Interpretable Node Representation with Attribute Decoding
arxiv(2022)
摘要
Variational Graph Autoencoders (VGAEs) are powerful models for unsupervised learning of node representations from graph data. In this work, we systematically analyze modeling node attributes in VGAEs and show that attribute decoding is important for node representation learning. We further propose a new learning model, interpretable NOde Representation with Attribute Decoding (NORAD). The model encodes node representations in an interpretable approach: node representations capture community structures in the graph and the relationship between communities and node attributes. We further propose a rectifying procedure to refine node representations of isolated notes, improving the quality of these nodes' representations. Our empirical results demonstrate the advantage of the proposed model when learning graph data in an interpretable approach.
更多查看译文
关键词
representation
AI 理解论文
溯源树
样例
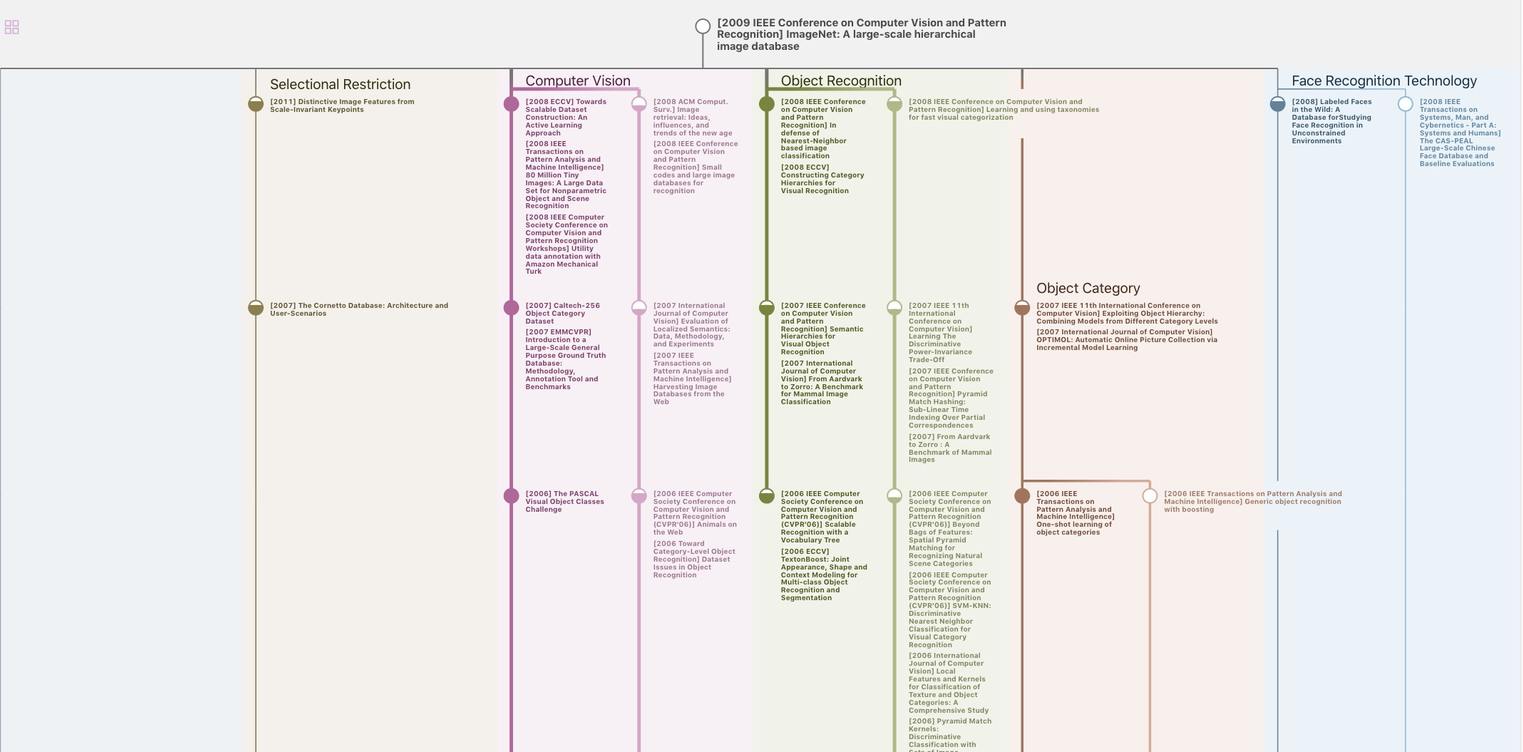
生成溯源树,研究论文发展脉络
Chat Paper
正在生成论文摘要