InternVideo: General Video Foundation Models via Generative and Discriminative Learning
arXiv (Cornell University)(2022)
摘要
The foundation models have recently shown excellent performance on a variety of downstream tasks in computer vision. However, most existing vision foundation models simply focus on image-level pretraining and adpation, which are limited for dynamic and complex video-level understanding tasks. To fill the gap, we present general video foundation models, InternVideo, by taking advantage of both generative and discriminative self-supervised video learning. Specifically, InternVideo efficiently explores masked video modeling and video-language contrastive learning as the pretraining objectives, and selectively coordinates video representations of these two complementary frameworks in a learnable manner to boost various video applications. Without bells and whistles, InternVideo achieves state-of-the-art performance on 39 video datasets from extensive tasks including video action recognition/detection, video-language alignment, and open-world video applications. Especially, our methods can obtain 91.1% and 77.2% top-1 accuracy on the challenging Kinetics-400 and Something-Something V2 benchmarks, respectively. All of these results effectively show the generality of our InternVideo for video understanding. The code will be released at https://github.com/OpenGVLab/InternVideo .
更多查看译文
关键词
general internvideo foundation models,discriminative learning,generative
AI 理解论文
溯源树
样例
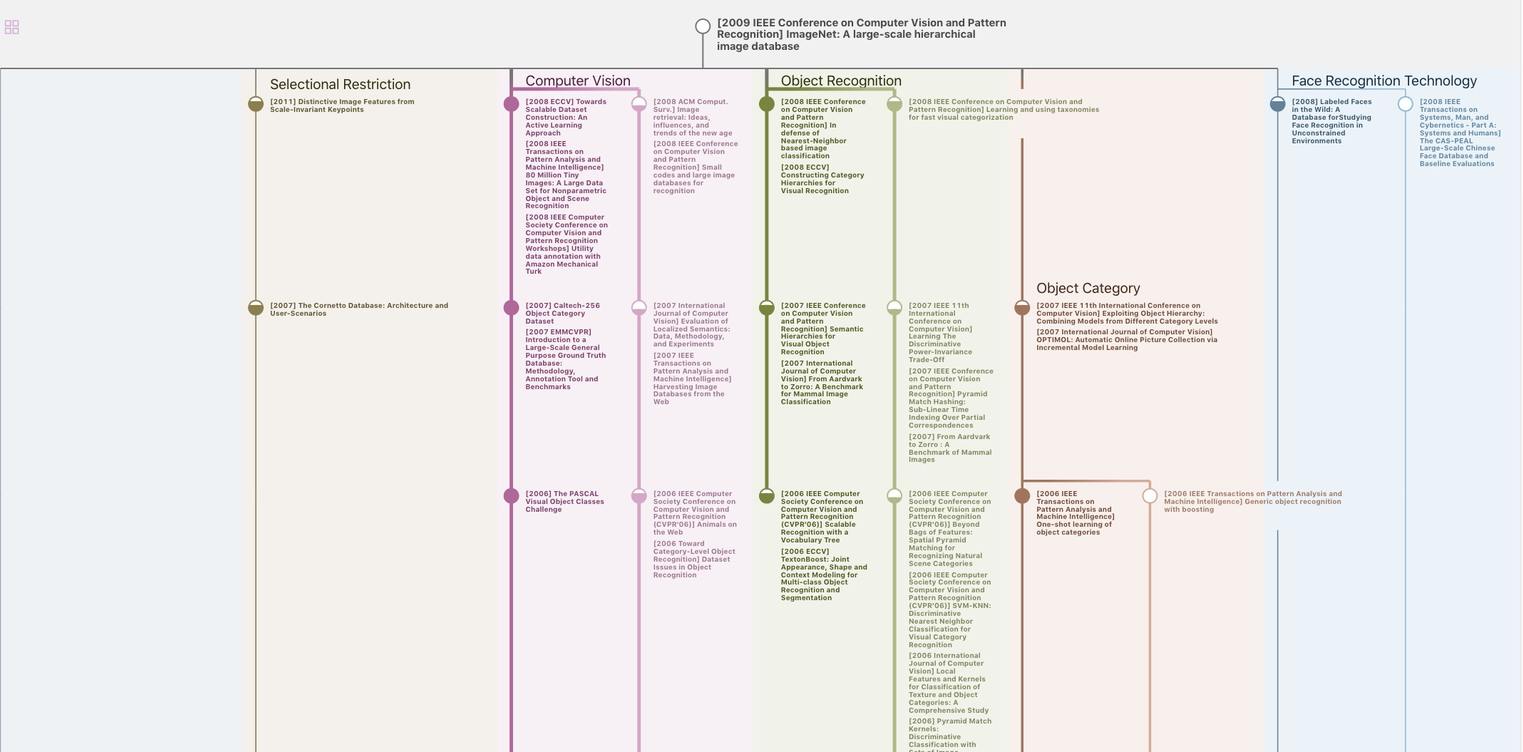
生成溯源树,研究论文发展脉络
Chat Paper
正在生成论文摘要