A fast implementation of coalitional model predictive controllers based on machine learning: Application to solar power plants
Engineering Applications of Artificial Intelligence(2023)
摘要
This article proposes a real-time implementation of distributed model predictive controllers to maximize the thermal energy generated by parabolic trough collector fields. For this control strategy, we consider that each loop of the solar collector field is individually managed by a controller, which can form coalition with other controllers to attain its local goals while contributing to the overall objective. The formation of coalitions is based on a market-based mechanism in which the heat transfer fluid is traded. To relieve the computational burden online, we propose a learning-based approach that approximates optimization problems so that the controller can be applied in real time. Finally, simulations in a 100 -loop solar collector field are used to assess the coalitional strategy based on neural networks in comparison with the coalitional model predictive control. The results show that the coalitional strategy based on neural networks provides a reduction in computing time of up to 99.74% and a minimal reduction in performance compared to the coalitional model predictive controller used as the baseline.
更多查看译文
关键词
Neural networks,Artificial intelligence,Non-linear model predictive control,Coalitional control,Multi-agent systems,Solar thermal applications
AI 理解论文
溯源树
样例
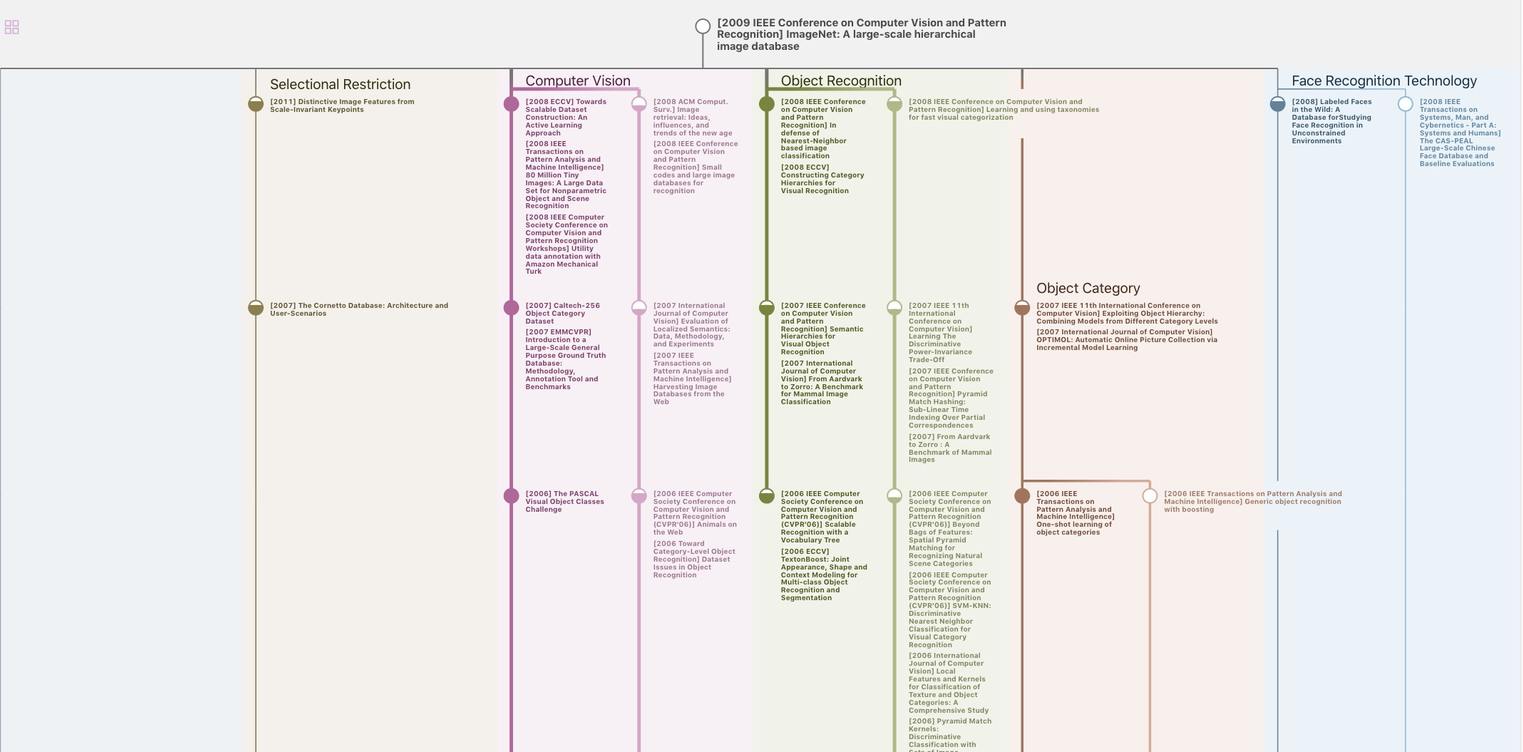
生成溯源树,研究论文发展脉络
Chat Paper
正在生成论文摘要