Physics-guided Data Augmentation for Learning the Solution Operator of Linear Differential Equations
arxiv(2022)
摘要
Neural networks, especially the recent proposed neural operator models, are increasingly being used to find the solution operator of differential equations. Compared to traditional numerical solvers, they are much faster and more efficient in practical applications. However, one critical issue is that training neural operator models require large amount of ground truth data, which usually comes from the slow numerical solvers. In this paper, we propose a physics-guided data augmentation (PGDA) method to improve the accuracy and generalization of neural operator models. Training data is augmented naturally through the physical properties of differential equations such as linearity and translation. We demonstrate the advantage of PGDA on a variety of linear differential equations, showing that PGDA can improve the sample complexity and is robust to distributional shift.
更多查看译文
关键词
data augmentation,solution operator,differential equations,learning,physics-guided
AI 理解论文
溯源树
样例
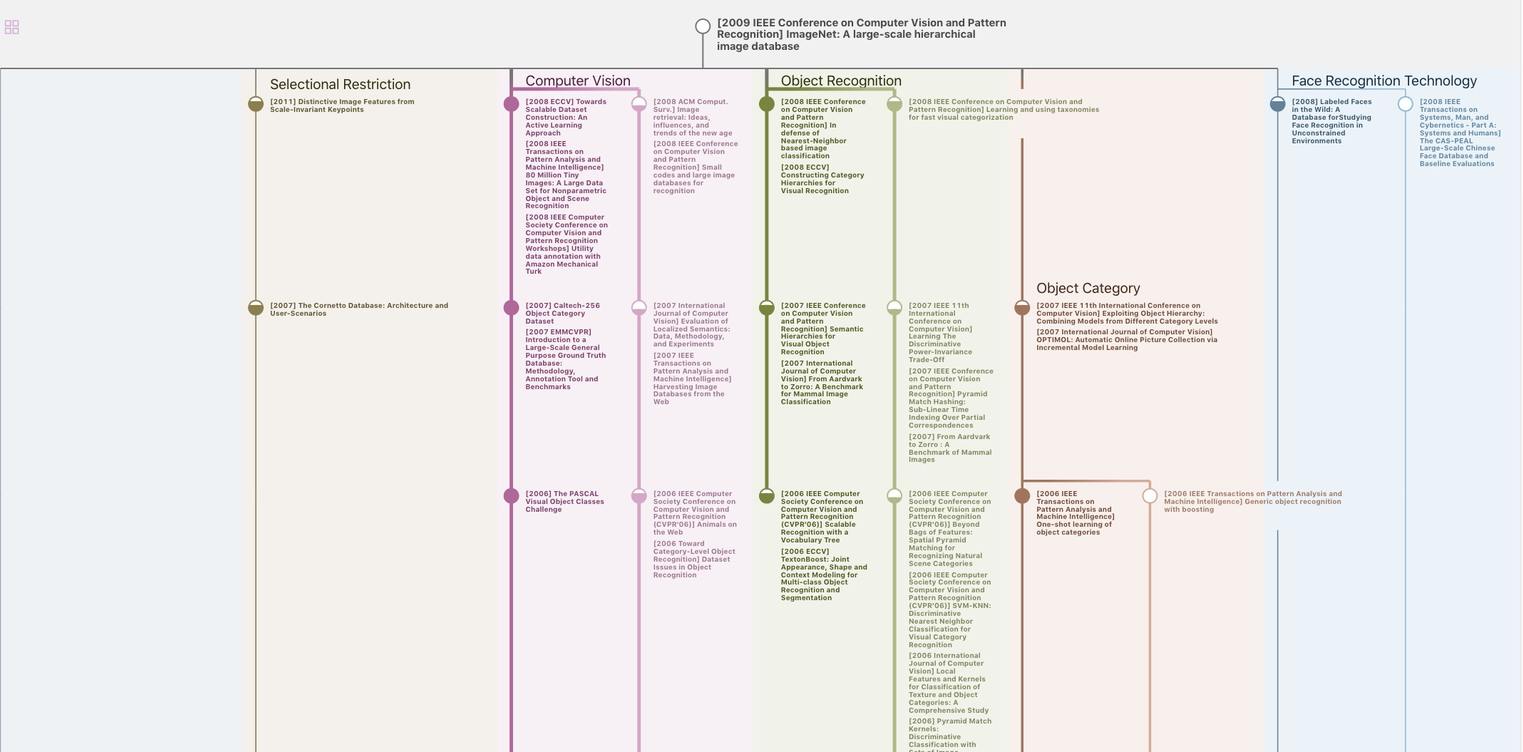
生成溯源树,研究论文发展脉络
Chat Paper
正在生成论文摘要