Effective Community Search over Large Star-Schema Heterogeneous Information Networks.
Proceedings of the VLDB Endowment(2022)
摘要
Community search (CS) enables personalized community discovery and has found a wide spectrum of emerging applications such as setting up social events and friend recommendation. While CS has been extensively studied for conventional homogeneous networks, the problem for heterogeneous information networks (HINs) has received attention only recently. However, existing studies suffer from several limitations, e.g., they either require users to specify a meta-path or relational constraints, which pose great challenges to users who are not familiar with HINs. To address these limitations, in this paper, we systematically study the problem of CS over large star-schema HINs without asking users to specify these constraints; that is, given a set.. of query vertices with the same type, find the most-likely community from a star-schema HIN containing Q, in which all the vertices are with the same type and close relationships. To capture the close relationships among vertices of the community, we employ the meta-path-based core model, and maximize the number of shared meta-paths such that each of them results in a cohesive core containing Q. To enable efficient CS, we first develop online algorithms via exploiting the anti-monotonicity property of shared meta-paths. We further boost the efficiency by proposing a novel index and an efficient index-based algorithm with elegant pruning techniques. Extensive experiments on four real large starschema HINs show that our solutions are effective and efficient for searching communities, and the index-based algorithm is much faster than the online algorithms.
更多查看译文
关键词
effective community search,star-schema
AI 理解论文
溯源树
样例
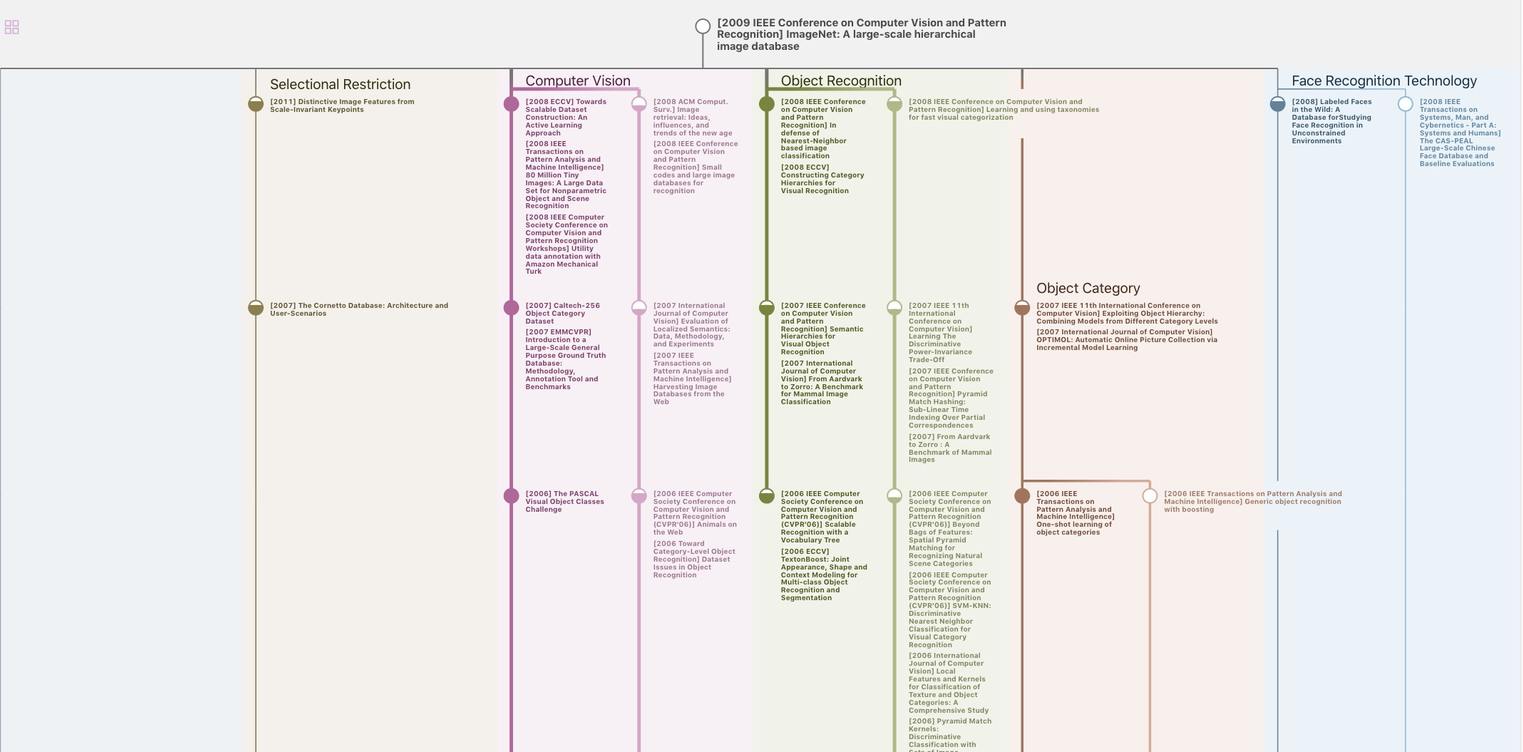
生成溯源树,研究论文发展脉络
Chat Paper
正在生成论文摘要