EPRI sparse reconstruction method based on deep learning
Magnetic Resonance Imaging(2023)
摘要
Electron paramagnetic resonance imaging (EPRI) is an advanced tumor oxygen concentration imaging method. Now, the bottleneck problem of EPRI is that the scanning time is too long. Sparse reconstruction is an effective and fast imaging method, which means reconstructing images from sparse-view projections. However, the EPRI images sparsely reconstructed by the classic filtered back projection (FBP) algorithm often contain severe streak artifacts, which affect subsequent image processing. In this work, we propose a feature pyramid attention-based, residual, dense, deep convolutional network (FRD-Net) to suppress the streak artifacts in the FBP-reconstructed images. This network combines residual connection, attention mechanism, dense connections and introduces perceptual loss. The EPRI image with streak artifacts is used as the input of the network and the output-label is the corresponding high-quality image densely reconstructed by the FBP algorithm. After training, the FRD-Net gets the capability of suppressing streak artifacts. The real data reconstruction experiments show that the FRD-Net can better improve the sparse reconstruction accuracy, compared with three existing representative deep networks.
更多查看译文
关键词
EPRI,Sparse reconstruction,Streak artifacts,Deep learning,Convolutional neural network
AI 理解论文
溯源树
样例
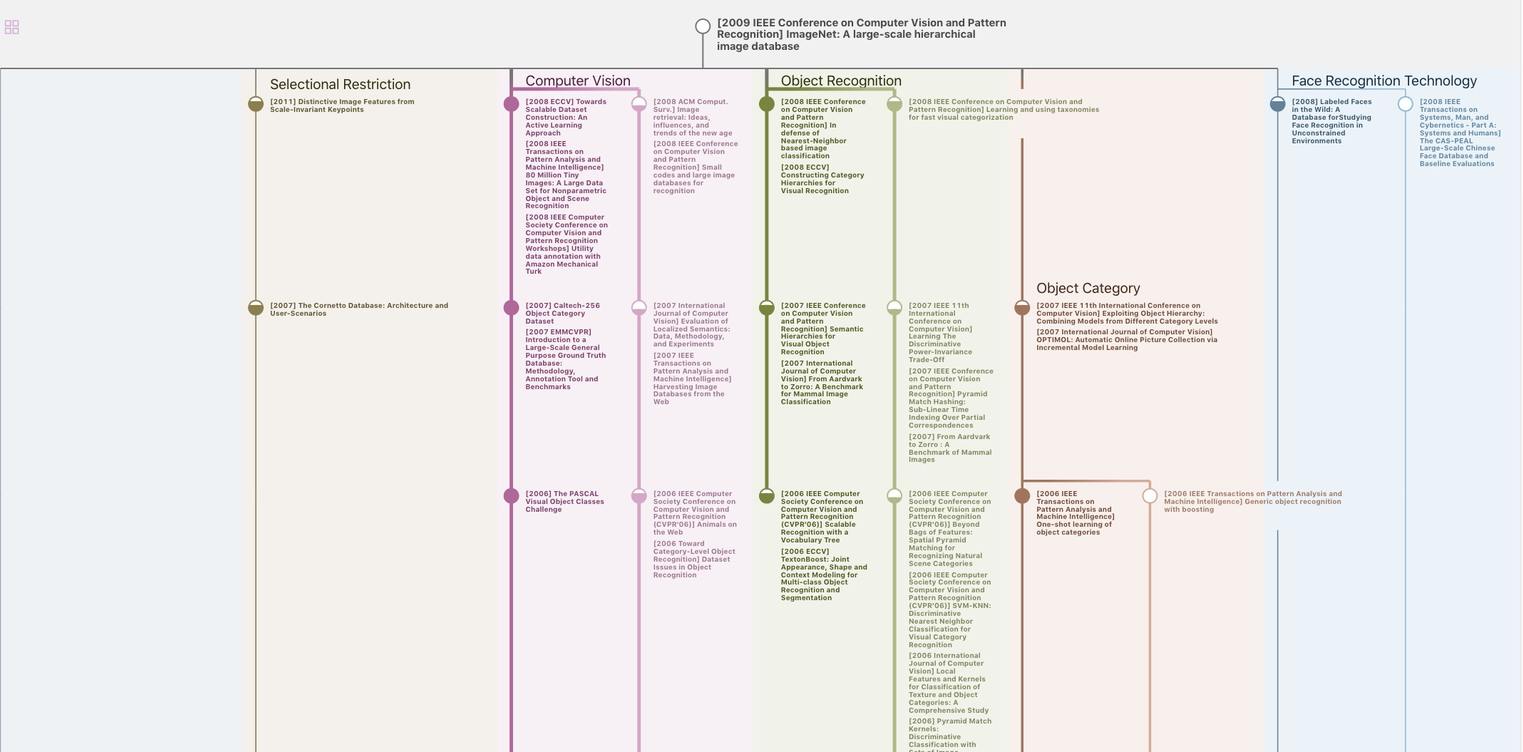
生成溯源树,研究论文发展脉络
Chat Paper
正在生成论文摘要