Energy Efficient Approximate 3D Image Reconstruction
IEEE Transactions on Emerging Topics in Computing(2022)
摘要
We demonstrate an efficient and accelerated parallel, sparse depth reconstruction framework using compressed sensing (compressed sensing (CS)) and approximate computing. Employing data parallelism for rapid image formation, the depth image is reconstructed from sparsely sampled scenes using convex optimization. Coupled with faster imaging, this sparse sampling reduces significantly the projected laser power in active systems such as light detection and ranging (LiDAR) to allow eye safe operation at longer range. We also demonstrate how reduced precision is leveraged to reduce the number of logic units in field-programmable gate array (FPGA) implementations for such sparse imaging systems. It enables significant reduction in logic units, memory requirements and power consumption by over 80% with minimal impact on the quality of reconstruction. To further accelerate processing, pre-computed, important components of the lower-upper (LU) decomposition and other linear algebraic computations are used to solve the convex optimization problems. Our methodology is demonstrated by the application of the alternating direction method of multipliers (ADMM) and proximal gradient descent (PGD) algorithms. For comparison, a fully discrete least square reconstruction method (
$d$
Sparse) is also presented. This demonstrates the feasibility of novel, high resolution, low power and high frame rate LiDAR depth imagers based on sparse illumination for use in applications where resources are strictly limited.
更多查看译文
关键词
Approximate computing,parallel computing,FPGA,LiDAR,depth reconstruction,compressed sensing,convex optimisation
AI 理解论文
溯源树
样例
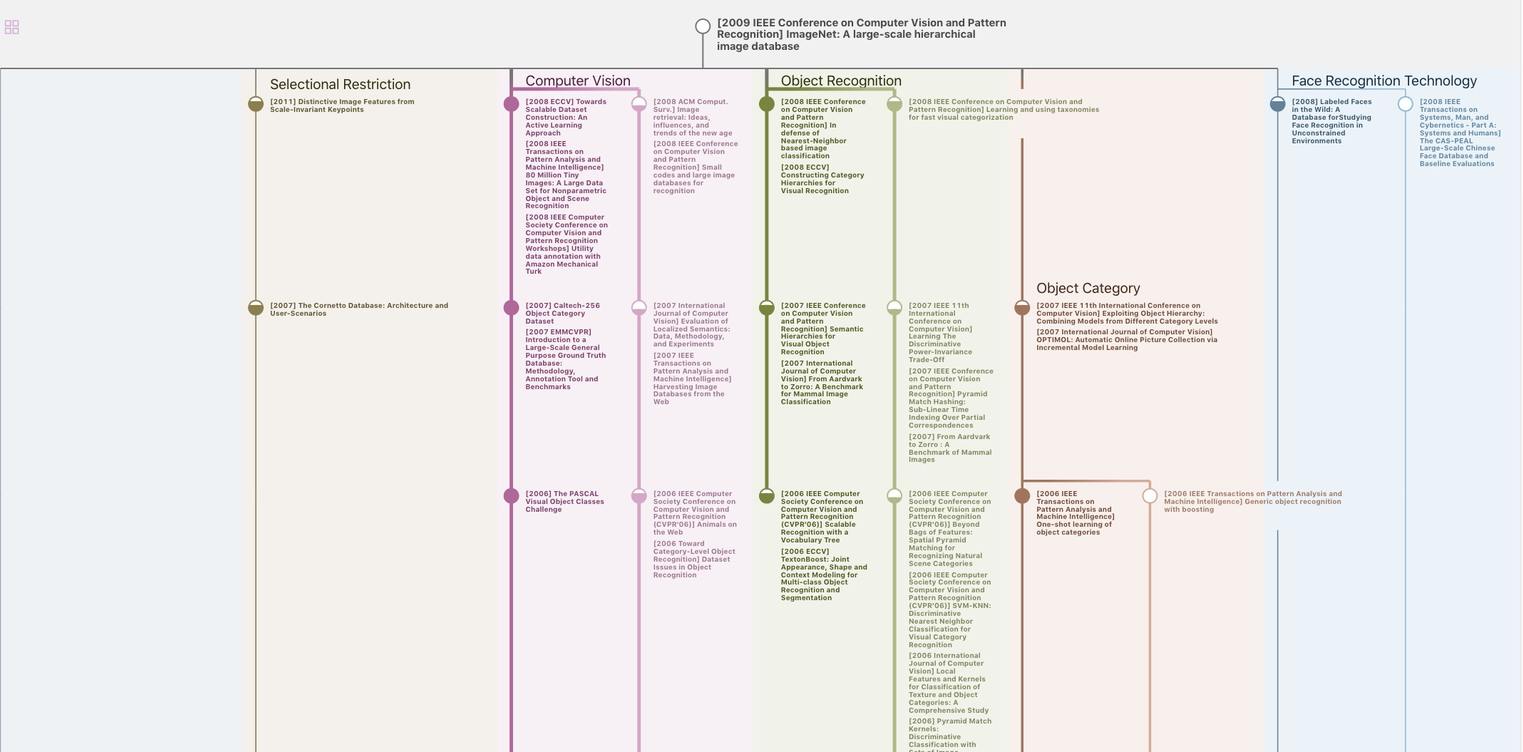
生成溯源树,研究论文发展脉络
Chat Paper
正在生成论文摘要