Comparative Analysis of Generative Adversarial Network-Based Single-Image Super-Resolution Approaches
Emerging Technology Trends in Electronics, Communication and Networking(2022)
摘要
In the past decade, a convolutional neural network that can work on image became most popular since there were many powerful GPUs and datasets available to work. Convolutional neural network advanced the performance in various image processing tasks. In single-image-based super-resolution, convolutional neural network predicated methods have obtained remarkable performance in terms of error quantification (i.e., PSNR and SSIM) than earlier traditional machine learning predicated methods. Even though achieving better error measurements, the super-resolution results look blurry in appearance because of the classical
$$L_1$$
or
$$L_2$$
loss functions that have been used in the training process. Recently, generative adversarial network has been used to obtain a super-resolve image with better perceptual quality as compared to a convolutional neural network. In this manuscript, the technique and performance of those generative adversarial networks for single-image super-resolution approaches are compared for upscaling factor
$$\times \, 4$$
.
更多查看译文
关键词
Single-image super-resolution, Convolutional neural network, Generative adversarial network
AI 理解论文
溯源树
样例
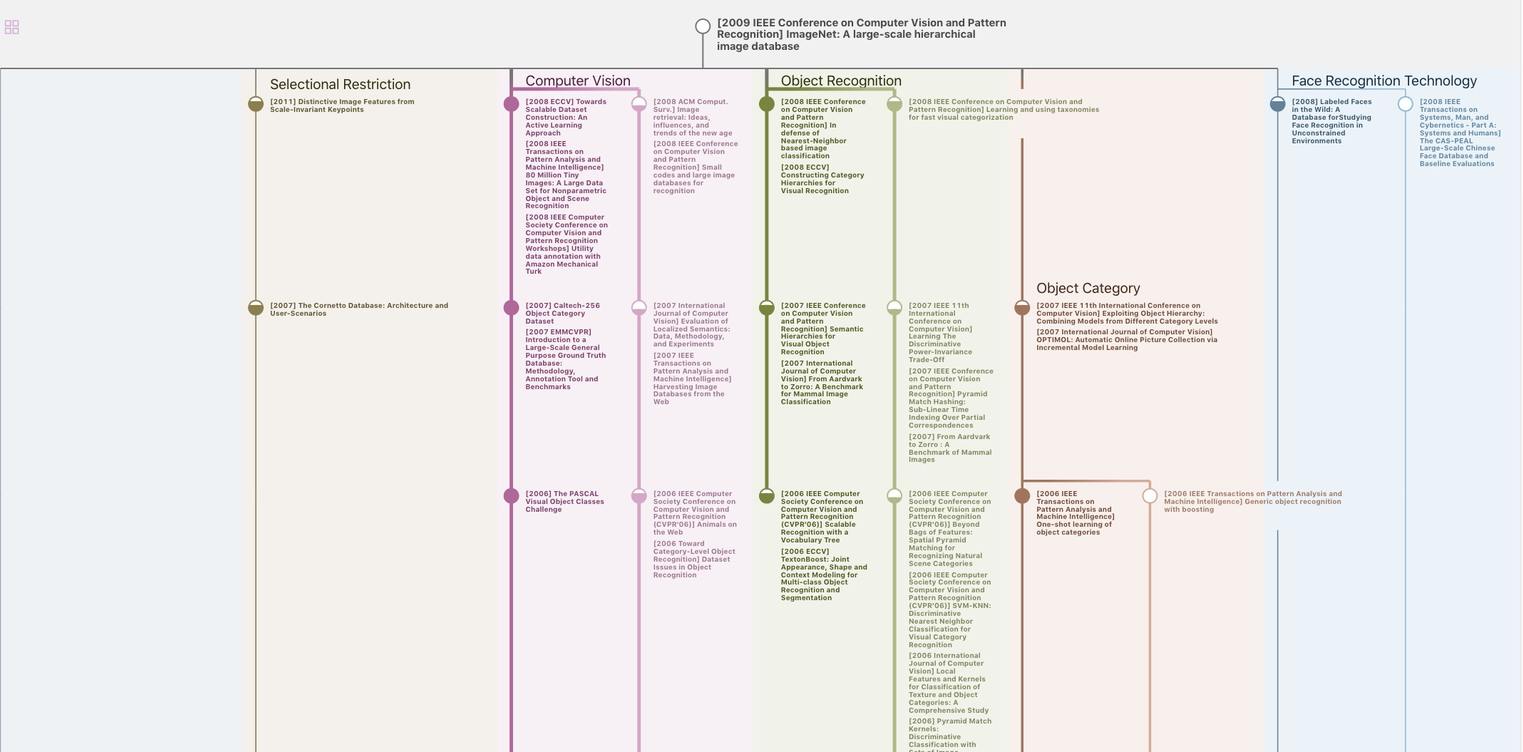
生成溯源树,研究论文发展脉络
Chat Paper
正在生成论文摘要