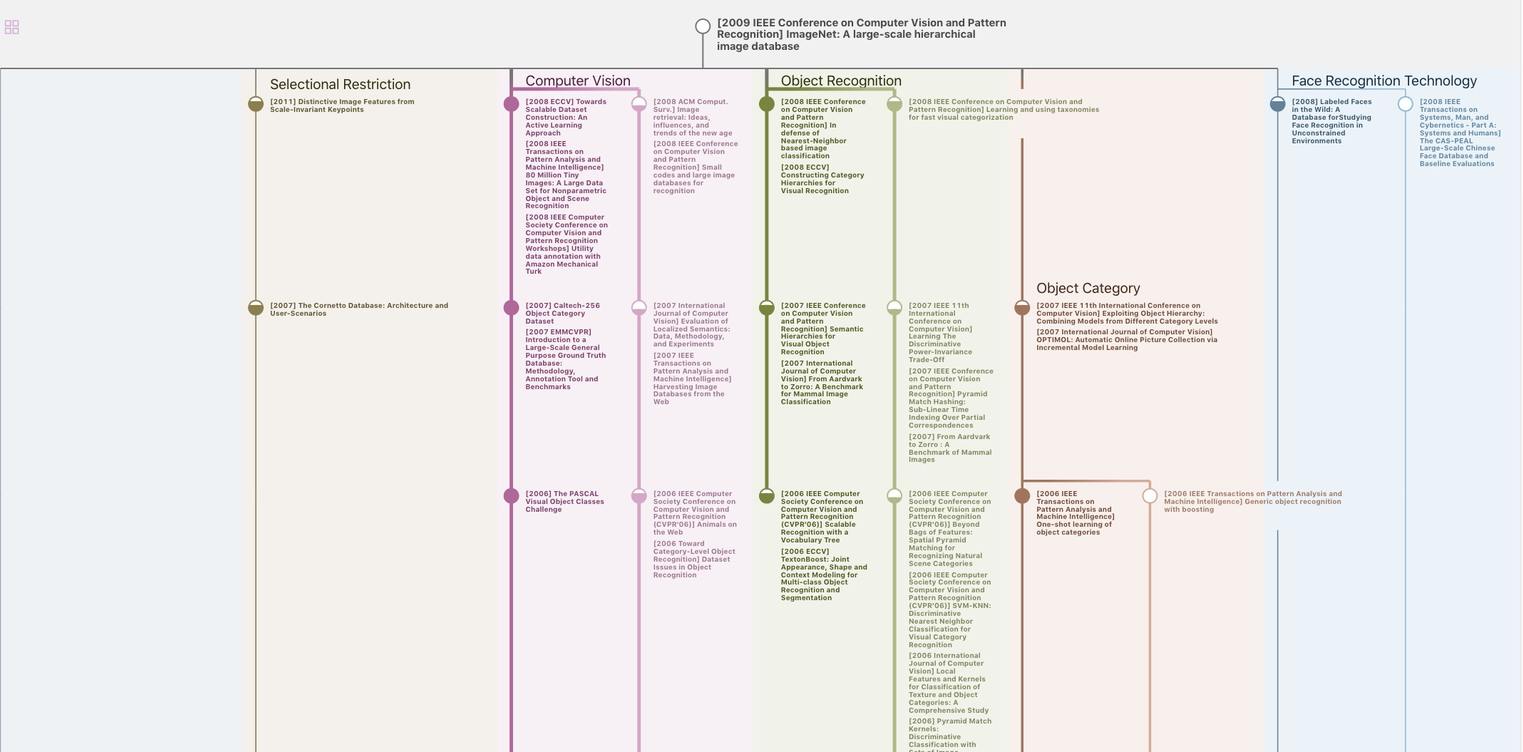
生成溯源树,研究论文发展脉络
Chat Paper
正在生成论文摘要