Multi-adversarial Faster-RCNN with Paradigm Teacher for Unrestricted Object Detection
INTERNATIONAL JOURNAL OF COMPUTER VISION(2022)
摘要
Recently, the cross-domain object detection task has been raised by reducing the domain disparity and learning domain invariant features. Inspired by the image-level discrepancy dominated in object detection, we introduce a M ulti- A dversarial F aster-RCNN (MAF). Our proposed MAF has two distinct contributions: (1) The Hierarchical Domain Feature Alignment (HDFA) module is introduced to minimize the image-level domain disparity, where Scale Reduction Module (SRM) reduces the feature map size without information loss and increases the training efficiency. (2) Aggregated Proposal Feature Alignment (APFA) module integrates the proposal feature and the detection results to enhance the semantic alignment, in which a weighted GRL (WGRL) layer highlights the hard-confused features rather than the easily-confused features. However, MAF only considers the domain disparity and neglects domain adaptability. As a result, the label-agnostic and inaccurate target distribution leads to the source error collapse, which is harmful to domain adaptation. Therefore, we further propose a Paradigm Teacher (PT) with knowledge distillation and formulated an extensive P aradigm T eacher MAF (PT-MAF), which has two new contributions: (1) The Paradigm Teacher (PT) overcomes source error collapse to improve the adaptability of the model. (2) The Dual-Discriminator HDFA (D ^2 -HDFA) improves the marginal distribution and achieves better alignment compared to HDFA. Extensive experiments on numerous benchmark datasets, including the Cityscapes, Foggy Cityscapes, Pascal VOC, Clipart, Watercolor, etc. demonstrate the superiority of our approach over SOTA methods.
更多查看译文
关键词
Object detection,Transfer learning,Domain adaptation,CNN
AI 理解论文
溯源树
样例
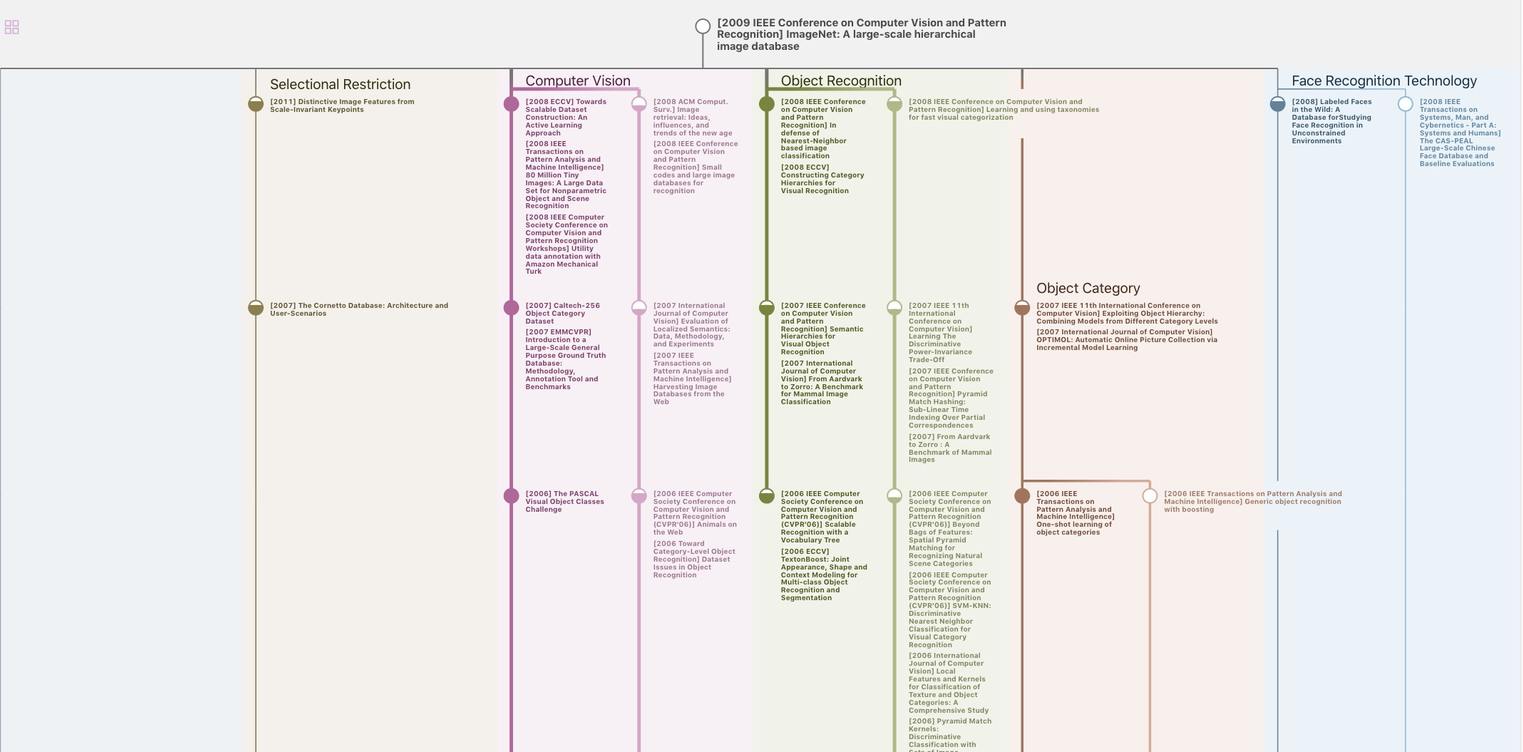
生成溯源树,研究论文发展脉络
Chat Paper
正在生成论文摘要