An industrially viable wavelet long-short term memory-deep multilayer perceptron-based approach to tool condition monitoring considering operational variability
PROCEEDINGS OF THE INSTITUTION OF MECHANICAL ENGINEERS PART E-JOURNAL OF PROCESS MECHANICAL ENGINEERING(2022)
摘要
Tool wear is a fundamental aspect of the machining process. Therefore, tool condition monitoring is of paramount importance to ensure part quality and avoid catastrophic damage. Tool wear has a direct correlation with the vibration emanating from the process; however, accurate prediction of tool wear indirectly from the vibration level is difficult because machining parameters such as cutting speed, depth of cut, and feed rate may vary continuously during an operation, depending on tool diameter, geometry, and material. These affect vibration levels as much as wear progress, which demands advanced intelligence that can adapt to variations in cutting conditions. This paper proposes a wavelet long-short term memory (WLSTM)-deep multilayer perceptron (DMLP)-based model, which utilizes the continuous wavelet transform for preprocessing of raw data, long-short term memory (LSTM) for extracting temporal information, and DMLP for regression of the tool wear. First, the model is evaluated by comparing it with other LSTM studies developed using the PHM 2010 dataset in the literature. Afterward, its industrial viability and adaptability performance to variations in cutting speed and tool diameter are assessed with several training scenarios. The results revealed auspicious performance in the proposed architecture's potential in predicting tool wear under operational variability.
更多查看译文
关键词
Tool condition monitoring,deep learning,wavelet long-short term memory,deep multilayer perceptron,continuous wavelet transform
AI 理解论文
溯源树
样例
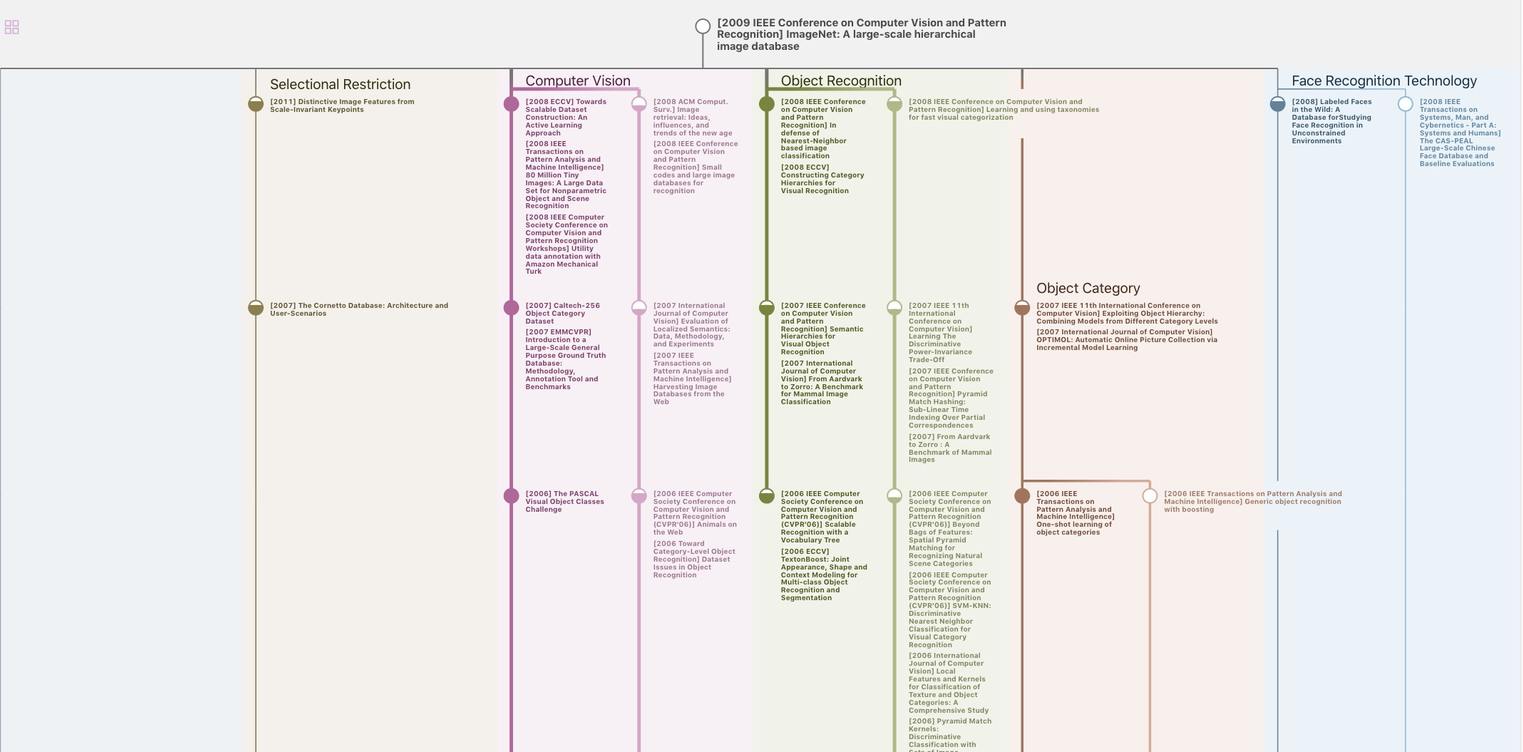
生成溯源树,研究论文发展脉络
Chat Paper
正在生成论文摘要