Spatiotemporal Prediction of Nitrogen Dioxide Based on Graph Neural Networks
Advances and New Trends in Environmental Informatics(2022)
摘要
Air quality prediction, especially spatiotemporal prediction, is still a challenging issue. Considering the impact of numerous factors on air quality causes difficulties in integrating these factors in a spatiotemporal dimension and developing a model to make efficient predictions. At the same time, machine learning and deep learning development bring advanced approaches to addressing these challenges and propose novel solutions. The current work introduces one of the most advanced methods, an attention temporal graph convolutional network, which was implemented on datasets constructed by combining air quality, meteorological and traffic data on a spatiotemporal axis. The datasets were obtained from the city of Madrid for the periods January-June 2019 and January–June 2020. The evaluation metrics, the Root Mean Square Error and the Mean Absolute Error confirmed the proposed model’s advantages compared with long short-term memory (reference model). Particularly, it outperformed the latter method by 14.18% and 3.78%, respectively.
更多查看译文
关键词
Graph neural network, Attention temporal graph convolutional network, Spatiotemporal prediction, Nitrogen dioxide prediction
AI 理解论文
溯源树
样例
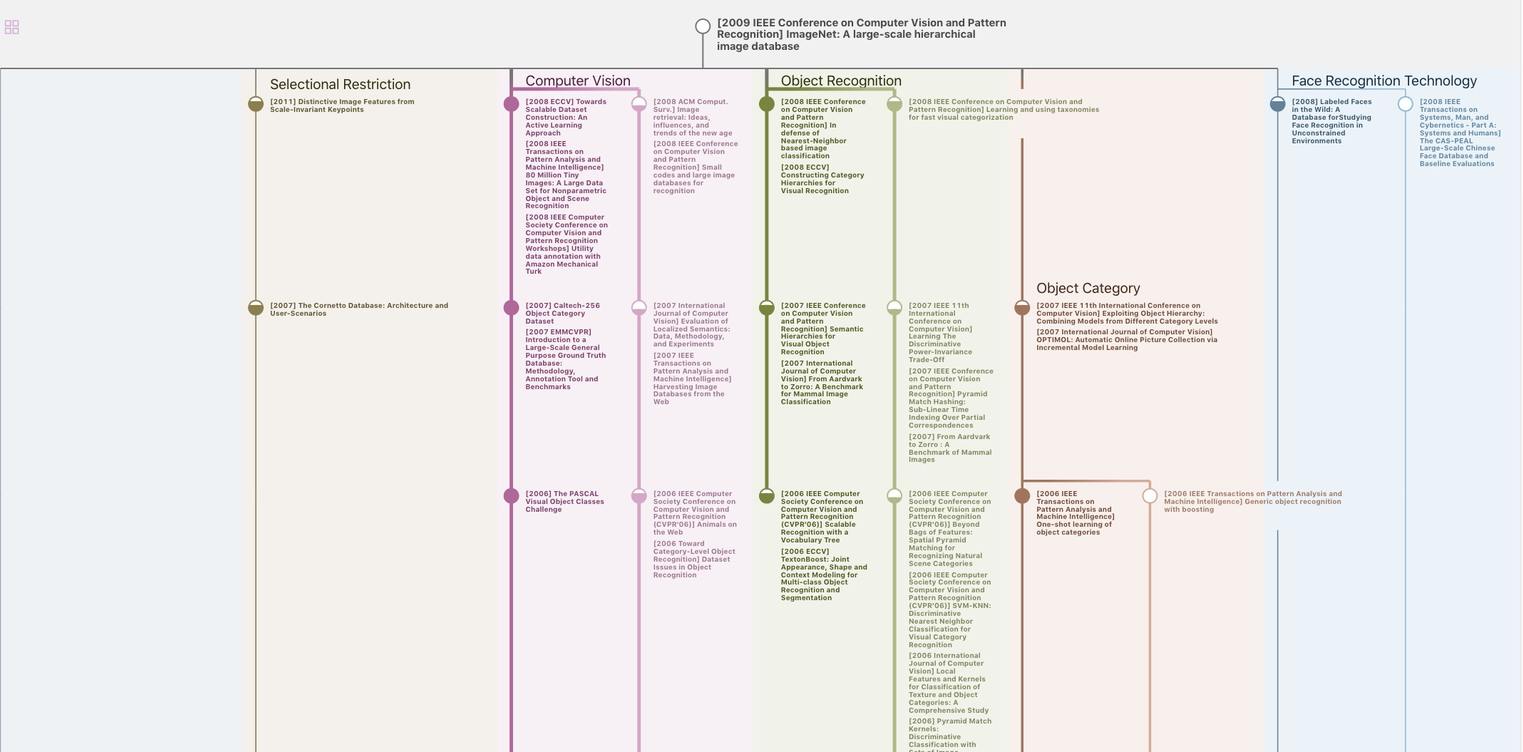
生成溯源树,研究论文发展脉络
Chat Paper
正在生成论文摘要