MicroCrack-Net: A Deep Neural Network With Outline Profile-Guided Feature Augmentation and Attention-Based Multiscale Fusion for MicroCrack Detection of Tantalum Capacitors
IEEE Transactions on Aerospace and Electronic Systems(2022)
摘要
The microcrack defect in the terminal electrode of the tantalum capacitor seriously affects the service life of the capacitor. However, the microcrack displays low contrast and large noises in the image. Meanwhile, the width of the microcrack is small, and the area in the image is tiny, which brings additional challenges to the detection of microcracks on the terminal electrodes. To improve the performance of detecting microcracks, this article proposes a detection model with outline profile-guided feature augmentation and attention-based multiscale fusion, titled with MicroCrack-Net. In this method, the microcrack outline is utilized to guide the lossless feature extraction to strengthen unobvious microcrack features without pooling operation to avoid information loss of tiny defects during the layer-by-layer processing in the convolutional neural network. A gradual attention mechanism is proposed to attract the attention of the detection model to local defect areas in the image with noises. Multiscale feature extraction and fusion are designed to process the data in tensors of the same size. The experimental results demonstrated that the proposed model is effective and has superior performance over VGG16, resNet50, resNext50, DenseNet, Racki-Net, and SegDecNet in detecting microcracks.
更多查看译文
关键词
Attention mechanism,feature augmentation,neural network,multiscale feature extraction and fusion
AI 理解论文
溯源树
样例
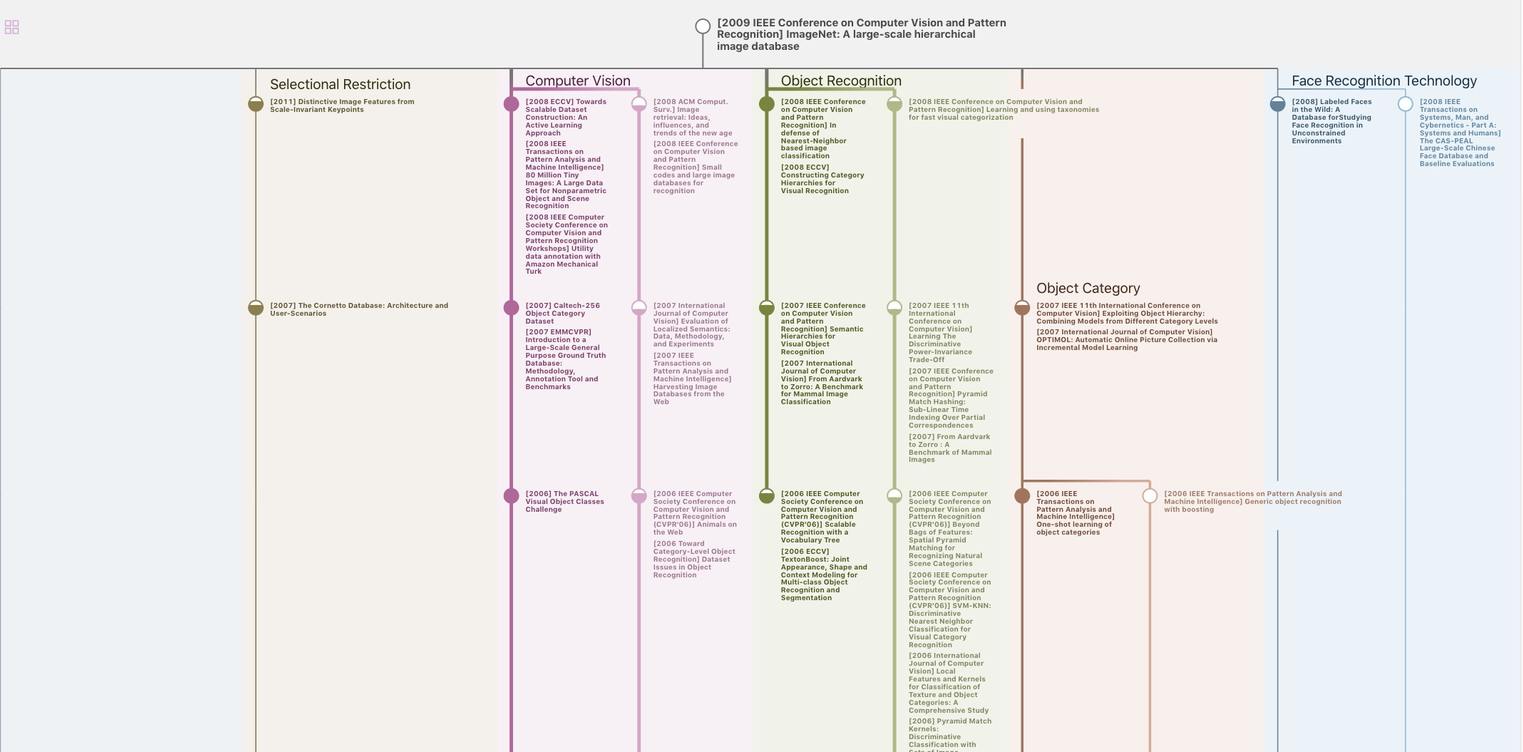
生成溯源树,研究论文发展脉络
Chat Paper
正在生成论文摘要