Haphazard Cuboids Feature Extraction for Micro-Expression Recognition.
IEEE Access(2022)
摘要
Facial micro-expressions can reveal a person's actual mental state and emotions. Therefore, it has crucial applications in many fields, such as lie detection, clinical medicine, and defense security. However, conventional methods have extracted features on designed facial regions to recognize micro-expressions, failing to effectively hit the micro-expression critical regions since micro-expressions are localized and asymmetric. Consequently, we propose the Haphazard Cuboids (HC) feature extraction method, which generates target regions by haphazard sampling technique and then extracts micro-expression spatio-temporal features. HC consists of two modules: spatial patches generation (SPG) and temporal segments generation (TSG). SPG is assigned to generate localized facial regions, and TSG is dedicated to generating temporal intervals. Through extensive experiments, we demonstrate the superiority of the proposed method. Afterward, we analyze two modules with conventional and deep-learning methods and find that they can significantly improve the performance of micro-expression recognition, respectively. Thereinto, we embed the SPG module into deep learning and experimentally demonstrate the effectiveness and superiority of our proposed sampling method in comparison with state-of-the-art methods. Furthermore, we analyze the TSG module with the maximum overlapping interval (MOI) method and find its coherence with the maximum interval of the apex frame distribution in CASME II and SAMM. Therefore, analogous to the human face's region of interest (ROI), micro-expressions also inherit similar ROI in the temporal dimension, whose positions are highly relevant to the intensive moment, i.e., the apex frame.
更多查看译文
关键词
Feature extraction,haphazard sampling,micro-expression recognition,ROI
AI 理解论文
溯源树
样例
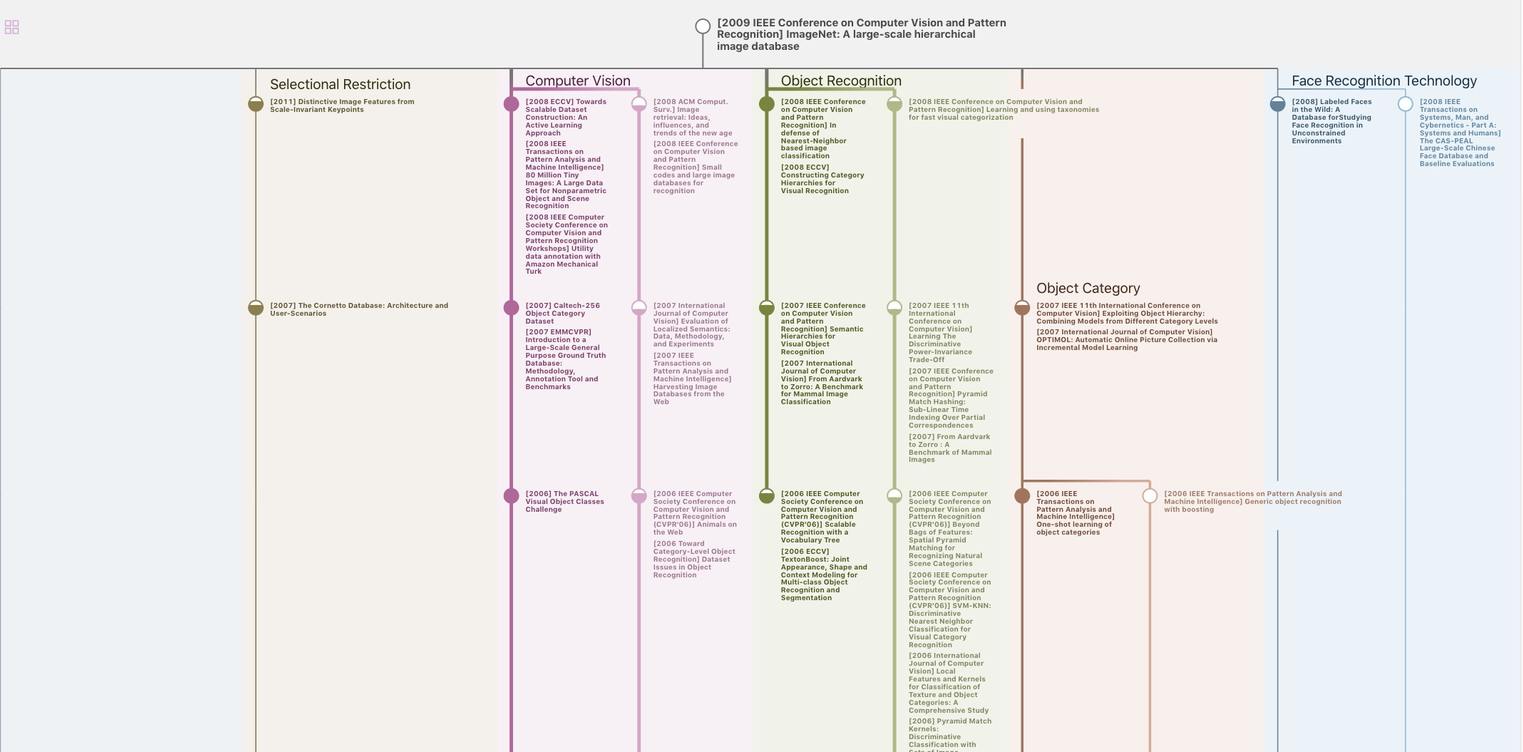
生成溯源树,研究论文发展脉络
Chat Paper
正在生成论文摘要