A 64-core mixed-signal in-memory compute chip based on phase-change memory for deep neural network inference
arxiv(2022)
摘要
The need to repeatedly shuttle around synaptic weight values from memory to processing units has been a key source of energy inefficiency associated with hardware implementation of artificial neural networks. Analog in-memory computing (AIMC) with spatially instantiated synaptic weights holds high promise to overcome this challenge, by performing matrix-vector multiplications (MVMs) directly within the network weights stored on a chip to execute an inference workload. However, to achieve end-to-end improvements in latency and energy consumption, AIMC must be combined with on-chip digital operations and communication to move towards configurations in which a full inference workload is realized entirely on-chip. Moreover, it is highly desirable to achieve high MVM and inference accuracy without application-wise re-tuning of the chip. Here, we present a multi-core AIMC chip designed and fabricated in 14-nm complementary metal-oxide-semiconductor (CMOS) technology with backend-integrated phase-change memory (PCM). The fully-integrated chip features 64 256x256 AIMC cores interconnected via an on-chip communication network. It also implements the digital activation functions and processing involved in ResNet convolutional neural networks and long short-term memory (LSTM) networks. We demonstrate near software-equivalent inference accuracy with ResNet and LSTM networks while implementing all the computations associated with the weight layers and the activation functions on-chip. The chip can achieve a maximal throughput of 63.1 TOPS at an energy efficiency of 9.76 TOPS/W for 8-bit input/output matrix-vector multiplications.
更多查看译文
关键词
Electrical and electronic engineering,Information technology,Electrical Engineering
AI 理解论文
溯源树
样例
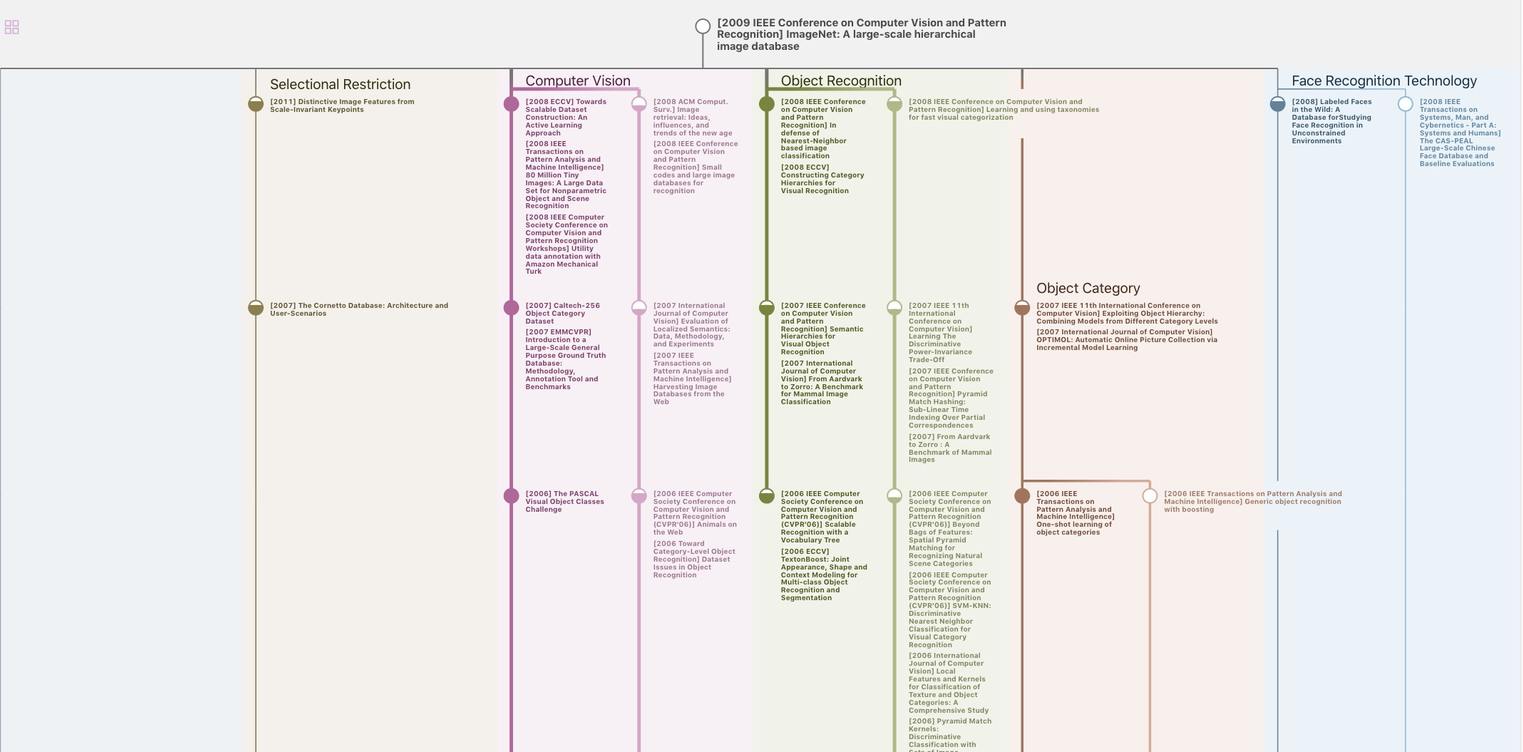
生成溯源树,研究论文发展脉络
Chat Paper
正在生成论文摘要