Pixel2ISDF: Implicit Signed Distance Fields based Human Body Model from Multi-view and Multi-pose Images
arxiv(2022)
摘要
In this report, we focus on reconstructing clothed humans in the canonical space given multiple views and poses of a human as the input. To achieve this, we utilize the geometric prior of the SMPLX model in the canonical space to learn the implicit representation for geometry reconstruction. Based on the observation that the topology between the posed mesh and the mesh in the canonical space are consistent, we propose to learn latent codes on the posed mesh by leveraging multiple input images and then assign the latent codes to the mesh in the canonical space. Specifically, we first leverage normal and geometry networks to extract the feature vector for each vertex on the SMPLX mesh. Normal maps are adopted for better generalization to unseen images compared to 2D images. Then, features for each vertex on the posed mesh from multiple images are integrated by MLPs. The integrated features acting as the latent code are anchored to the SMPLX mesh in the canonical space. Finally, latent code for each 3D point is extracted and utilized to calculate the SDF. Our work for reconstructing the human shape on canonical pose achieves 3rd performance on WCPA MVP-Human Body Challenge.
更多查看译文
关键词
human body,pixel2isdf,images,multi-view,multi-pose
AI 理解论文
溯源树
样例
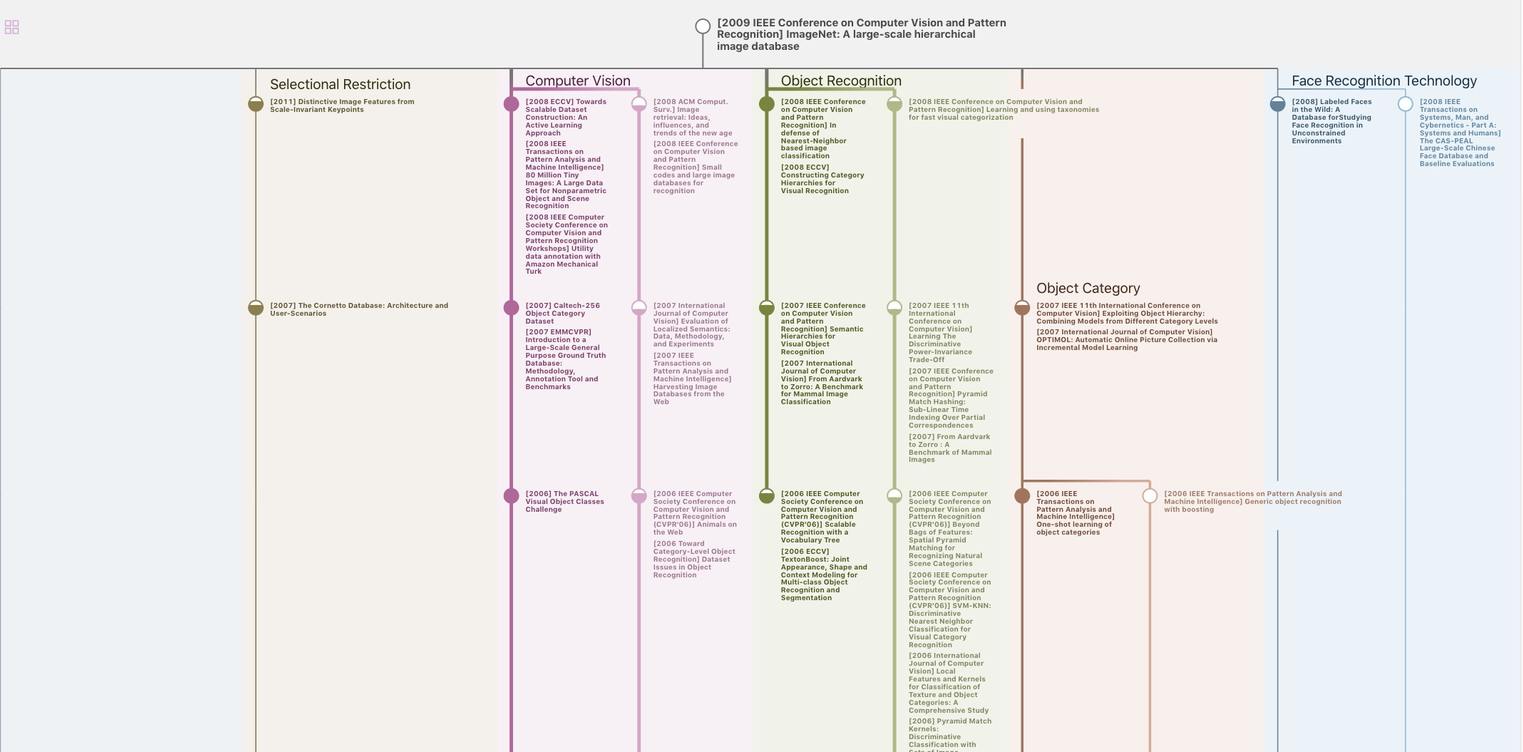
生成溯源树,研究论文发展脉络
Chat Paper
正在生成论文摘要