Transformer-Based Feature Compensation and Aggregation for DeepFake Detection.
IEEE Signal Processing Letters(2022)
摘要
Deepfake detection has attracted increasing attention in recent years. In this paper, we propose a transformer-based framework with feature compensation and aggregation (Trans-FCA) to extract rich forgery cues for deepfake detection. To compensate local features for transformers, we propose a Locality Compensation Block (LCB) containing a Global-Local Cross-Attention (GLCA) to attentively fuse global transformer features and local convolutional features. To aggregate features of all layers for capturing comprehensive and various fake flaws, we propose Multi-head Clustering Projection (MCP) and Frequency-guided Fusion Module (FFM), where the MCP attentively reduces redundant features into a few concentrated clusters, and the FFM interacts all clustered features under the guidance of frequency cues. In Trans-FCA, besides global cues captured by transformer architecture, local details and rich forgery defects are also captured using the proposed fetaure compensation and aggregation. Extensive experiments show our method outperforms the state-of-the-art methods on both intra-dataset and cross-dataset testings (with AUCs of 99.85% on FaceForensics++ and 78.57% on Celeb-DF), which clearly demonstrates the superiority of our Trans-FCA for deepfake detection.
更多查看译文
关键词
Feature extraction,Deepfakes,Current transformers,Frequency-domain analysis,Aggregates,Forgery,Standards,Face forgery detection,transformer,deep learning
AI 理解论文
溯源树
样例
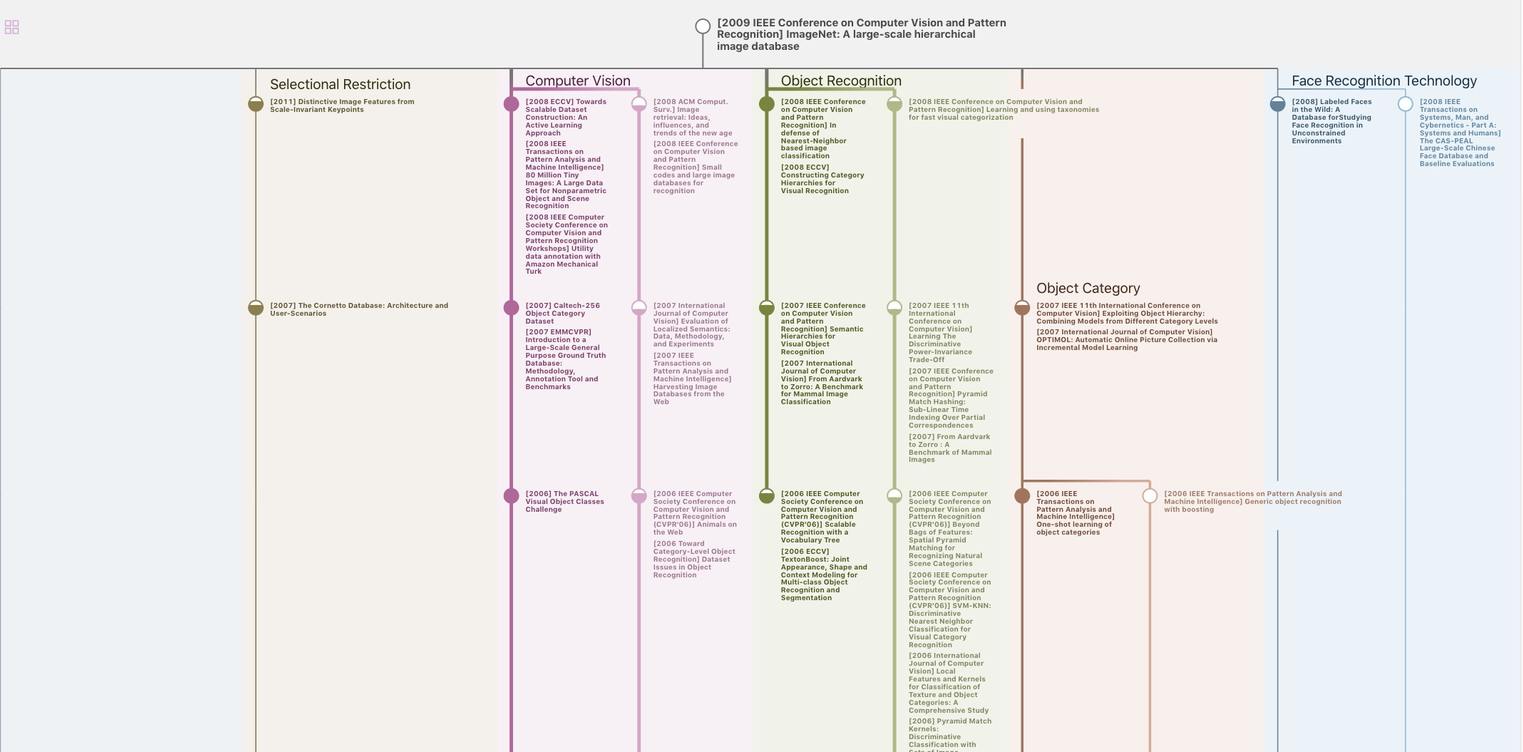
生成溯源树,研究论文发展脉络
Chat Paper
正在生成论文摘要