Projection-aware Deep Neural Network for DC Optimal Power Flow Without Constraint Violations
2022 IEEE International Conference on Communications, Control, and Computing Technologies for Smart Grids (SmartGridComm)(2022)
摘要
Solving optimal power flow is essential to ensure stable and cost effective power system operations. However, frequently solving optimal power flow under numerous scenarios using conventional solvers suffers from high computational complexity due to the large-scale power network. As a substitute, using deep neural network to solve optimal power flow draws researchers' attention. Even though deep neural network is beneficial for reducing the computational time of solving optimal power flow, its outputs often violate physical constraints of the power network. To overcome the constraint violations of deep neural network, we propose projection-aware deep neural network (PA-DNN) for solving optimal power flow. To the best of our knowledge, this is the first paper that guarantees no constraint violations of DC optimal power flow using deep neural network. The proposed PA-DNN takes active power demand as an input and has a projection layer at the final layer. Through the projection layer, hidden vectors are projected onto a feasible region. Then, by minimizing error between the projected vector and optimal active power generation, PA-DNN learns to predict accurate generation dispatch. Simulation results on various PGLib-OPF networks show that PA-DNN achieves nearly zero optimality gap with no constraint violations using only 15 % of the training data of the baseline.
更多查看译文
关键词
Deep learning,optimal power flow,DC-OPF,zero-constraint violations
AI 理解论文
溯源树
样例
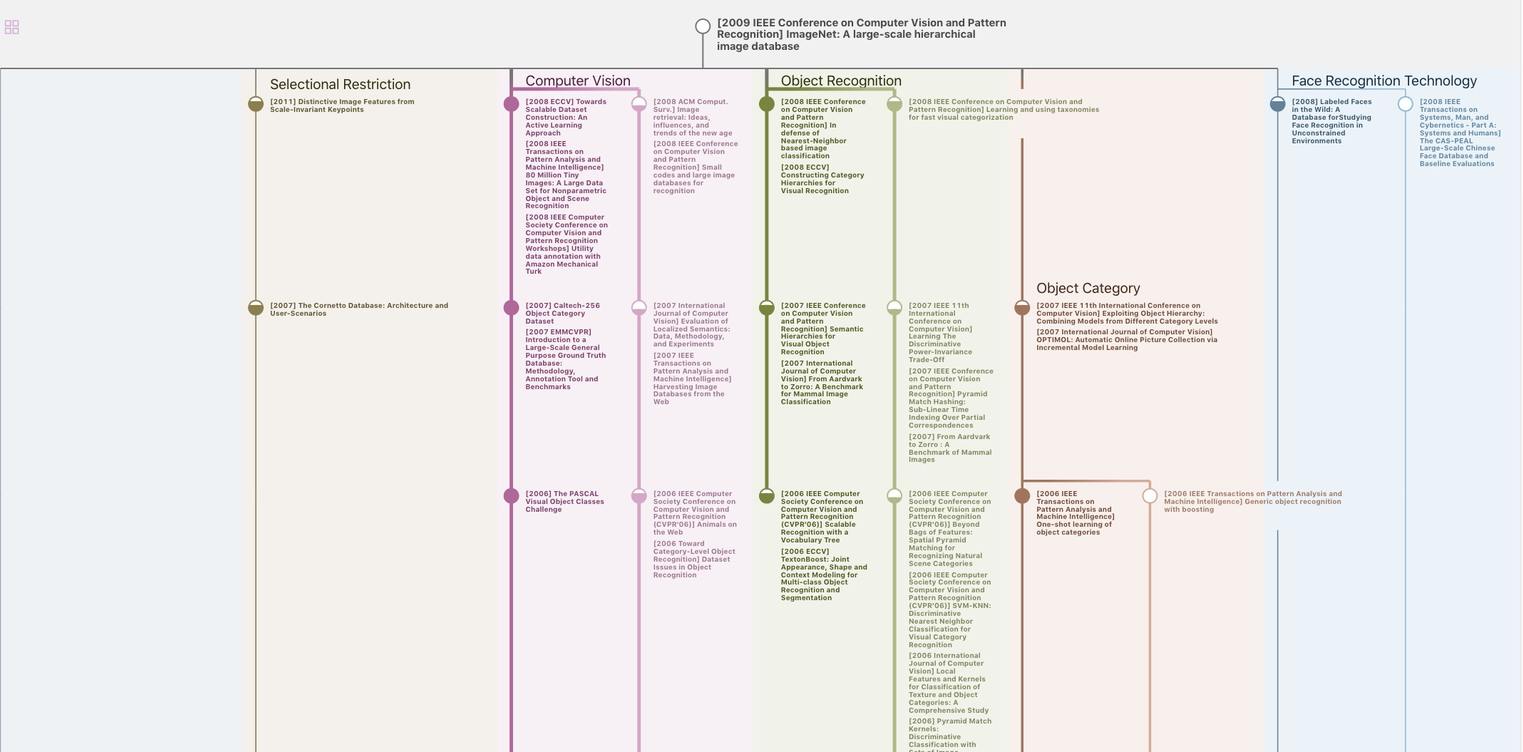
生成溯源树,研究论文发展脉络
Chat Paper
正在生成论文摘要