Collaborating Heterogeneous Natural Language Processing Tasks via Federated Learning
CoRR(2022)
摘要
The increasing privacy concerns on personal private text data promote the development of federated learning (FL) in recent years. However, the existing studies on applying FL in NLP are not suitable to coordinate participants with heterogeneous or private learning objectives. In this study, we further broaden the application scope of FL in NLP by proposing an Assign-Then-Contrast (denoted as ATC) framework, which enables clients with heterogeneous NLP tasks to construct an FL course and learn useful knowledge from each other. Specifically, the clients are suggested to first perform local training with the unified tasks assigned by the server rather than using their own learning objectives, which is called the Assign training stage. After that, in the Contrast training stage, clients train with different local learning objectives and exchange knowledge with other clients who contribute consistent and useful model updates. We conduct extensive experiments on six widely-used datasets covering both Natural Language Understanding (NLU) and Natural Language Generation (NLG) tasks, and the proposed ATC framework achieves significant improvements compared with various baseline methods. The source code is available at \url{https://github.com/alibaba/FederatedScope/tree/master/federatedscope/nlp/hetero_tasks}.
更多查看译文
关键词
collaborating,natural language,learning,processing
AI 理解论文
溯源树
样例
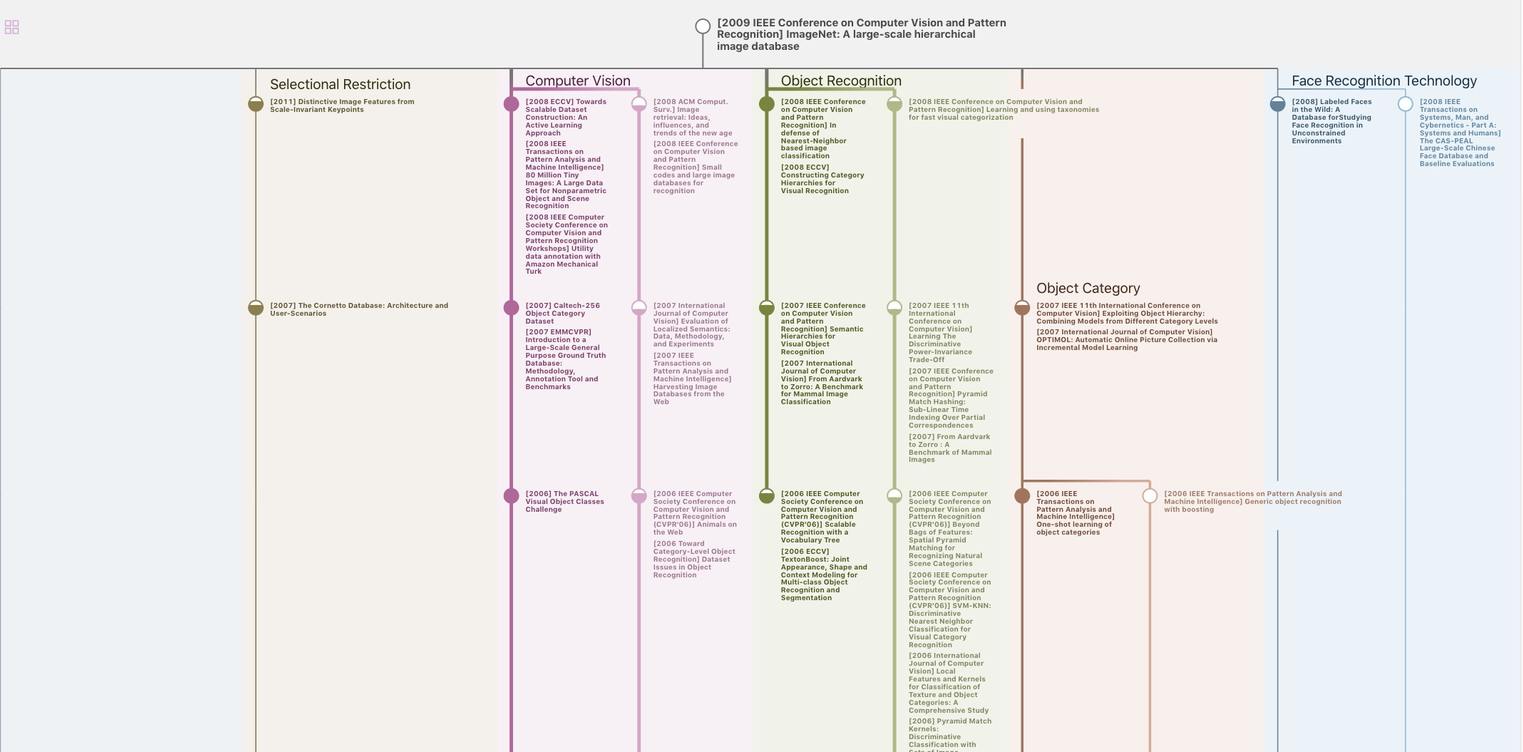
生成溯源树,研究论文发展脉络
Chat Paper
正在生成论文摘要