Uncertainty-aware Evaluation of Machine Learning Performance in binary Classification Tasks.
Journal of WSCG(2022)
摘要
Machine learning has become a standard tool in computer vision. Nowadays, neural networks are one of the most prominent representatives in this class of algorithms that usually require training and evaluation to work as desired. There exist a variety of evaluation metrics to determine the quality of a trained neural network, which are usually threshold dependent. This results in massive changes in the resulting evaluation when the threshold is changed slightly. Further, measurements of uncertainty such as resulting from Bayesian approaches, are not considered in this analysis. In this paper, we present evaluation metrics for machine learning approaches that are able to attach a probability distribution to the utilized threshold and include uncertainty measures. We demonstrate the applicability of our approach by applying the defined metrics to a real-world example where a Bayesian neural network has been used to predict stroke lesions.
更多查看译文
关键词
machine learning performance,machine learning,classification,uncertainty-aware
AI 理解论文
溯源树
样例
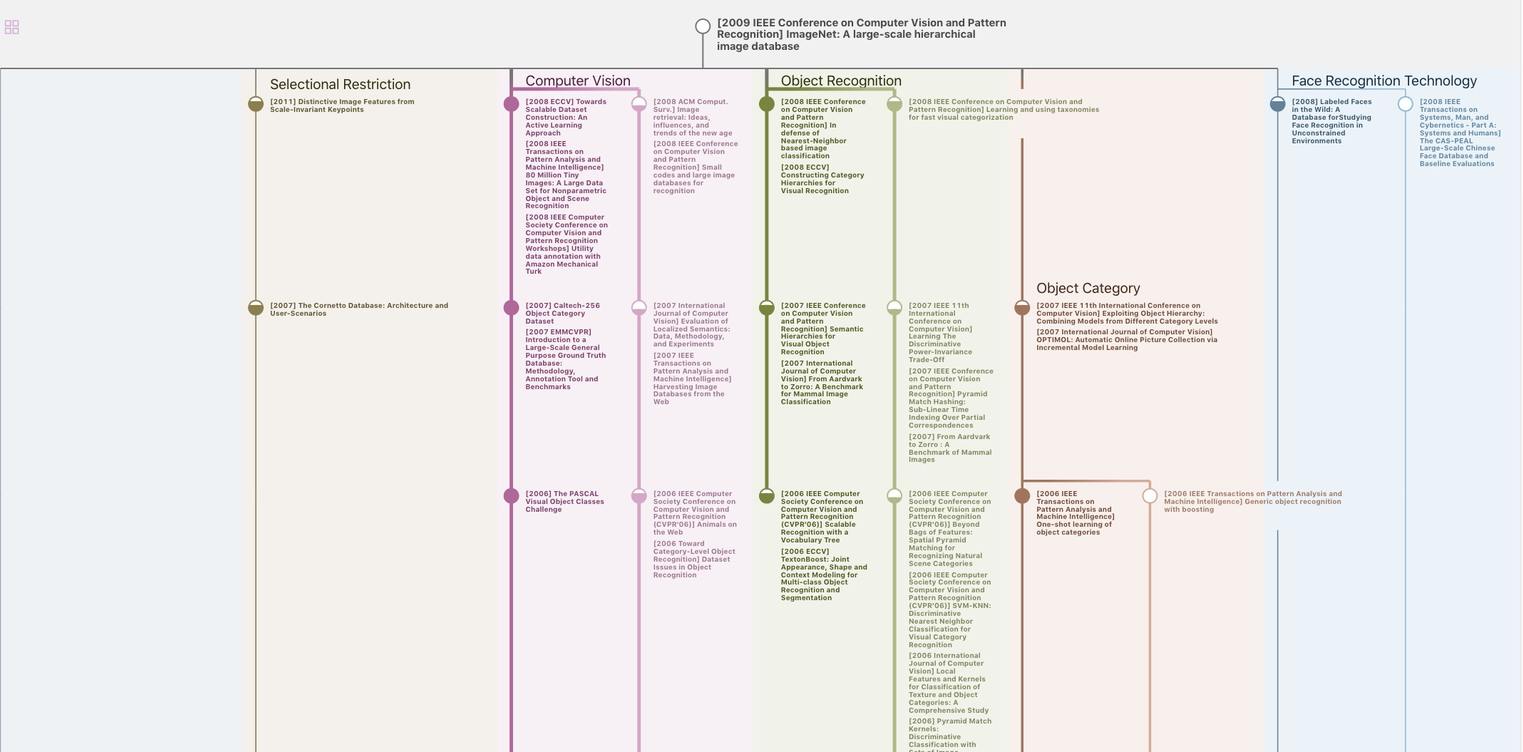
生成溯源树,研究论文发展脉络
Chat Paper
正在生成论文摘要