Short Time Series Forecasting: Recommended Methods and Techniques.
Symmetry(2022)
摘要
This paper tackles the problem of forecasting real-life crime. However, the recollected data only produced thirty-five short-sized crime time series for three urban areas. We present a comparative analysis of four simple and four machine-learning-based ensemble forecasting methods. Additionally, we propose five forecasting techniques that manage the seasonal component of the time series. Furthermore, we used the symmetric mean average percentage error and a Friedman test to compare the performance of the forecasting methods and proposed techniques. The results showed that simple moving average with seasonal removal techniques produce the best performance for these series. It is important to highlight that a high percentage of the time series has no auto-correlation and a high level of symmetry, which is deemed as white noise and, therefore, difficult to forecast.
更多查看译文
关键词
short-sized time-series, forecasting methods, seasonal extraction, forecasting techniques
AI 理解论文
溯源树
样例
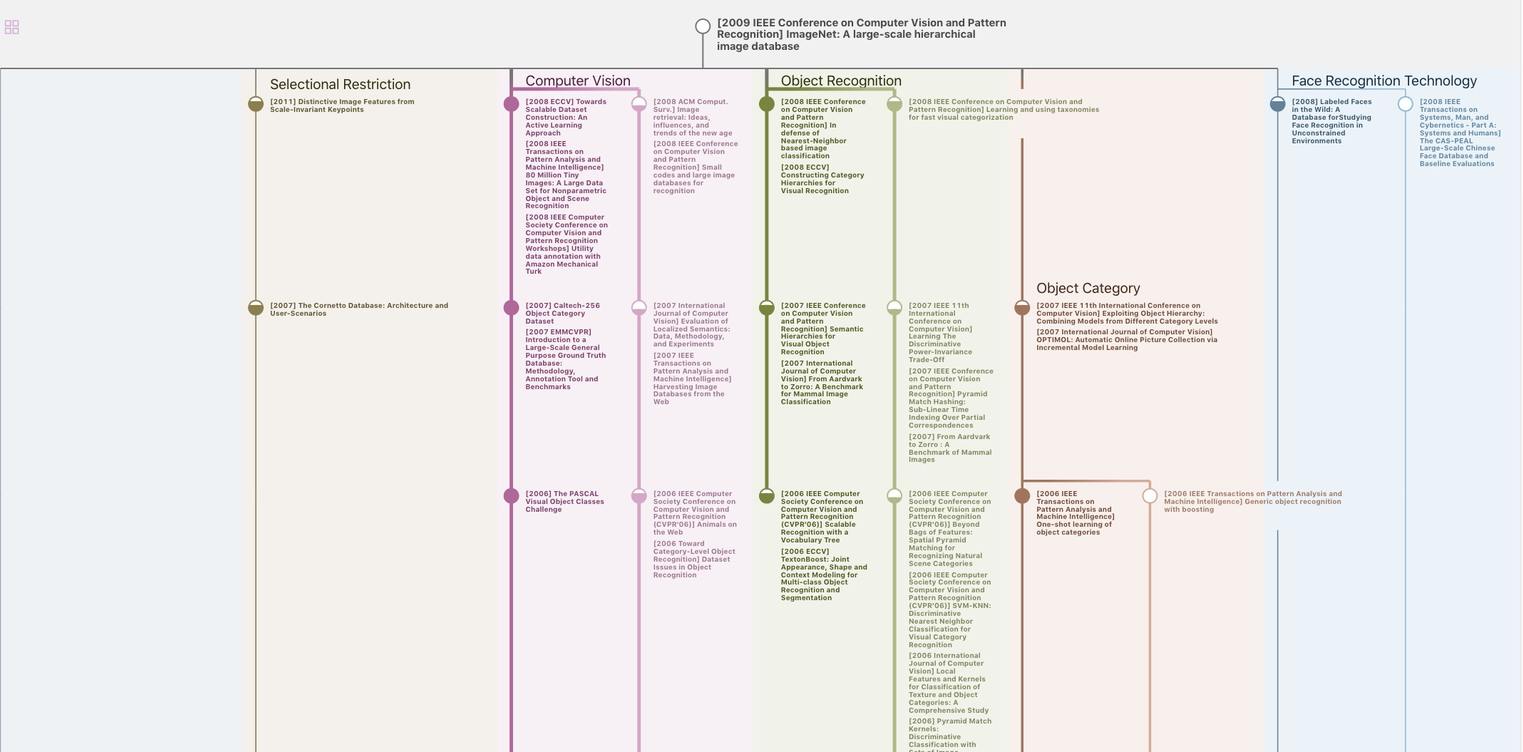
生成溯源树,研究论文发展脉络
Chat Paper
正在生成论文摘要