Noise2Contrast: Multi-Contrast Fusion Enables Self-Supervised Tomographic Image Denoising.
CoRR(2022)
摘要
Self-supervised image denoising techniques emerged as convenient methods that allow training denoising models without requiring ground-truth noise-free data. Existing methods usually optimize loss metrics that are calculated from multiple noisy realizations of similar images, e.g., from neighboring tomographic slices. However, those approaches fail to utilize the multiple contrasts that are routinely acquired in medical imaging modalities like MRI or dual-energy CT. In this work, we propose the new self-supervised training scheme Noise2Contrast that combines information from multiple measured image contrasts to train a denoising model. We stack denoising with domain-transfer operators to utilize the independent noise realizations of different image contrasts to derive a self-supervised loss. The trained denoising operator achieves convincing quantitative and qualitative results, outperforming state-of-the-art self-supervised methods by 4.7-11.0%/4.8-7.3% (PSNR/SSIM) on brainMRI data and by 43.6-50.5%/57.1-77.1% (PSNR/SSIM) on dual-energy CT X-raymicroscopy data with respect to the noisy baseline. Our experiments on different real measured data sets indicate that Noise2Contrast training generalizes to other multi-contrast imaging modalities.
更多查看译文
AI 理解论文
溯源树
样例
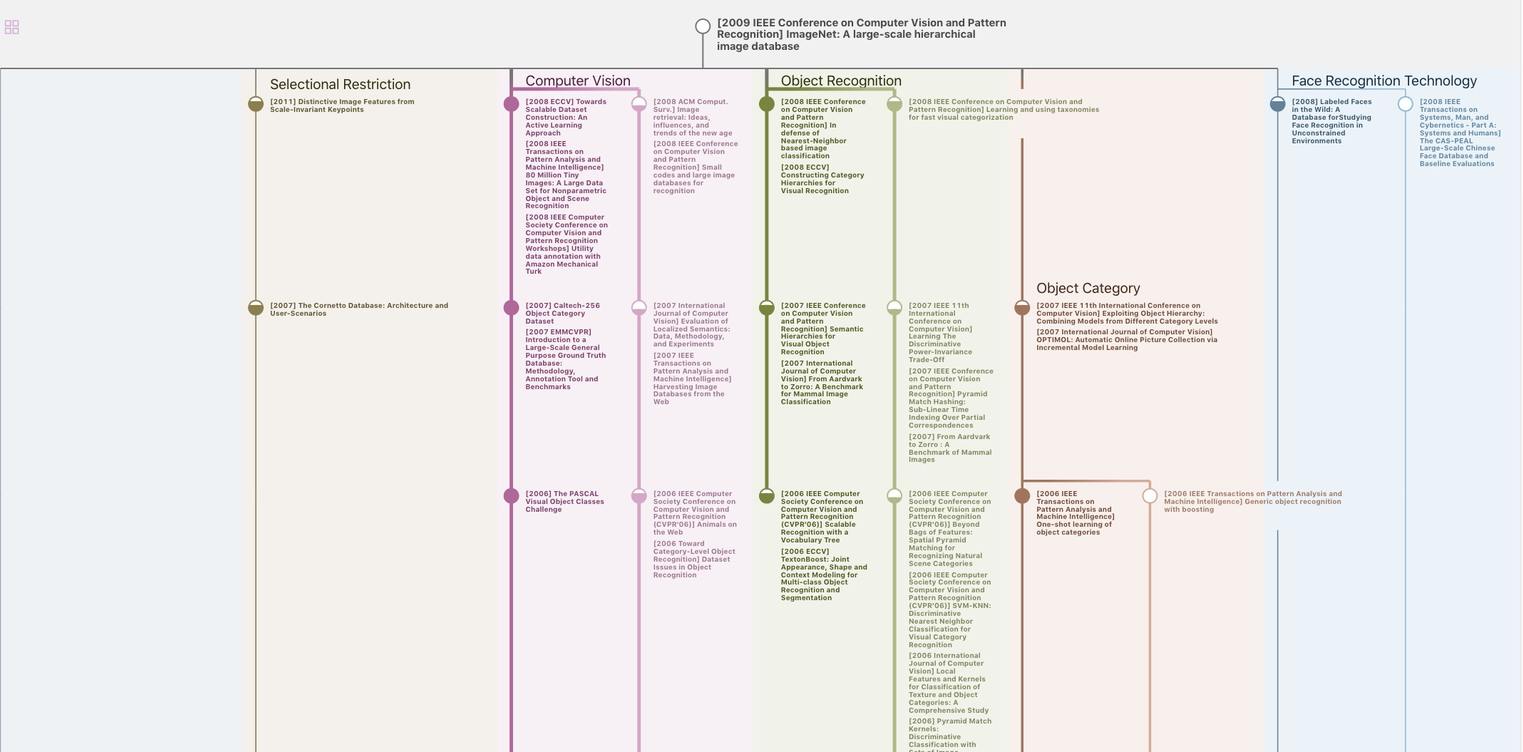
生成溯源树,研究论文发展脉络
Chat Paper
正在生成论文摘要