Distribution Fitting for Combating Mode Collapse in Generative Adversarial Networks.
arxiv(2023)
摘要
Mode collapse is a significant unsolved issue of generative adversarial networks (GANs). In this work, we examine the causes of mode collapse from a novel perspective. Due to the nonuniform sampling in the training process, some subdistributions may be missed when sampling data. As a result, even when the generated distribution differs from the real one, the GAN objective can still achieve the minimum. To address the issue, we propose a global distribution fitting (GDF) method with a penalty term to confine the generated data distribution. When the generated distribution differs from the real one, GDF will make the objective harder to reach the minimal value, while the original global minimum is not changed. To deal with the circumstance when the overall real data is unreachable, we also propose a local distribution fitting (LDF) method. Experiments on several benchmarks demonstrate the effectiveness and competitive performance of GDF and LDF.
更多查看译文
关键词
Distribution fitting,generative adversarial nets,mode collapse,nonuniform sampling
AI 理解论文
溯源树
样例
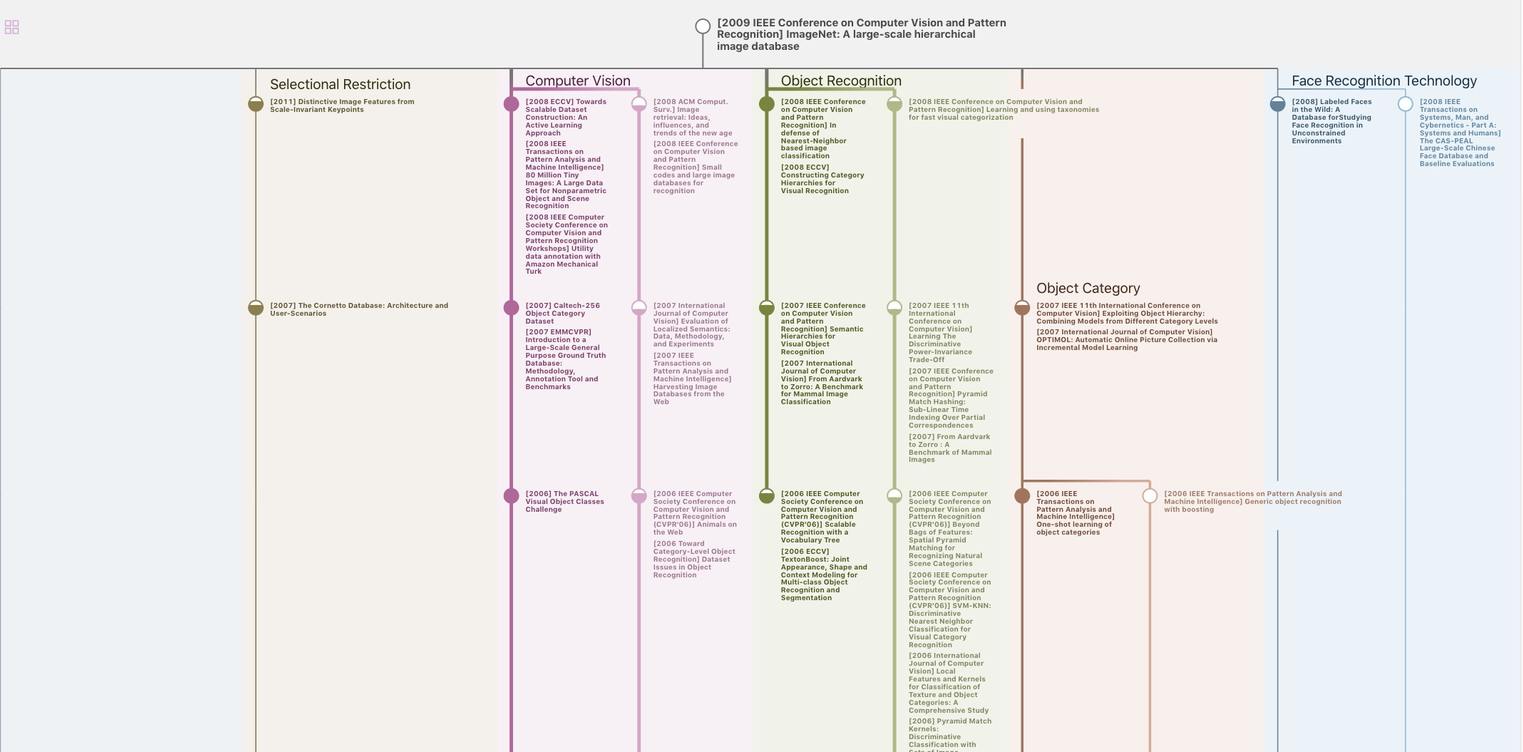
生成溯源树,研究论文发展脉络
Chat Paper
正在生成论文摘要