Acela: Predictable Datacenter-level Maintenance Job Scheduling
arxiv(2022)
摘要
Datacenter operators ensure fair and regular server maintenance by using automated processes to schedule maintenance jobs to complete within a strict time budget. Automating this scheduling problem is challenging because maintenance job duration varies based on both job type and hardware. While it is tempting to use prior machine learning techniques for predicting job duration, we find that the structure of the maintenance job scheduling problem creates a unique challenge. In particular, we show that prior machine learning methods that produce the lowest error predictions do not produce the best scheduling outcomes due to asymmetric costs. Specifically, underpredicting maintenance job duration has results in more servers being taken offline and longer server downtime than overpredicting maintenance job duration. The system cost of underprediction is much larger than that of overprediction. We present Acela, a machine learning system for predicting maintenance job duration, which uses quantile regression to bias duration predictions toward overprediction. We integrate Acela into a maintenance job scheduler and evaluate it on datasets from large-scale, production datacenters. Compared to machine learning based predictors from prior work, Acela reduces the number of servers that are taken offline by 1.87-4.28X, and reduces the server offline time by 1.40-2.80X.
更多查看译文
关键词
scheduling,maintenance,datacenter-level
AI 理解论文
溯源树
样例
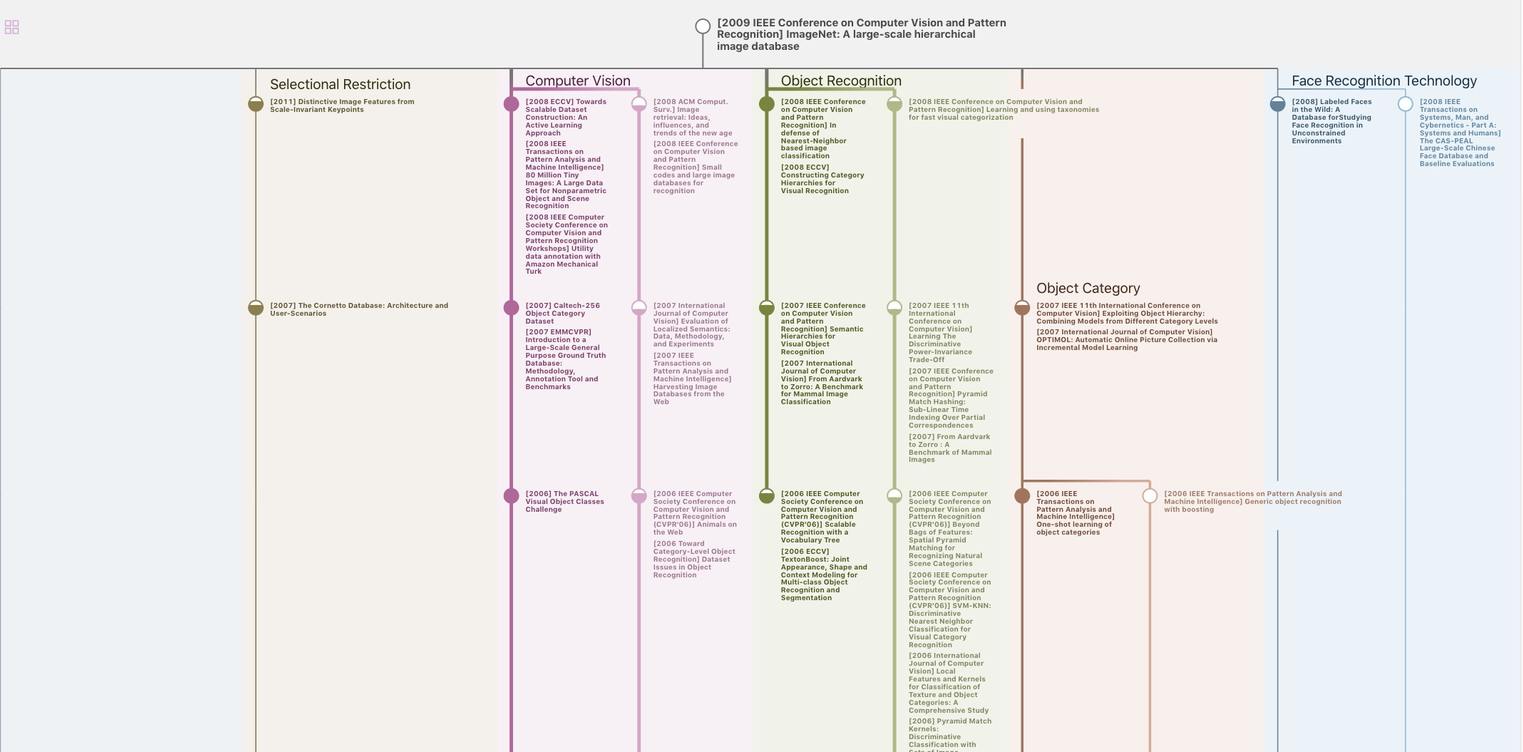
生成溯源树,研究论文发展脉络
Chat Paper
正在生成论文摘要