Joint Variation Learning of Fusion and Difference Features for Change Detection in Remote Sensing Images
IEEE Transactions on Geoscience and Remote Sensing(2022)
摘要
Remote sensing (RS) image change detection (CD) is an Earth observation technique for detecting surface changes in the same area during a period. With the rapid development of deep learning, various deep neural networks especially Siamese ones have been widely used in the field of CD. However, they have the deficiency of insufficient contextual information aggregation, thus resulting in false and missed detections, and it is difficult to refine the detection of change edges. To alleviate these problems and obtain more accurate results, we propose an efficient self-weighted spatial-temporal attention network (SSANet). In contrast to the Siamese structure, our network is a novel joint learning framework composed of fusion subnetwork, difference subnetwork, and decoder. Fusion subnetwork is used to extract multiscale object features where we propose a multicore channel-aligning attention (MCA) module to capture the long-range semantic information for multiscale context aggregation. Difference subnetwork is used to extract the difference variation features, where we propose a feature differential reconfiguration (FDR) module to learn the temporal change information. FDR can effectively filter change information and reconstruct features to improve the perception of changed regions. To better balance the MCA and FDR modules, an asymmetric weighting (AW) module is proposed in the decoder to self-weight the multiscale features and generate the change map. Experiments demonstrate the efficiency of proposed subnetworks and modules, and the state-of-the-art performance of SSANet.
更多查看译文
关键词
Feature extraction,Task analysis,Neural networks,Data mining,Decoding,Semantics,Deep learning,Asymmetric weighting (AW) module,change detection (CD),deep learning,feature differential reconfiguration (FDR) module,multicore channel-aligning attention (MCA) module
AI 理解论文
溯源树
样例
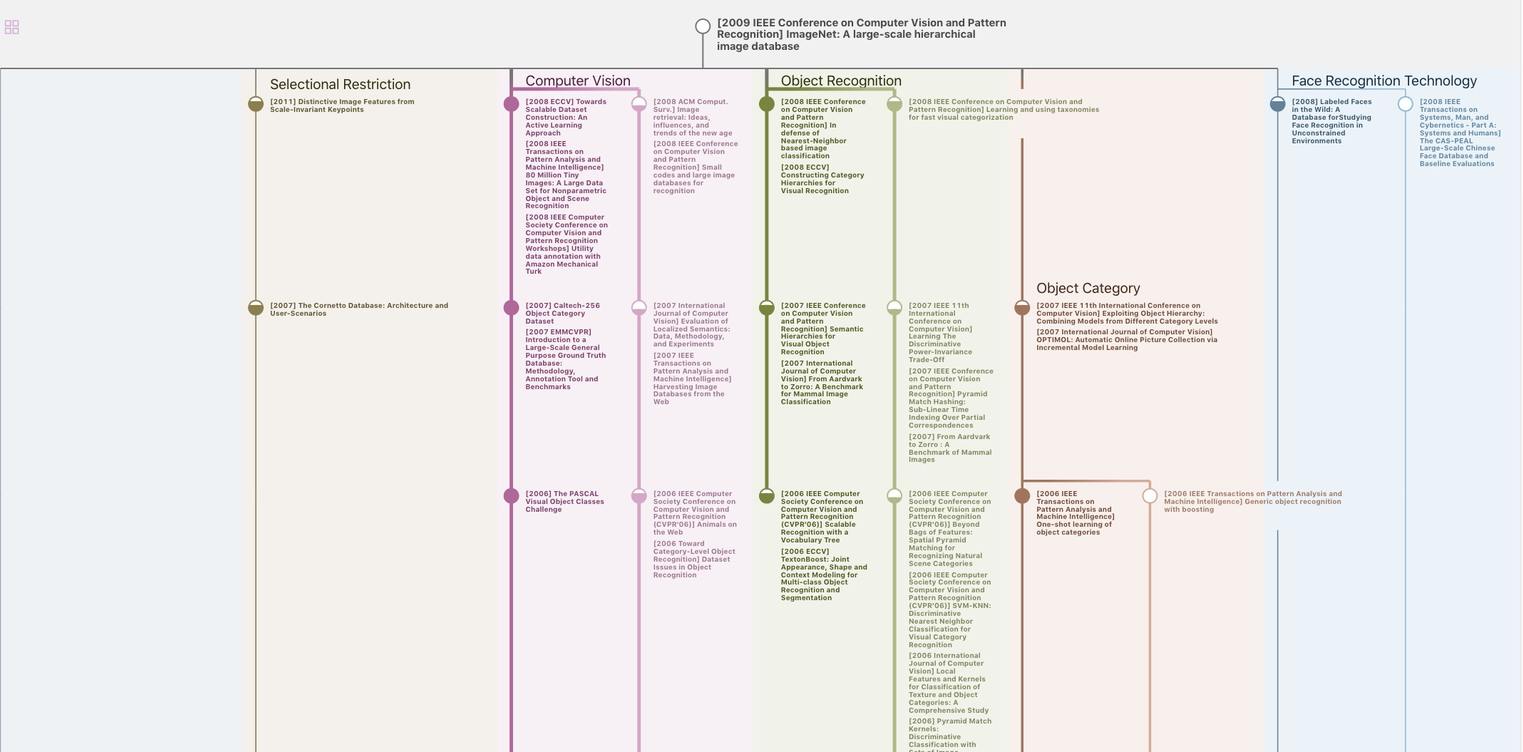
生成溯源树,研究论文发展脉络
Chat Paper
正在生成论文摘要