Lower Bounds on the Rate of Convergence for Accept-Reject-based Markov Chains in Wasserstein and Total Variation Distances
arXiv (Cornell University)(2022)
摘要
To avoid poor empirical performance in Metropolis-Hastings and other accept-reject-based algorithms practitioners often tune them by trial and error. Lower bounds on the convergence rate are developed in both total variation and Wasserstein distances in order to identify how the simulations will fail so these settings can be avoided, providing guidance on tuning. Particular attention is paid to using the lower bounds to study the convergence complexity of accept-reject-based Markov chains and to constrain the rate of convergence for geometrically ergodic Markov chains. The theory is applied in several settings. For example, if the target density concentrates with a parameter n (e.g. posterior concentration, Laplace approximations), it is demonstrated that the convergence rate of a Metropolis-Hastings chain can be arbitrarily slow if the tuning parameters do not depend carefully on n. This is demonstrated with Bayesian logistic regression with Zellner's g-prior when the dimension and sample increase together and flat prior Bayesian logistic regression as n tends to infinity.
更多查看译文
关键词
Approximation Algorithms,Inverse Problems,Approximate Bayesian Computation
AI 理解论文
溯源树
样例
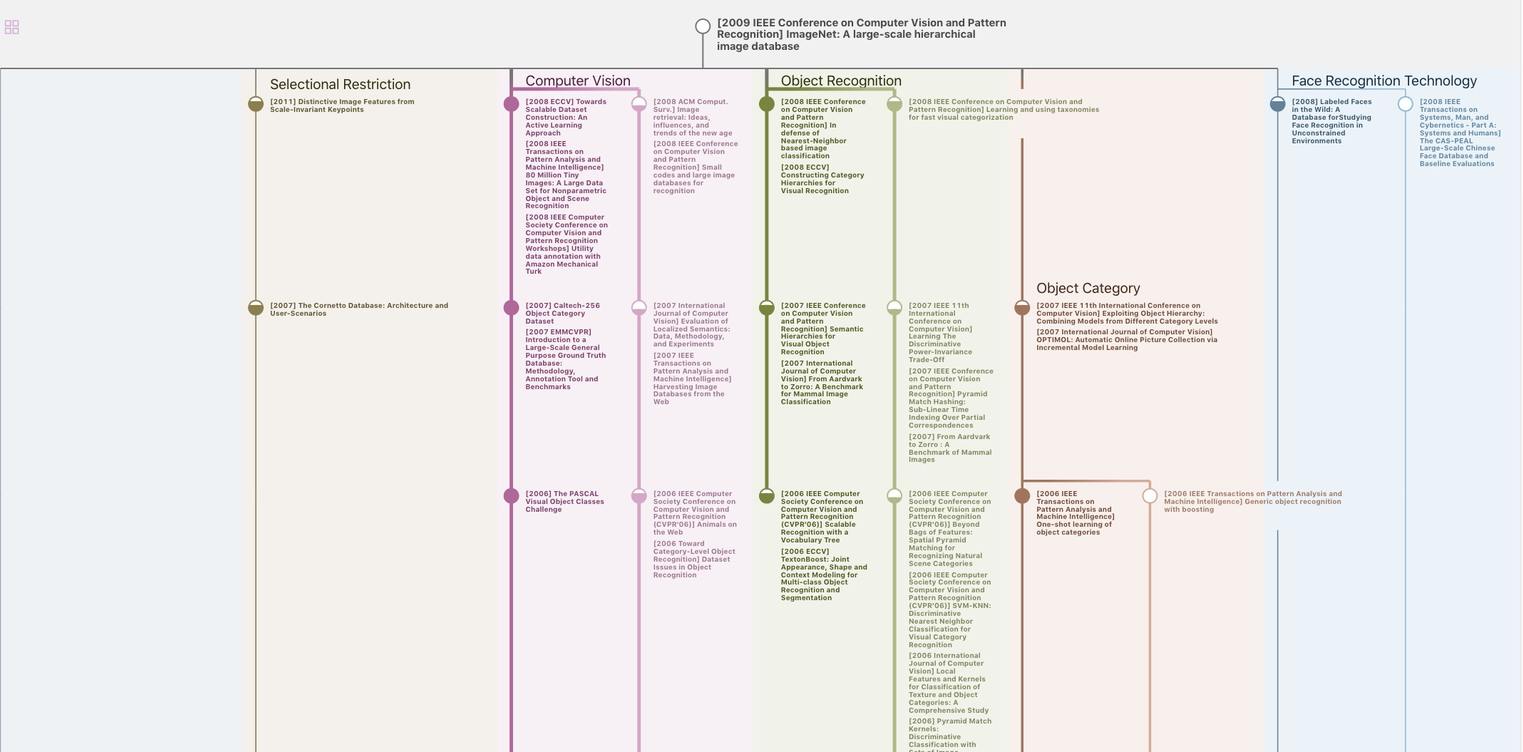
生成溯源树,研究论文发展脉络
Chat Paper
正在生成论文摘要