Lymphocyte Classification from Hoechst Stained Slides with Deep Learning.
Cancers(2022)
摘要
Multiplex immunofluorescence and immunohistochemistry benefit patients by allowing cancer pathologists to identify proteins expressed on the surface of cells. This enables cell classification, better understanding of the tumour microenvironment, and more accurate diagnoses, prognoses, and tailored immunotherapy based on the immune status of individual patients. However, these techniques are expensive. They are time consuming processes which require complex staining and imaging techniques by expert technicians. Hoechst staining is far cheaper and easier to perform, but is not typically used as it binds to DNA rather than to the proteins targeted by immunofluorescence techniques. In this work we show that through the use of deep learning it is possible to identify an immune cell subtype without immunofluorescence. We train a deep convolutional neural network to identify cells expressing the T lymphocyte marker CD3 from Hoechst 33342 stained tissue only. CD3 expressing cells are often used in key prognostic metrics such as assessment of immune cell infiltration, and by identifying them without the need for costly immunofluorescence, we present a promising new approach to cheaper prediction and improvement of patient outcomes. We also show that by using deep learning interpretability techniques, we can gain insight into the previously unknown morphological features which make this possible.
更多查看译文
关键词
computer vision,deep learning,image classification,imaging,lymphocyte subsets
AI 理解论文
溯源树
样例
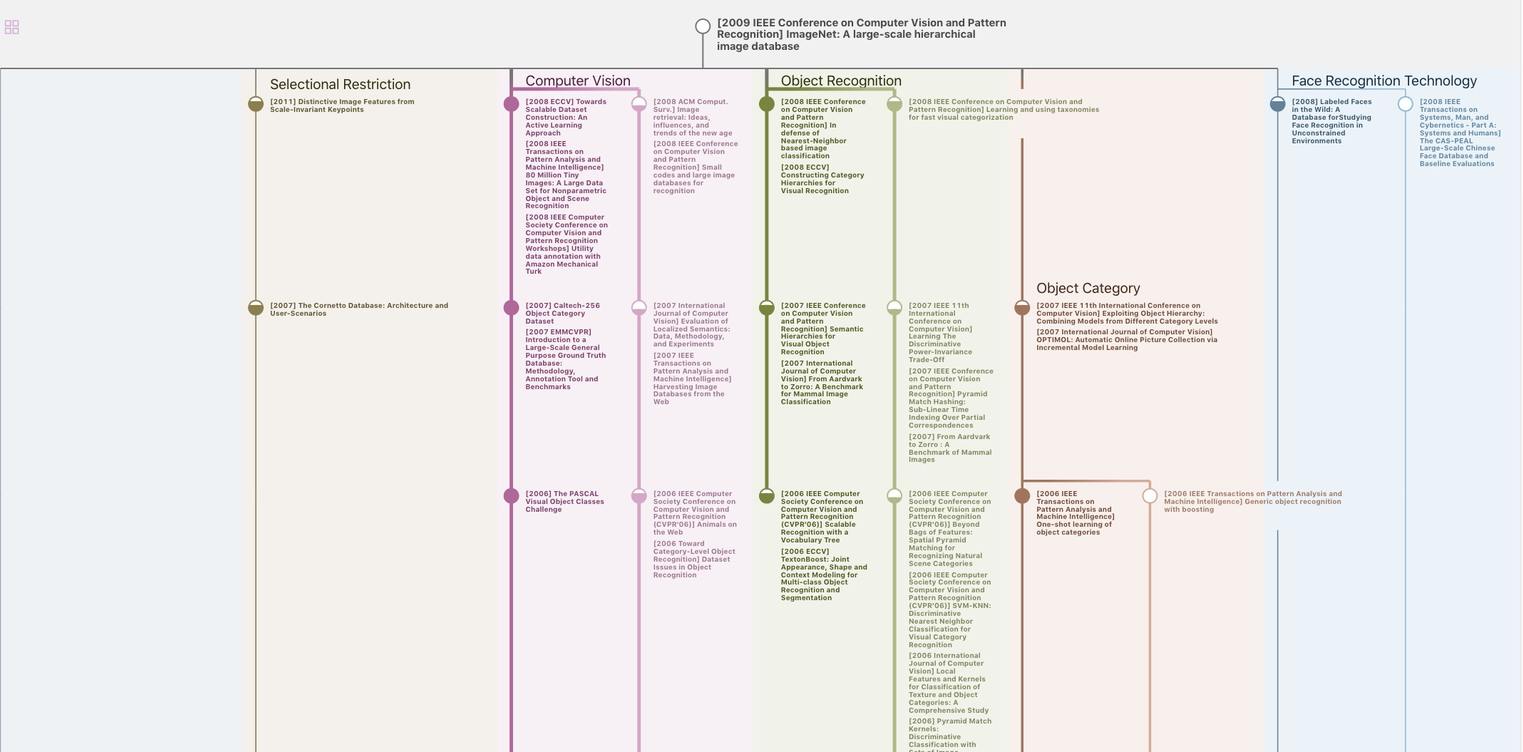
生成溯源树,研究论文发展脉络
Chat Paper
正在生成论文摘要