Revisiting Nearest-Neighbor-Based Information Set Decoding
CRYPTOGRAPHY AND CODING, IMACC 2023(2024)
摘要
The syndrome decoding problem lies at the heart of codebased cryptographic constructions. Information Set Decoding (ISD) algorithms are commonly used to assess the security of these systems. The most efficient ISD algorithms rely heavily on nearest neighbor search techniques. However, the runtime result of the fastest known ISD algorithm by Both-May (PQCrypto '18) was recently challenged by Carrier et al. (Asiacrypt '22), which introduce themselves a new technique called RLPN decoding which yields improvements over ISD for codes with small rates k/n <= 0.3. In this work we first revisit the Both-May algorithm, by giving a clean exposition and a corrected analysis. In this context we confirm the result by Carrier et al. that the initial analysis is flawed and conclude with the same runtime exponent. Our work aims at fully substantiating the corrected runtime exponent by a detailed analysis. Furthermore, we show that the Both-May algorithm significantly improves on memory complexity over previous algorithms. Our main contribution is therefore to give the correct perspective on the significance of the Both-May algorithm and to clarify any remaining doubts on the corrected baseline. Further, we outline a potential strategy for enhancing the Both-May algorithm by merging two of its subroutines, by introducing a fixed-weight nearest neighbor variant. Although we do not obtain immediate improvements, the nearest neighbor variant has already found novel applications in recent research. This initiated study of algorithms to solve the fixed-weight variant could potentially lead to future improvements through our construction.
更多查看译文
关键词
representation technique,syndrome decoding,nearest neighbor search,code-based cryptography
AI 理解论文
溯源树
样例
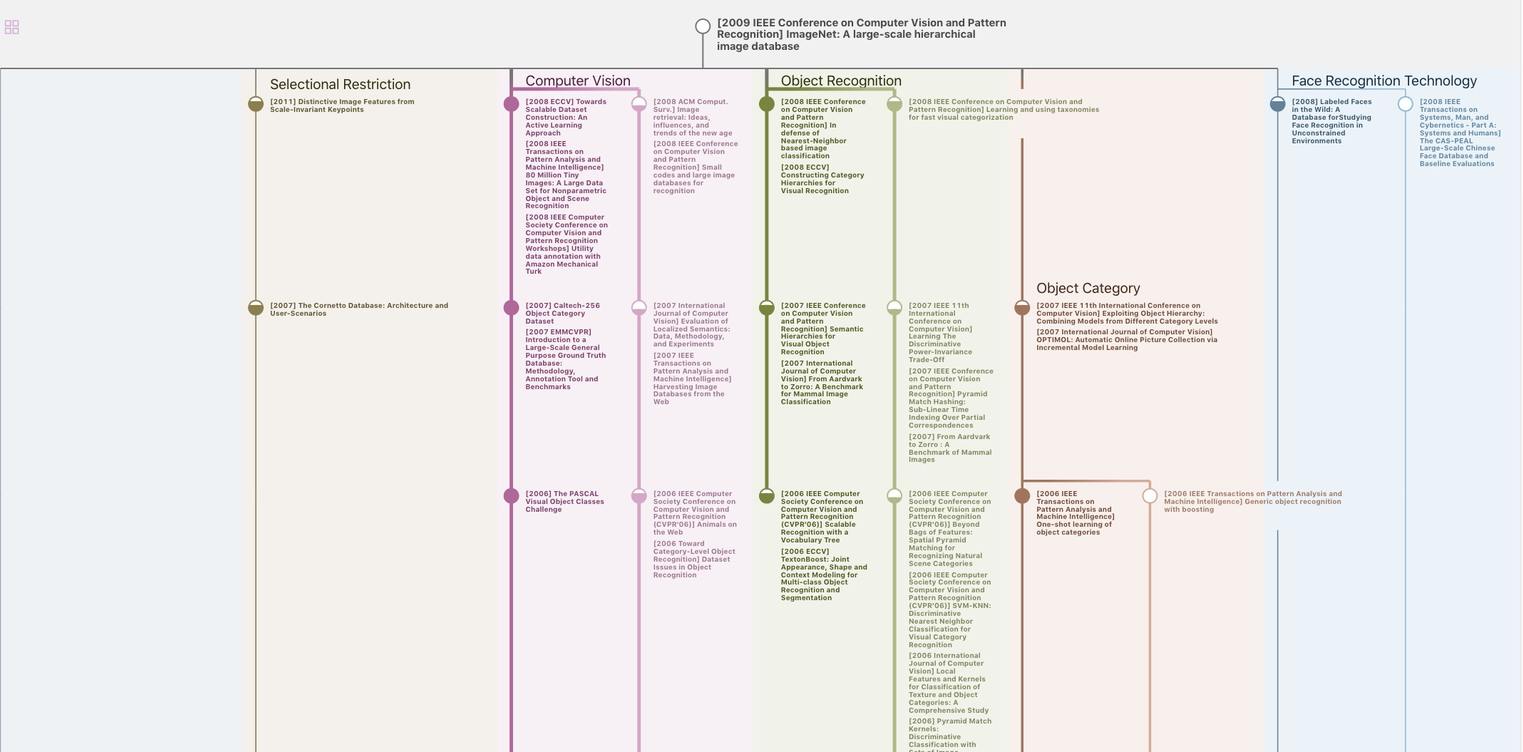
生成溯源树,研究论文发展脉络
Chat Paper
正在生成论文摘要