Detection of Image Level Forgery with Various Constraints Using DFDC Full and Sample Datasets.
Sensors(2022)
摘要
The emergence of advanced machine learning or deep learning techniques such as autoencoders and generative adversarial networks, can generate images known as deepfakes, which astonishingly resemble the realistic images. These deepfake images are hard to distinguish from the real images and are being used unethically against famous personalities such as politicians, celebrities, and social workers. Hence, we propose a method to detect these deepfake images using a light weighted convolutional neural network (CNN). Our research is conducted with Deep Fake Detection Challenge (DFDC) full and sample datasets, where we compare the performance of our proposed model with various state-of-the-art pretrained models such as VGG-19, Xception and Inception-ResNet-v2. Furthermore, we perform the experiments with various resolutions maintaining 1:1 and 9:16 aspect ratios, which have not been explored for DFDC datasets by any other groups to date. Thus, the proposed model can flexibly accommodate various resolutions and aspect ratios, without being constrained to a specific resolution or aspect ratio for any type of image classification problem. While most of the reported research is limited to sample or preview DFDC datasets only, we have also attempted the testing on full DFDC datasets and presented the results. Contemplating the fact that the detailed results and resource analysis for various scenarios are provided in this research, the proposed deepfake detection method is anticipated to pave new avenues for deepfake detection research, that engages with DFDC datasets.
更多查看译文
关键词
VGG-19,Xception,full DFDC,image-detection,inception-ResNet-v2,light weighted CNN,non-square aspect ratios,sample DFDC,variable resolutions
AI 理解论文
溯源树
样例
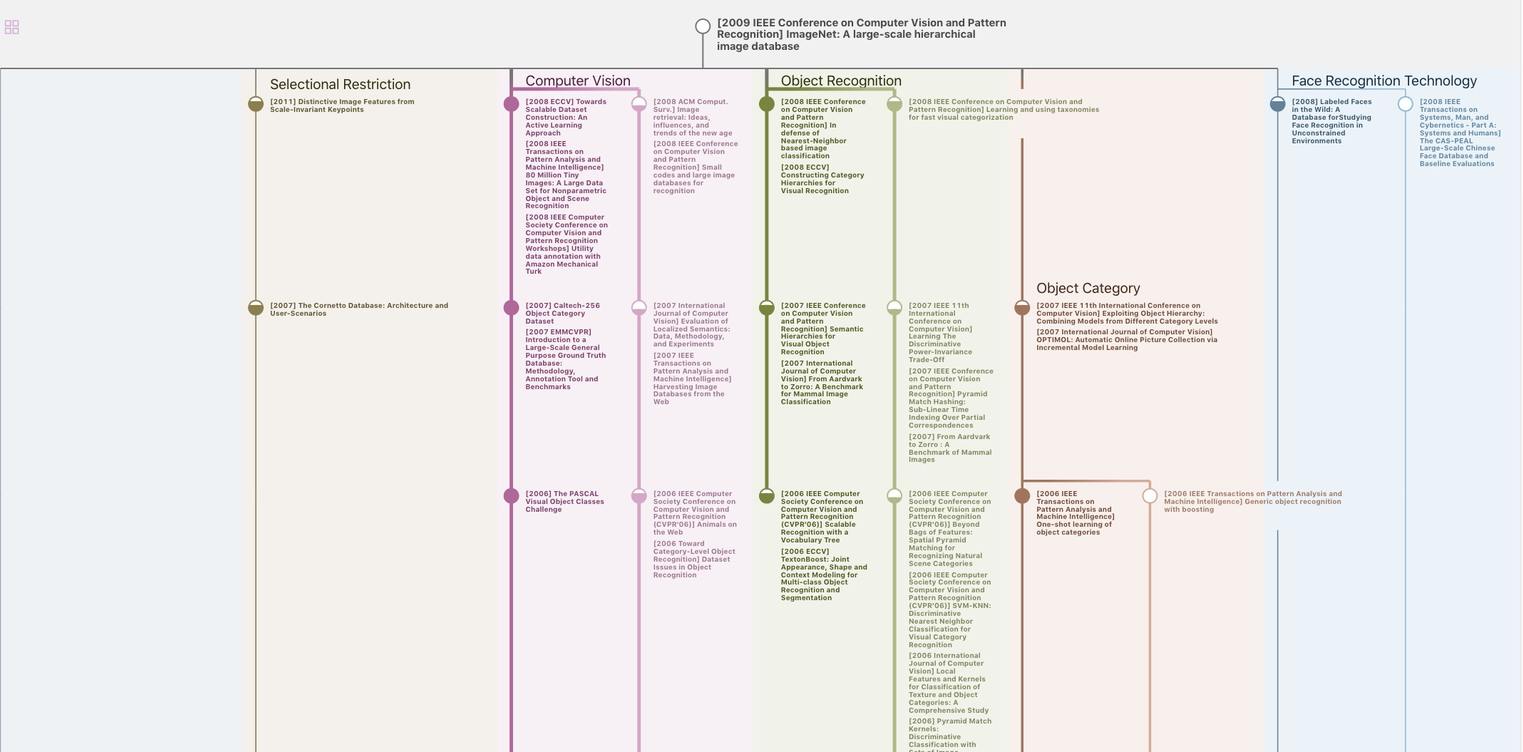
生成溯源树,研究论文发展脉络
Chat Paper
正在生成论文摘要