Uncertainty Minimization for Systems with Measurable Disturbance. Study case of Anesthesia-Hemodynamic Interactions*
2022 IEEE Conference on Control Technology and Applications (CCTA)(2022)
摘要
In this paper, a novel control methodology is presented to regulate the complex anesthetic-hemodynamic interaction during general anesthesia by means of predictive control algorithm. The proposed framework minimizes the risk of instability arising from large uncertainty in the patient model dynamics and external disturbances such as surgical events. Identification is very difficult and only a limited amount of data can be actually used during the startup of the anesthesia as to calibrate the generic patient models. There is a crucial need to explore additional ways to minimize uncertainty in the closed loop. The paper introduces a natural mimicking strategy of actual anesthesiologists real-life decision making procedures. The framework is tested in an open access patient simulator for hypnotic drug delivery management in general anesthesia.
更多查看译文
关键词
uncertainty,measurable disturbance,interactions,systems,anesthesia-hemodynamic
AI 理解论文
溯源树
样例
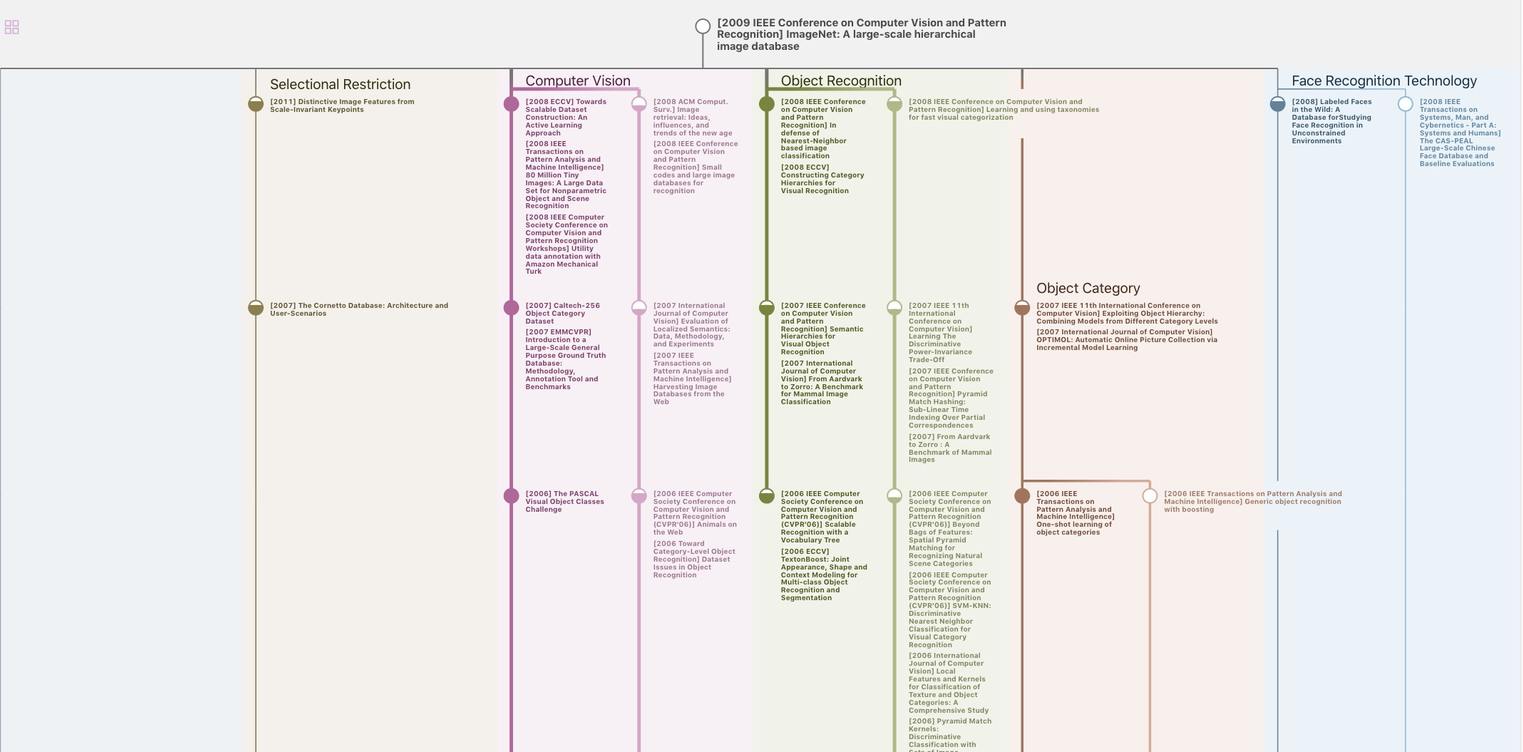
生成溯源树,研究论文发展脉络
Chat Paper
正在生成论文摘要