General stain deconvolution of histopathology images with physics-guided deep learning
biorxiv(2022)
摘要
Advances have been made in the use of deep learning to extract quantitative and predictive information from digital pathology slides, yet many barriers remain before clinical translation and deployment. In particular, models need to be generalizable despite the wide variations in image characteristics due to inter-scanner variability and differences in slide preparation protocols. This has led to an interest in stain deconvolution methods that could correct for the variability in image appearances. However, most existing stain deconvolution methods were developed and validated on specific datasets and perform poorly on unseen data. We developed Physics-Guided Deep Image Prior network for Stain deconvolution (PGDIPS), a method that combines a novel optical physics model and a self-supervised deep neural network to perform deconvolution for various classes and any number of stains, without the need of training data. PGDIPS outperformed state-of-the-art approaches for the deconvolution of conventional stain combinations, enabled analysis of previously unsupported special stains, and provided superior interpretability by explicitly encoding representations for stain properties and the light transmittance/absorbance process. PGDIPS is publicly available as an end-to-end off-the-shelf tool that does not require data curation, domain knowledge or high computation power.
### Competing Interest Statement
The authors have declared no competing interest.
更多查看译文
关键词
general stain deconvolution,histopathology images,deep learning,physics-guided
AI 理解论文
溯源树
样例
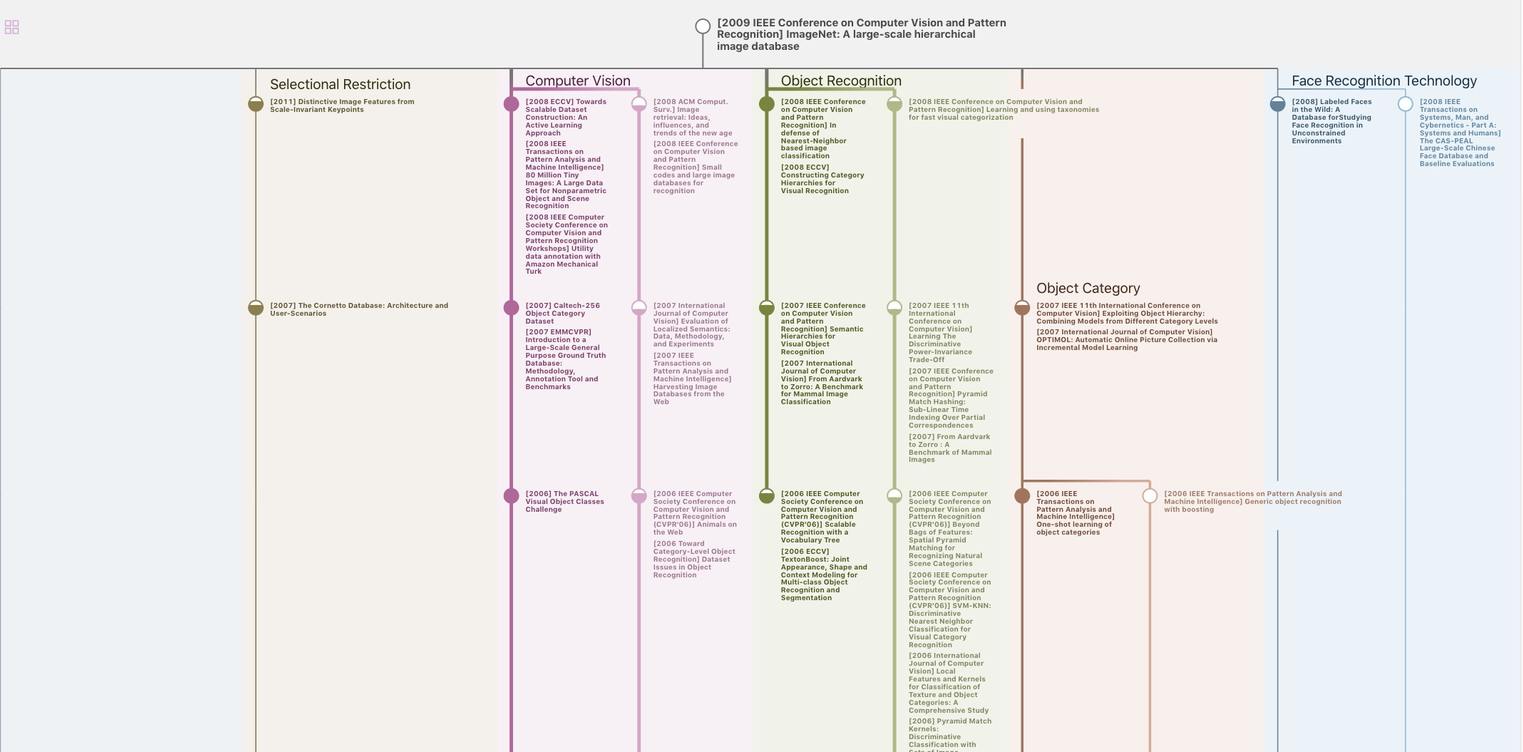
生成溯源树,研究论文发展脉络
Chat Paper
正在生成论文摘要