Text Embeddings by Weakly-Supervised Contrastive Pre-training
arxiv(2022)
摘要
This paper presents E5, a family of state-of-the-art text embeddings that transfer well to a wide range of tasks. The model is trained in a contrastive manner with weak supervision signals from our curated large-scale text pair dataset (called CCPairs). E5 can be readily used as a general-purpose embedding model for any tasks requiring a single-vector representation of texts such as retrieval, clustering, and classification, achieving strong performance in both zero-shot and fine-tuned settings. We conduct extensive evaluations on 56 datasets from the BEIR and MTEB benchmarks. For zero-shot settings, E5 is the first model that outperforms the strong BM25 baseline on the BEIR retrieval benchmark without using any labeled data. When fine-tuned, E5 obtains the best results on the MTEB benchmark, beating existing embedding models with 40x more parameters.
更多查看译文
关键词
text,weakly-supervised,pre-training
AI 理解论文
溯源树
样例
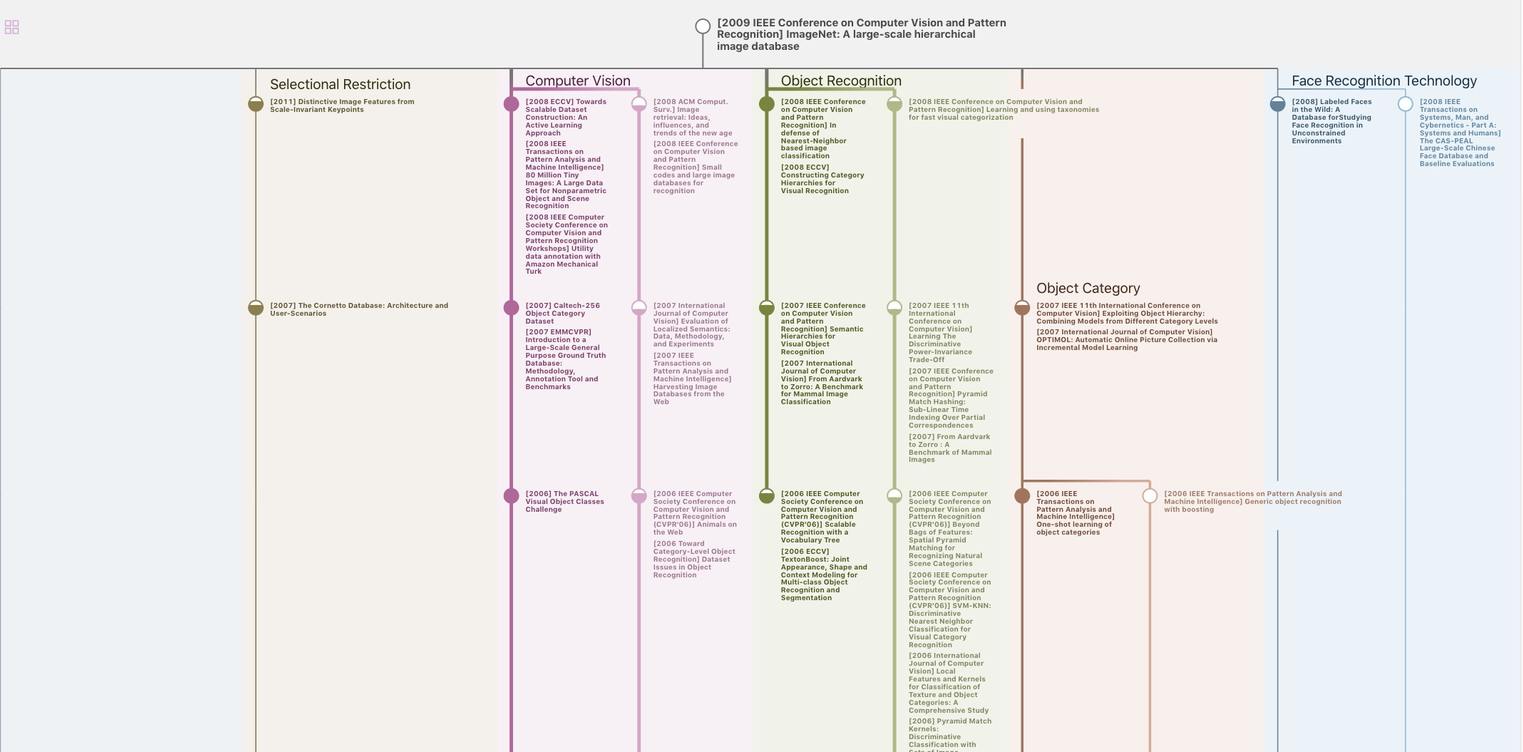
生成溯源树,研究论文发展脉络
Chat Paper
正在生成论文摘要