COVERED, CollabOratiVE Robot Environment Dataset for 3D Semantic segmentation
2022 IEEE 27th International Conference on Emerging Technologies and Factory Automation (ETFA)(2022)
摘要
Safe human-robot collaboration (HRC) has recently gained a lot of interest with the emerging Industry 5.0 paradigm. Conventional robots are being replaced with more intelligent and flexible collaborative robots (cobots). Safe and efficient collaboration between cobots and humans largely relies on the cobot’s comprehensive semantic understanding of the dynamic surrounding of industrial environments. Despite the importance of semantic understanding for such applications, 3D semantic segmentation of collaborative robot workspaces lacks sufficient research and dedicated datasets. The performance limitation caused by insufficient datasets is called ’data hunger’ problem. To overcome this current limitation, this work develops a new dataset specifically designed for this use case, named "COV-ERED", which includes point-wise annotated point clouds of a robotic cell. Lastly, we also provide a benchmark of current state-of-the-art (SOTA) algorithm performance on the dataset and demonstrate a real-time semantic segmentation of a collaborative robot workspace using a multi-LiDAR system. The promising results from using the trained Deep Networks on a real-time dynamically changing situation shows that we are on the right track. Our perception pipeline achieves 20Hz throughput with a prediction point accuracy of >96% and >92% mean intersection over union (mIOU) while maintaining an 8Hz throughput.
更多查看译文
关键词
Multi-LiDAR,dataset,Semantic understanding,Cobots,Data hunger,Real industrial environment
AI 理解论文
溯源树
样例
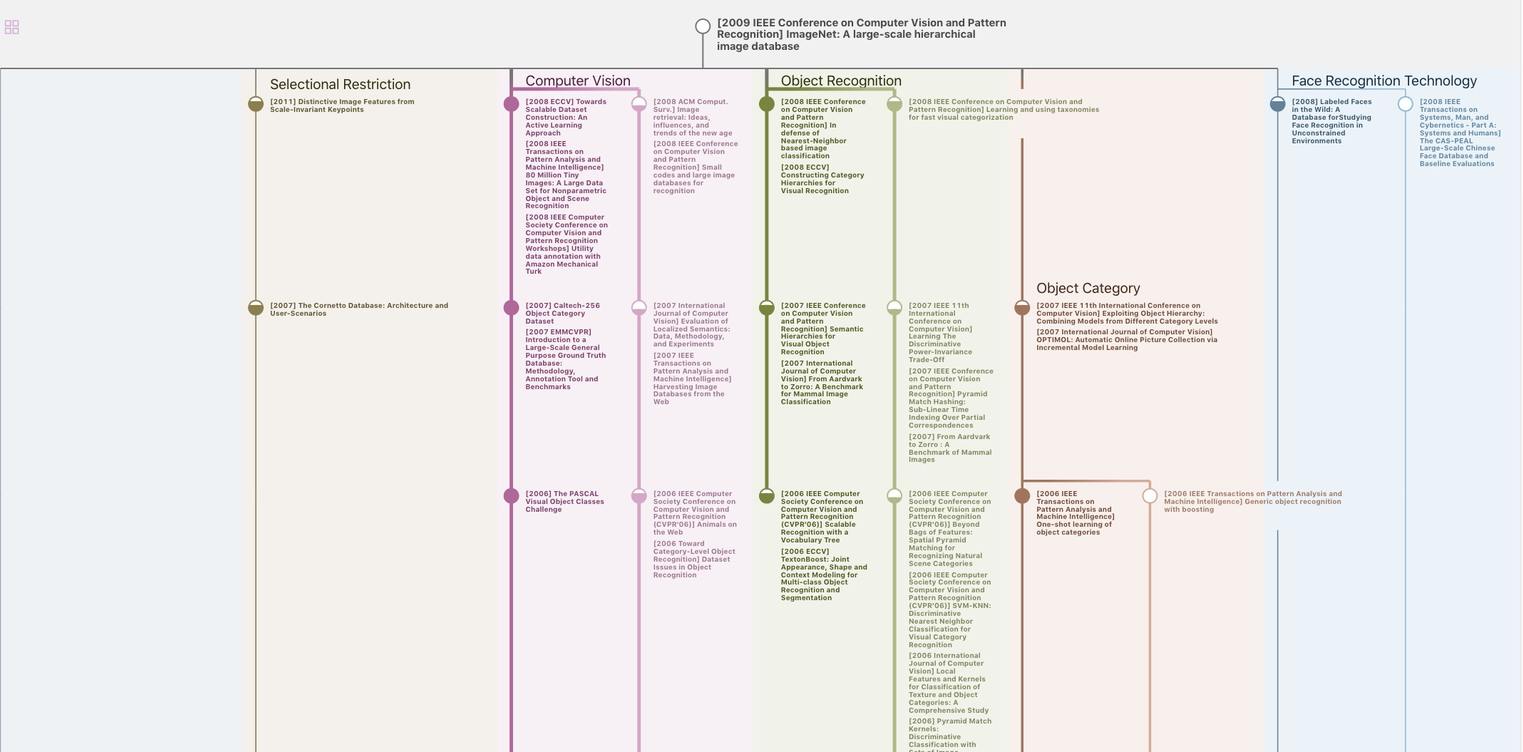
生成溯源树,研究论文发展脉络
Chat Paper
正在生成论文摘要