Sharing Massive Biomedical Data at Magnitudes Lower Bandwidth Using Implicit Neural Function
biorxiv(2022)
摘要
Efficient storage and sharing of massive biomedical data would open up their wide accessibility to different institutions and disciplines. However, compressors tailored for natural photos/videos are rapidly limited for biomedical data, while emerging deep learning based methods demand huge training data and are difficult to generalize. Here we propose to conduct biomedical data compRession with Implicit nEural Function (BRIEF) by representing the original data with compact deep neural networks, which are data specific and thus have no generalization issues. Benefiting from the strong representation capability of implicit neural function, BRIEF achieves significantly higher-fidelity on diverse biomedical data than existing techniques. Besides, BRIEF is of consistent performance across the whole data volume, supports customized spatially-varying fidelity. BRIEF's multi-fold advantageous features also serve reliable downstream tasks at low bandwidth. Our approach will facilitate biomedical data sharing at low bandwidth and maintenance costs, and promote collaboration and progress in the biomedical field.
### Competing Interest Statement
The authors have declared no competing interest.
更多查看译文
关键词
massive biomedical data,neural,magnitudes lower bandwidth,sharing
AI 理解论文
溯源树
样例
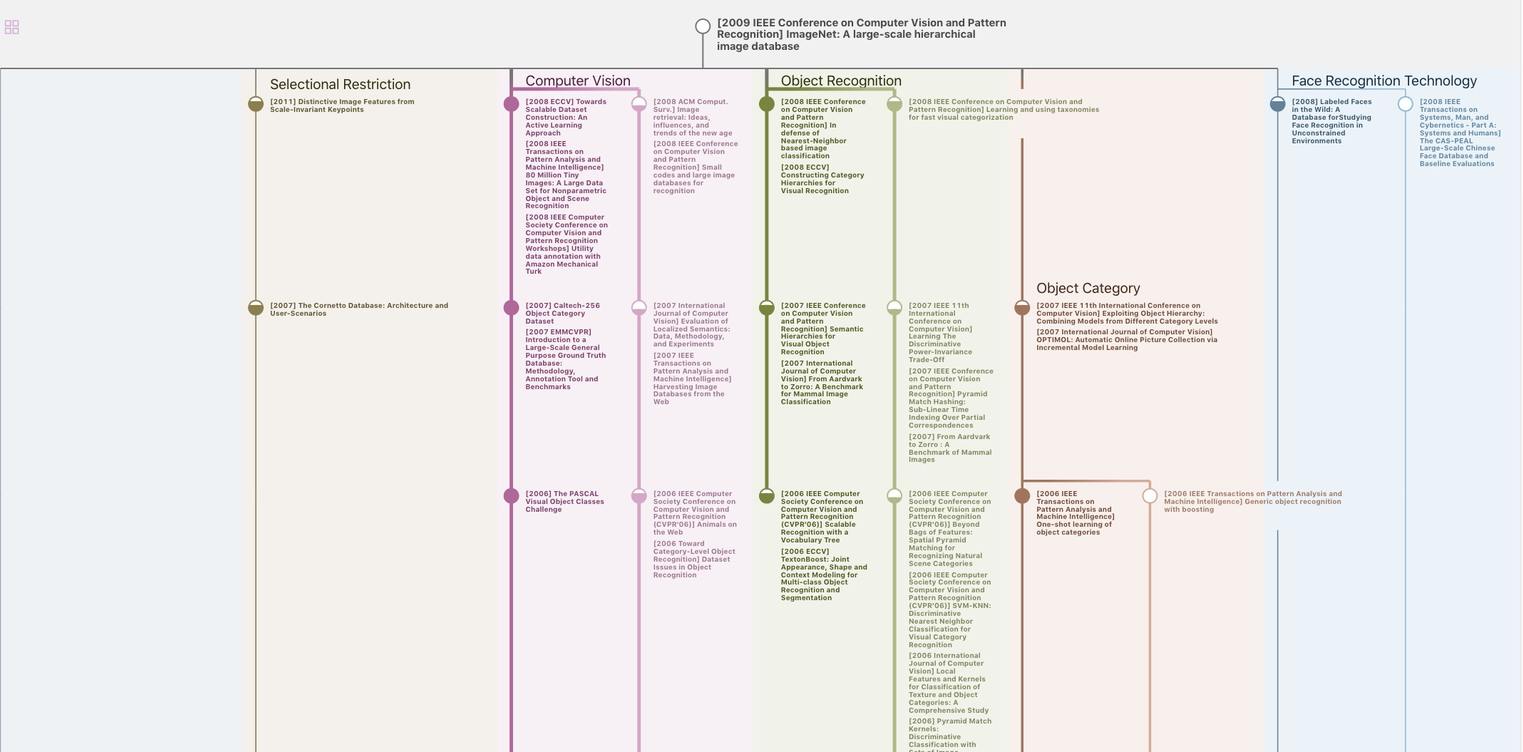
生成溯源树,研究论文发展脉络
Chat Paper
正在生成论文摘要