HANDOM: Heterogeneous Attention Network Model for Malicious Domain Detection
Computers & Security(2022)
摘要
Malicious domains are crucial vectors for attackers to conduct malicious activities. With the increasing numbers in domain-based attack activities and the enhancement of attacker evasion methods, the detection of malicious domains has become critical and increasingly difficult. Statistical feature-based and graph structure-based detection methods are mainstream technical approaches. However, highly hidden domains can escape feature detection, and the detection range of graph structure-based methods is limited. Based on these, we propose a malicious detection method called HANDOM. HANDOM combines statistical features and graph structural information to neutralize their limitations, and uses the Heterogeneous Attention Network (HAN) model to jointly handle both information to achieve high-performance malicious domain classification. We conduct experimental evaluations on real-world datasets and compare HANDOM with machine learning methods and other malicious detection methods. The results present that HANDOM has superior and robust performance, and can identify highly hidden domains.
更多查看译文
关键词
Malware domain detection,Spatial-Temporal contextual correlation,Heterogeneous attention network,Statistical-and-Structural information
AI 理解论文
溯源树
样例
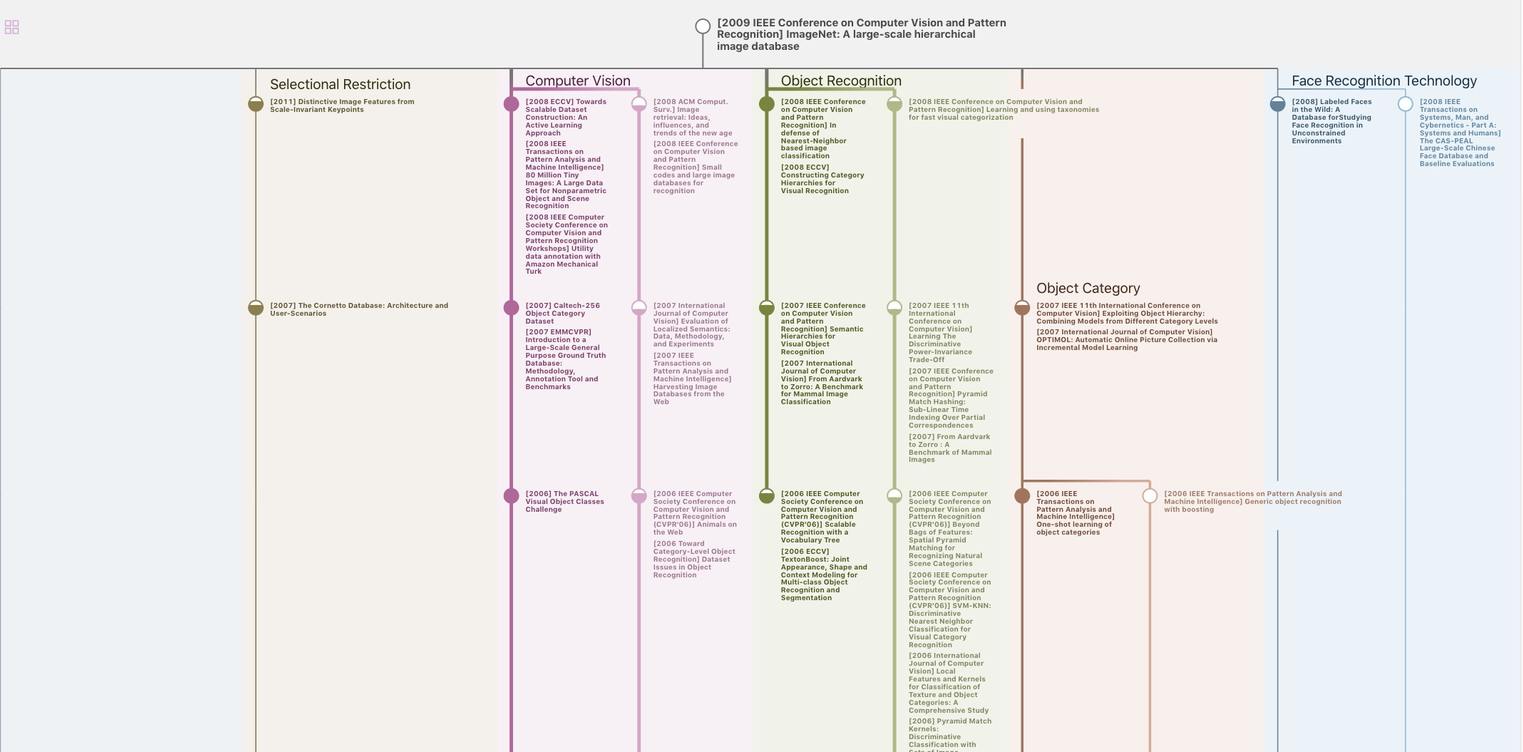
生成溯源树,研究论文发展脉络
Chat Paper
正在生成论文摘要